Learned Vertex Descent: A New Direction for 3D Human Model Fitting.
European Conference on Computer Vision(2022)
摘要
We propose a novel optimization-based paradigm for 3D human model fitting on images and scans. In contrast to existing approaches that directly regress the parameters of a low-dimensional statistical body model (e.g.SMPL) from input images, we train an ensemble of per vertex neural fields network. The network predicts, in a distributed manner, the vertex descent direction towards the ground truth, based on neural features extracted at the current vertex projection. At inference, we employ this network, dubbed LVD, within a gradient-descent optimization pipeline until its convergence, which typically occurs in a fraction of a second even when initializing all vertices into a single point. An exhaustive evaluation demonstrates that our approach is able to capture the underlying body of clothed people with very different body shapes, achieving a significant improvement compared to state-of-the-art. LVD is also applicable to 3D model fitting of humans and hands, for which we show a significant improvement to the SOTA with a much simpler and faster method. Code is released at https://www.iri.upc.edu/people/ecorona/lvd/
更多查看译文
AI 理解论文
溯源树
样例
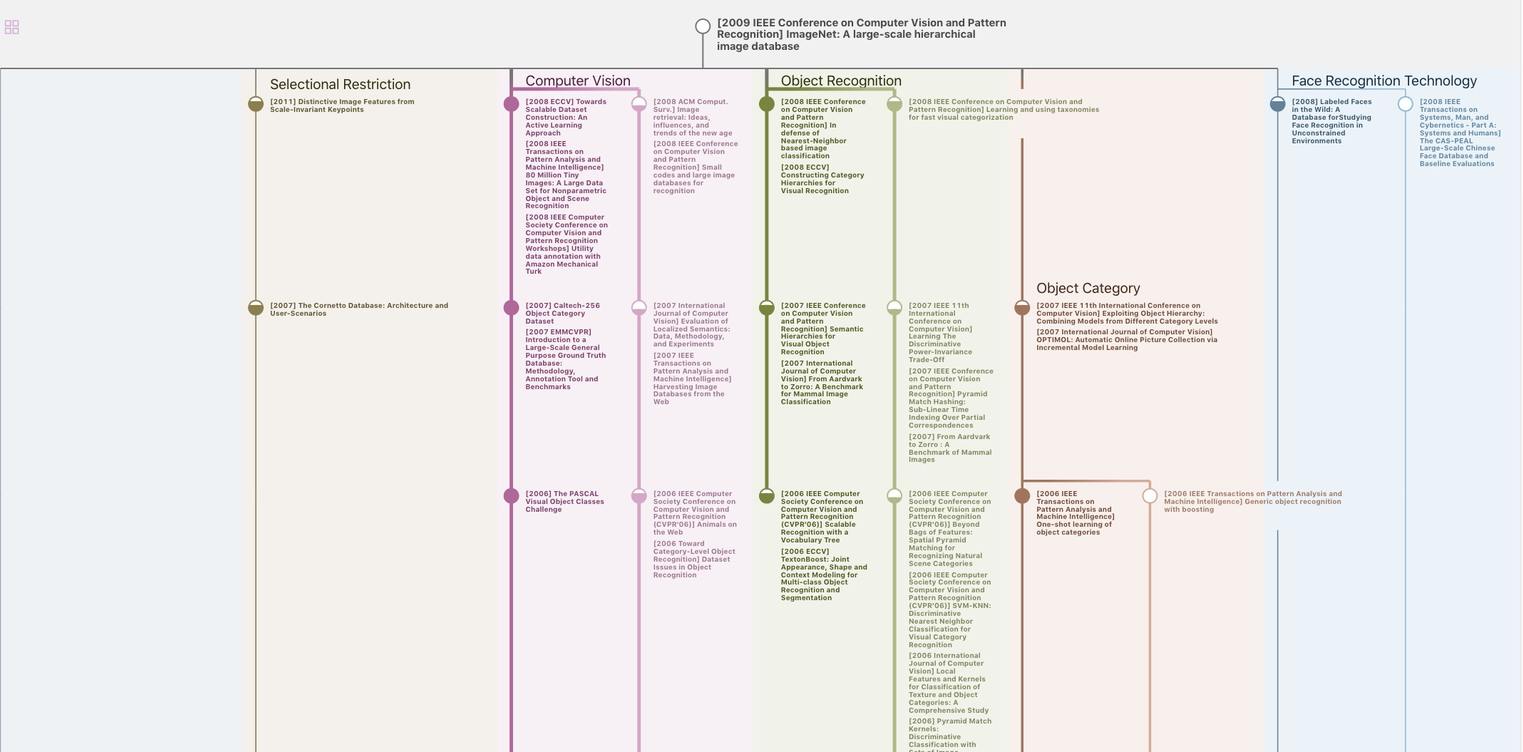
生成溯源树,研究论文发展脉络
Chat Paper
正在生成论文摘要