Robust and Provable Guarantees for Sparse Random Embeddings
ADVANCES IN KNOWLEDGE DISCOVERY AND DATA MINING, PAKDD 2022, PT II(2022)
摘要
In this work, we improve upon the guarantees for sparse random embeddings, as they were recently provided and analyzed by Freksen at al. (NIPS'18) and Jagadeesan (NIPS'19). Specifically, we show that (a) our bounds are explicit as opposed to the asymptotic guarantees provided previously, and (b) our bounds are guaranteed to be sharper by practically significant constants across a wide range of parameters, including the dimensionality, sparsity and dispersion of the data. Moreover, we empirically demonstrate that our bounds significantly outperform prior works on a wide range of real-world datasets, such as collections of images, text documents represented as bags-of-words, and text sequences vectorized by neural embeddings. Behind our numerical improvements are techniques of broader interest, which improve upon key steps of previous analyses in terms of (c) tighter estimates for certain types of quadratic chaos, (d) establishing extreme properties of sparse linear forms, and (e) improvements on bounds for the estimation of sums of independent random variables.
更多查看译文
关键词
Sparse random embeddings, Johnson-Lindenstrauss lemma
AI 理解论文
溯源树
样例
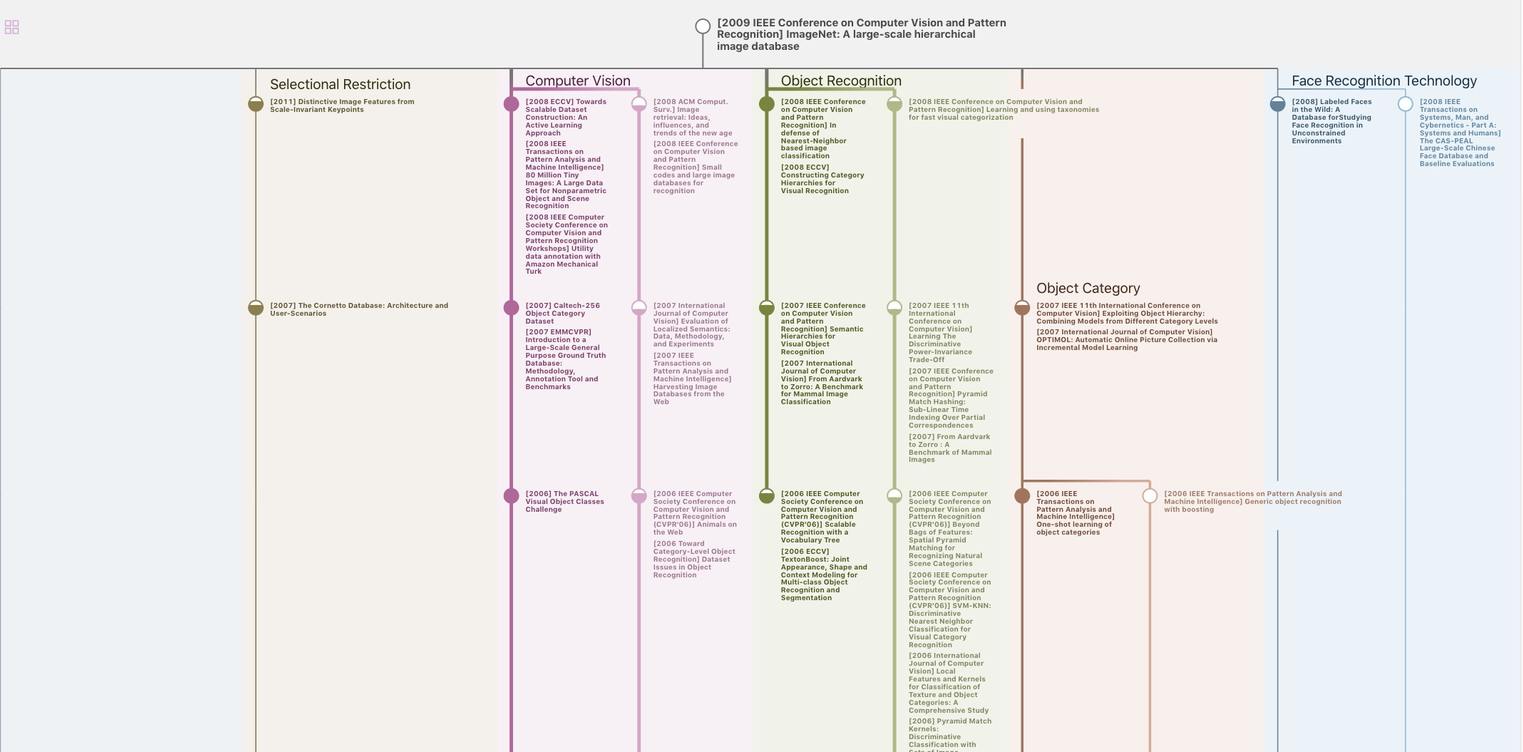
生成溯源树,研究论文发展脉络
Chat Paper
正在生成论文摘要