Real-World Learning Control for Autonomous Exploration of a Biomimetic Robotic Shark
IEEE Transactions on Industrial Electronics(2023)
摘要
With the development of learning-based autonomous underwater exploration method of robotic fish, how to improve data quality and sampling efficiency, so as to achieve better control performance, becomes a challenging research subject. To address this issue, in this article, a feasible real-world deep reinforcement learning framework for autonomous underwater exploration of robotic fish is proposed, which ably avoids model discrepancy generated in virtual training. The designed framework consists of three phases: 1) teaching initialization; 2) regular update of reinforcement learning; and 3) phased consolidation training. Especially, reasonable teaching initialization improves the data sampling efficiency and stabilizes the real-world early training. The consolidation training ensures the reproducibility of good controllers by interim imitation learning in the middle training phase. Extensive underwater experiments on a novel self-developed biomimetic robotic shark show that the proposed real-world learning method significantly improves the safety and efficiency of autonomous exploration based on local sensor information, providing a promising solution for exploring in uncharted wild waters.
更多查看译文
关键词
Autonomous exploration,deep reinforcement learning (DRL),imitation learning (IL),robotic fish
AI 理解论文
溯源树
样例
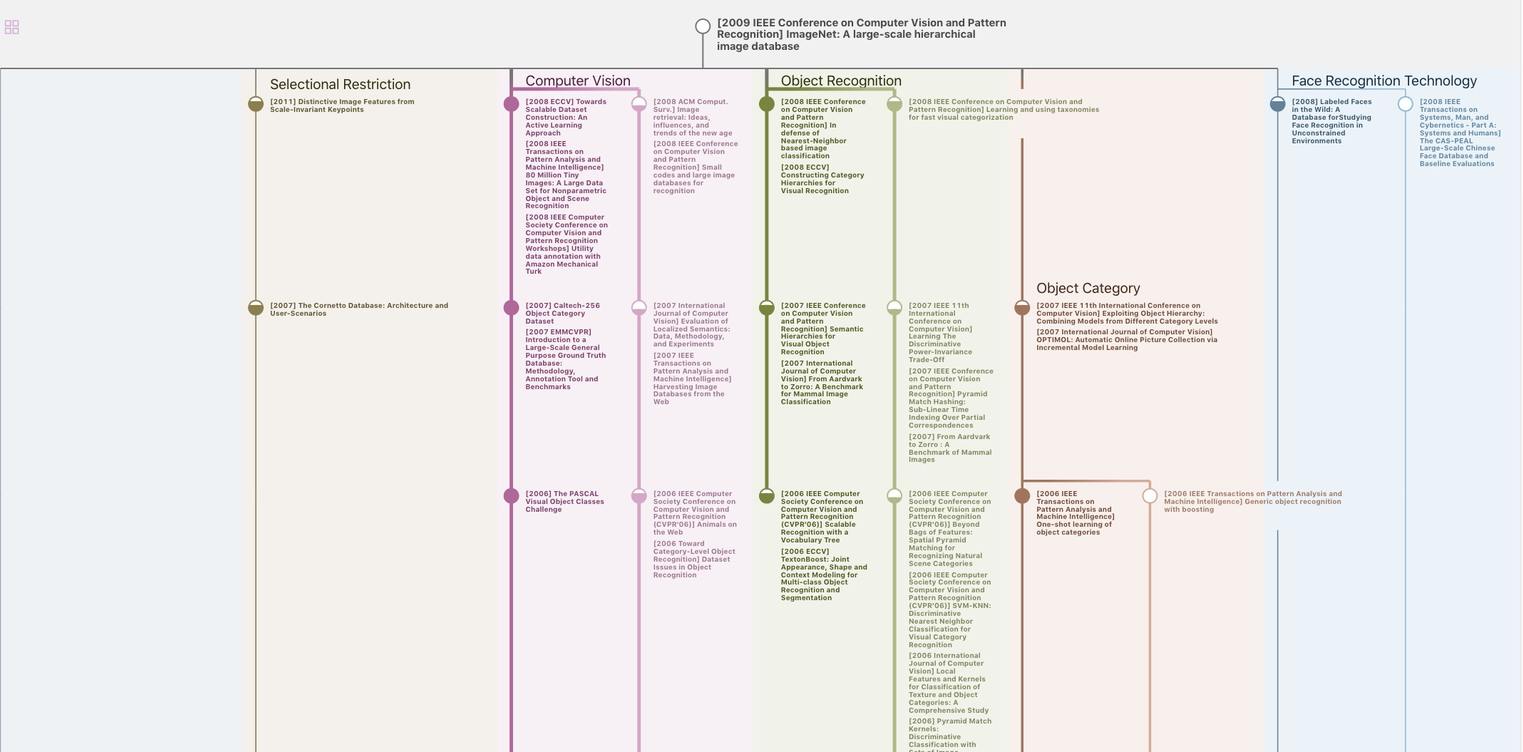
生成溯源树,研究论文发展脉络
Chat Paper
正在生成论文摘要