Learning Assisted Identification of Scenarios Where Network Optimization Algorithms Under-Perform
2021 IEEE GLOBAL COMMUNICATIONS CONFERENCE (GLOBECOM)(2021)
摘要
We present a generative adversarial method that uses deep learning to identify network load traffic conditions in which network optimization algorithms under-perform other known algorithms: the Deep Convolutional Failure Generator (DCFG). The spatial distribution of network load presents challenges for network operators for tasks such as load balancing, in which a network optimizer attempts to maintain high quality communication while at the same time abiding capacity constraints. Testing a network optimizer for all possible load distributions is challenging if not impossible. We propose a novel method that searches for load situations where a target network optimization method underperforms baseline, which are key test cases that can be used for future refinement and performance optimization. By modeling a realistic network simulator's quality assessments with a deep network and, in parallel, optimizing a load generation network, our method efficiently searches the high dimensional space of load patterns and reliably finds cases in which a target network optimization method under-performs a baseline by a significant margin.
更多查看译文
关键词
target network optimization method,load generation network,generative adversarial method,deep learning network,network load traffic conditions,deep convolutional failure generator,load balancing,load distributions,DCFG
AI 理解论文
溯源树
样例
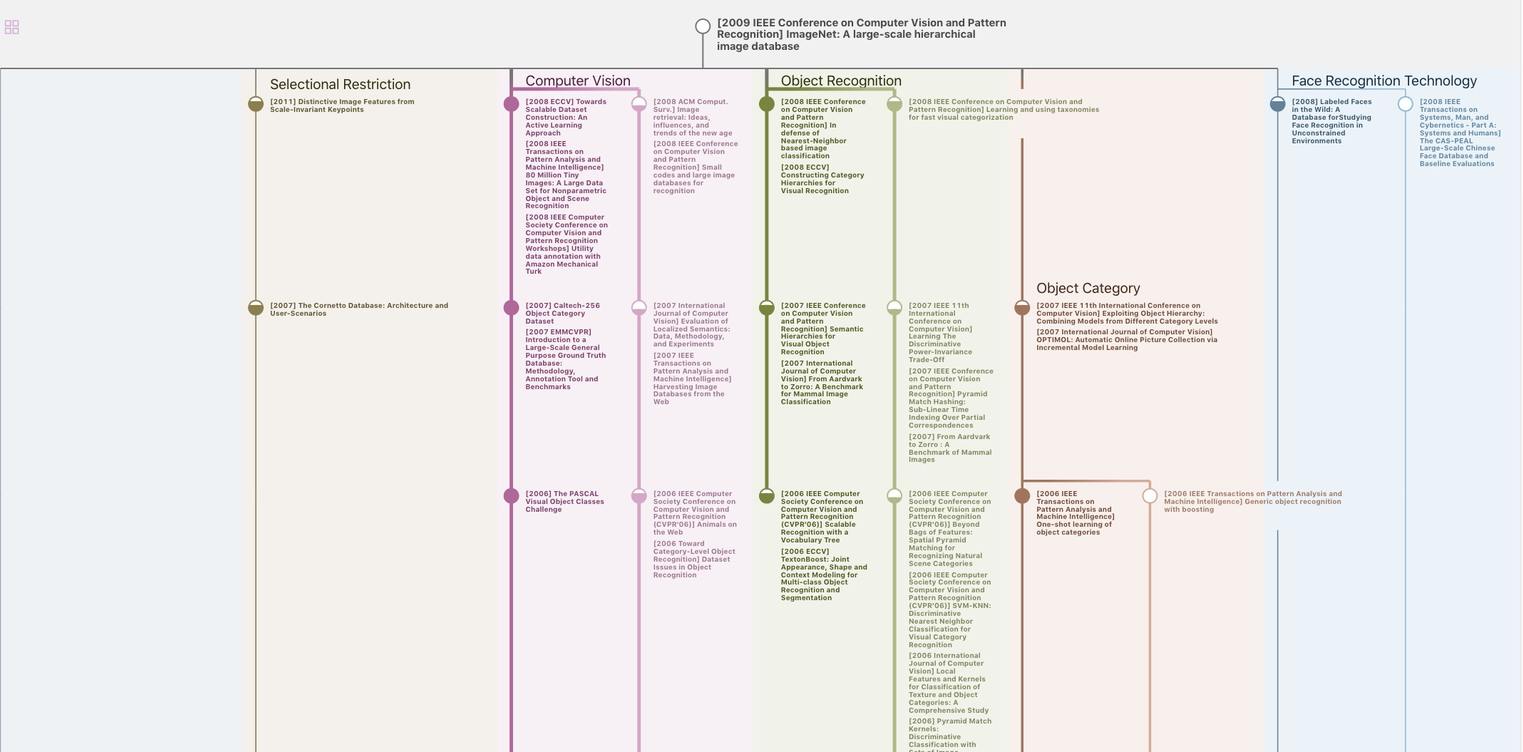
生成溯源树,研究论文发展脉络
Chat Paper
正在生成论文摘要