On the Robustness of Quality Measures for GANs.
European Conference on Computer Vision(2022)
摘要
This work evaluates the robustness of quality measures of generative models such as Inception Score (IS) and Fr\'echet Inception Distance (FID). Analogous to the vulnerability of deep models against a variety of adversarial attacks, we show that such metrics can also be manipulated by additive pixel perturbations. Our experiments indicate that one can generate a distribution of images with very high scores but low perceptual quality. Conversely, one can optimize for small imperceptible perturbations that, when added to real world images, deteriorate their scores. We further extend our evaluation to generative models themselves, including the state of the art network StyleGANv2. We show the vulnerability of both the generative model and the FID against additive perturbations in the latent space. Finally, we show that the FID can be robustified by simply replacing the standard Inception with a robust Inception. We validate the effectiveness of the robustified metric through extensive experiments, showing it is more robust against manipulation.
更多查看译文
关键词
Generative Adversarial Networks, Perceptual quality, Adversarial attacks, Network robustness
AI 理解论文
溯源树
样例
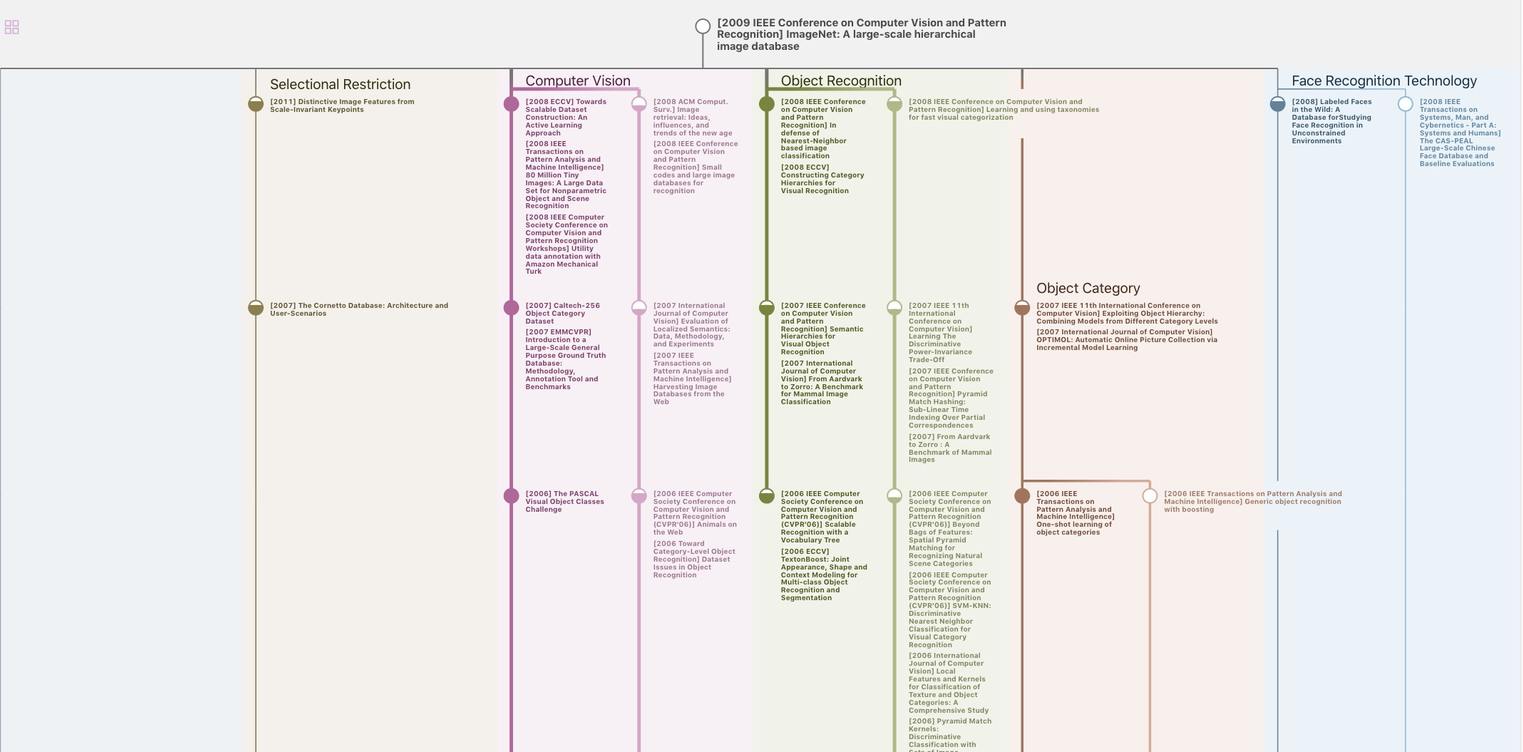
生成溯源树,研究论文发展脉络
Chat Paper
正在生成论文摘要