Learning to Rank Articles for Molecular Queries.
AAAI Conference on Artificial Intelligence(2022)
摘要
The cost of developing new drugs is estimated at billions of dollars per year. Identification of new molecules for drugs involves scanning existing bio-medical literature for relevant information. As the potential drug molecule is novel, retrieval of relevant information using a simple direct search is less likely to be productive. Identifying relevant papers is therefore a more complex and challenging task, which requires searching for information on molecules with similar characteristics to the novel drug. In this paper, we present the novel task of ranking documents based on novel molecule queries. Given a chemical molecular structure, we wish to rank medical papers that will contribute to a researcher's understanding of the novel molecule drug potential.We present a set of ranking algorithms and molecular embeddings to address the task. An extensive evaluation of the algorithms is performed over the molecular embeddings, studying their performance on a benchmark retrieval corpus, which we share with the community.Additionally, we introduce a heterogeneous edge-labeled graph embedding approach to address the molecule ranking task. Our evaluation shows that the proposed embedding model can significantly improve molecule ranking methods. The system is currently deployed in a targeted drug delivery and personalized medicine research laboratory.
更多查看译文
关键词
Health And Medicine,Molecule Ranking,Molecule Discovery
AI 理解论文
溯源树
样例
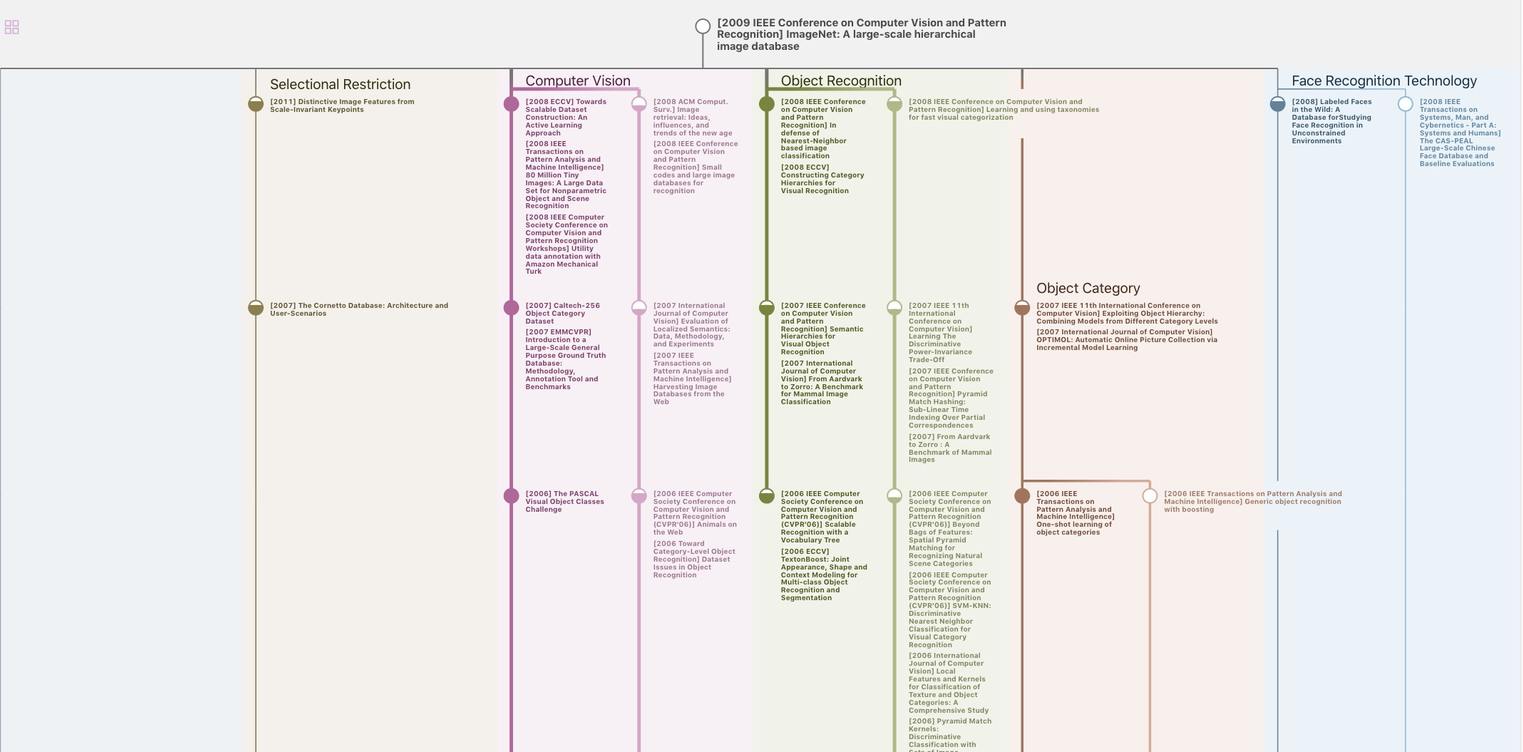
生成溯源树,研究论文发展脉络
Chat Paper
正在生成论文摘要