An Adaptive Imitation Learning Framework for Robotic Complex Contact-Rich Insertion Tasks
FRONTIERS IN ROBOTICS AND AI(2022)
摘要
Complex contact-rich insertion is a ubiquitous robotic manipulation skill and usually involves nonlinear and low-clearance insertion trajectories as well as varying force requirements. A hybrid trajectory and force learning framework can be utilized to generate high-quality trajectories by imitation learning and find suitable force control policies efficiently by reinforcement learning. However, with the mentioned approach, many human demonstrations are necessary to learn several tasks even when those tasks require topologically similar trajectories. Therefore, to reduce human repetitive teaching efforts for new tasks, we present an adaptive imitation framework for robot manipulation. The main contribution of this work is the development of a framework that introduces dynamic movement primitives into a hybrid trajectory and force learning framework to learn a specific class of complex contact-rich insertion tasks based on the trajectory profile of a single task instance belonging to the task class. Through experimental evaluations, we validate that the proposed framework is sample efficient, safer, and generalizes better at learning complex contact-rich insertion tasks on both simulation environments and on real hardware.
更多查看译文
关键词
compliance control, imitation learning, reinforcement learning, robotic assembly, robot autonomy
AI 理解论文
溯源树
样例
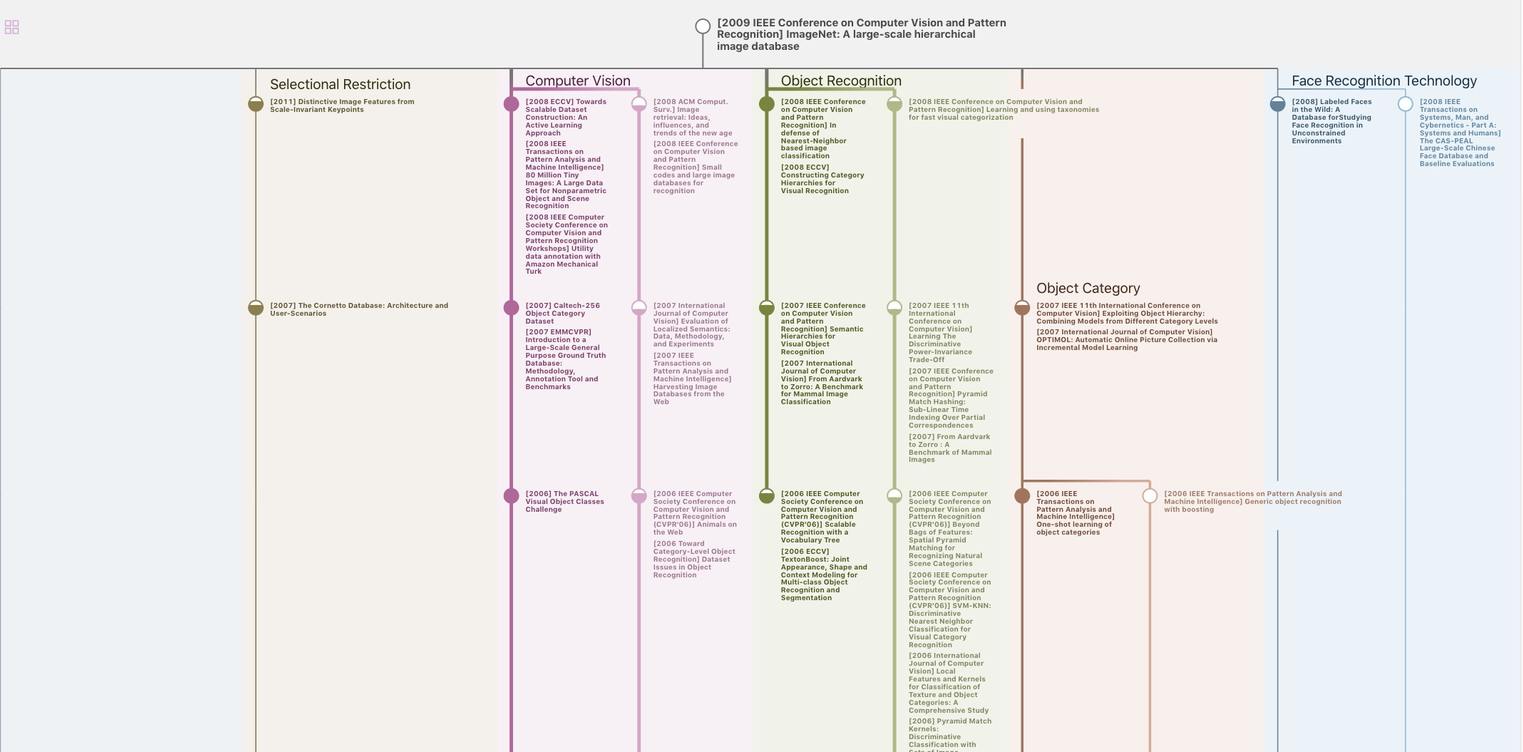
生成溯源树,研究论文发展脉络
Chat Paper
正在生成论文摘要