VI-IKD: High-Speed Accurate Off-Road Navigation using Learned Visual-Inertial Inverse Kinodynamics.
IEEE/RJS International Conference on Intelligent RObots and Systems (IROS)(2022)
摘要
One of the key challenges in high speed off road navigation on ground vehicles is that the kinodynamics of the vehicle terrain interaction can differ dramatically depending on the terrain. Previous approaches to addressing this challenge have considered learning an inverse kinodynamics (IKD) model, conditioned on inertial information of the vehicle to sense the kinodynamic interactions. In this paper, we hypothesize that to enable accurate high-speed off-road navigation using a learned IKD model, in addition to inertial information from the past, one must also anticipate the kinodynamic interactions of the vehicle with the terrain in the future. To this end, we introduce Visual-Inertial Inverse Kinodynamics (VI-IKD), a novel learning based IKD model that is conditioned on visual information from a terrain patch ahead of the robot in addition to past inertial information, enabling it to anticipate kinodynamic interactions in the future. We validate the effectiveness of VI-IKD in accurate high-speed off-road navigation experimentally on a scale 1/5 UT-AlphaTruck off-road autonomous vehicle in both indoor and outdoor environments and show that compared to other state-of-the-art approaches, VI-IKD enables more accurate and robust off-road navigation on a variety of different terrains at speeds of up to 3.5 m/s.
更多查看译文
关键词
different terrains,ground vehicles,high-speed accurate off-road navigation,inertial information,inverse kinodynamics model,kinodynamic interactions,learned IKD model,learning based IKD model,terrain patch,UT-AlphaTruck off-road autonomous vehicle,vehicle-terrain interaction,velocity 3.5 m/s,VI-IKD,visual information,visual-inertial inverse kinodynamics
AI 理解论文
溯源树
样例
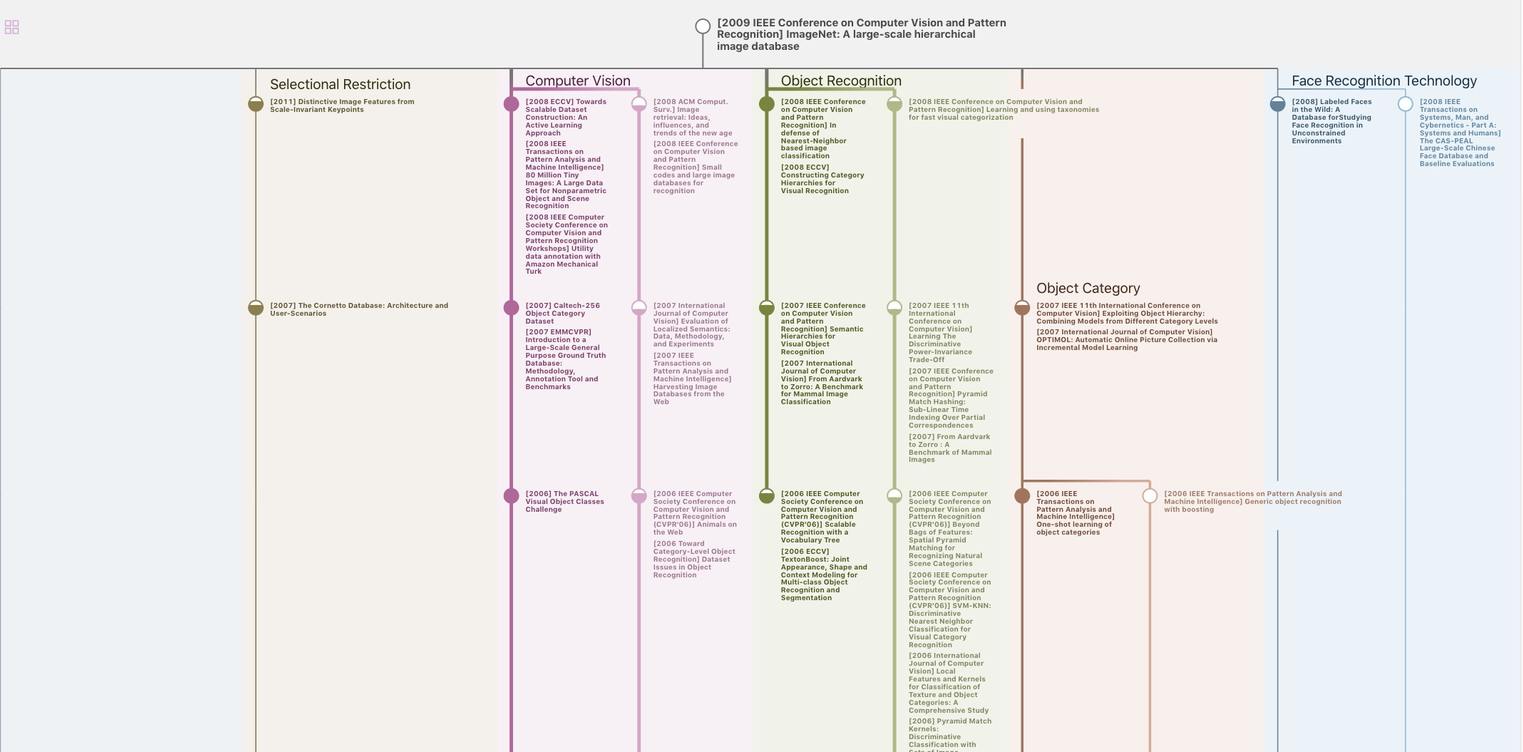
生成溯源树,研究论文发展脉络
Chat Paper
正在生成论文摘要