Speaker-turn aware diarization for speech-based cognitive assessments
FRONTIERS IN NEUROSCIENCE(2024)
摘要
Introduction Speaker diarization is an essential preprocessing step for diagnosing cognitive impairments from speech-based Montreal cognitive assessments (MoCA).Methods This paper proposes three enhancements to the conventional speaker diarization methods for such assessments. The enhancements tackle the challenges of diarizing MoCA recordings on two fronts. First, multi-scale channel interdependence speaker embedding is used as the front-end speaker representation for overcoming the acoustic mismatch caused by far-field microphones. Specifically, a squeeze-and-excitation (SE) unit and channel-dependent attention are added to Res2Net blocks for multi-scale feature aggregation. Second, a sequence comparison approach with a holistic view of the whole conversation is applied to measure the similarity of short speech segments in the conversation, which results in a speaker-turn aware scoring matrix for the subsequent clustering step. Third, to further enhance the diarization performance, we propose incorporating a pairwise similarity measure so that the speaker-turn aware scoring matrix contains both local and global information across the segments.Results Evaluations on an interactive MoCA dataset show that the proposed enhancements lead to a diarization system that outperforms the conventional x-vector/PLDA systems under language-, age-, and microphone-mismatch scenarios.Discussion The results also show that the proposed enhancements can help hypothesize the speaker-turn timestamps, making the diarization method amendable to datasets without timestamp information.
更多查看译文
关键词
speaker diarization,speaker embedding,comprehensive scoring,speaker-turn timestamps,MOCA,dementia detection
AI 理解论文
溯源树
样例
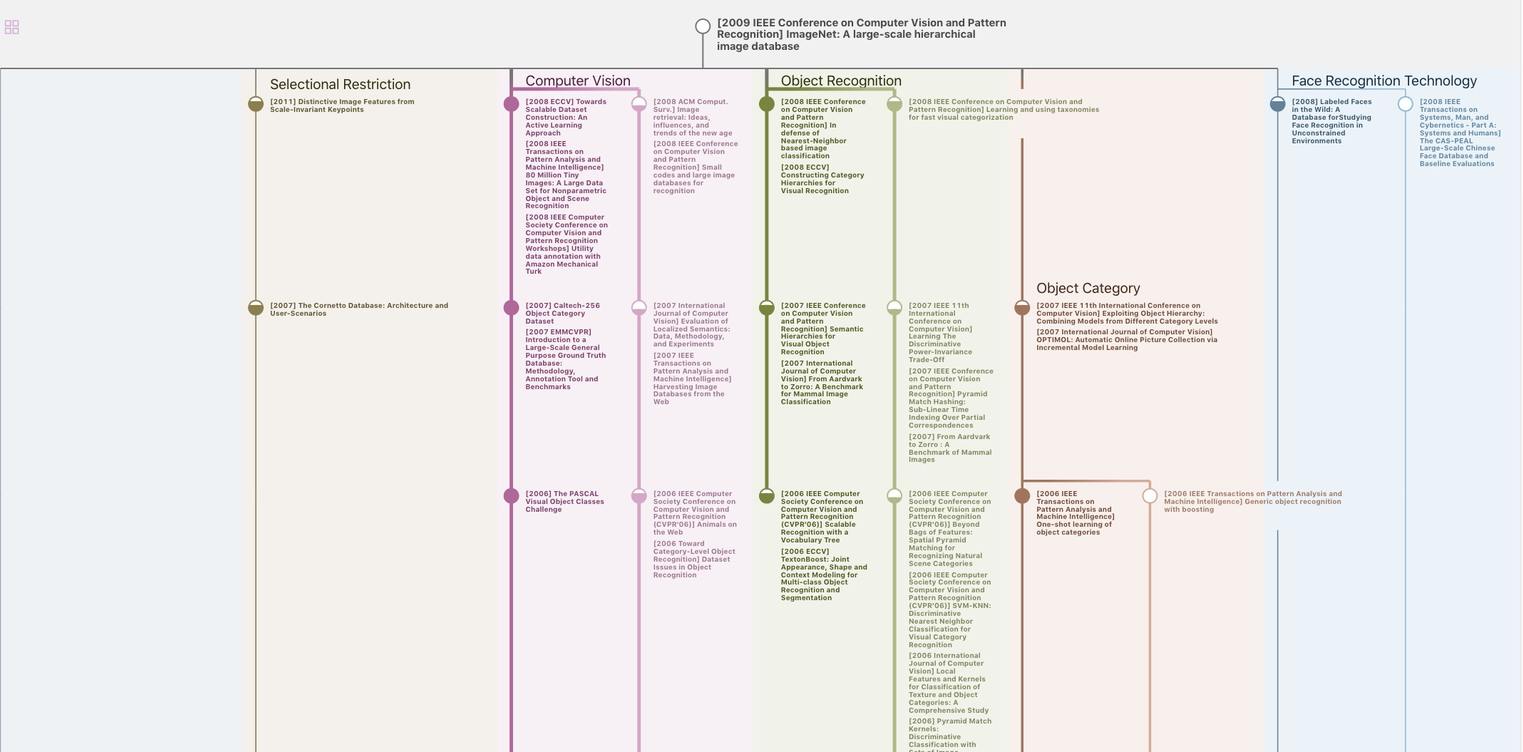
生成溯源树,研究论文发展脉络
Chat Paper
正在生成论文摘要