Competing risks analysis with missing cause-of-failure-penalized likelihood estimation of cause-specific Cox models
STATISTICAL METHODS IN MEDICAL RESEARCH(2022)
摘要
Competing risks models are attractive tools to analyze time-to-event data where several causes of an event are competing. However, a complexity may arise when, for instance, some subjects experience the event of interest but the causes are not known. Assuming that unknown causes of events are missing at random, we developed a novel constrained maximum penalized likelihood method for fitting semi-parametric cause-specific Cox regression models. Here, penalty functions were used to smooth the baseline hazards. An appealing feature of this approach is that all the relevant estimands in competing risks models are estimated including cause-specific hazard ratios, cause-specific baseline hazards, and cumulative incidence functions. Asymptotic results for these estimators were also developed, allowing for direct inferences. The proposed method was compared with some existing methods through a simulation study. A real data example was analyzed using the new method to evaluate the association of age at diagnosis with melanoma-death and non-melanoma-death in patients diagnosed with thin melanoma (tumour thickness <= 1.0 mm). An R function for our proposed method is currently available on GitHub and will be included in the R package "survivalMPL" at CRAN.
更多查看译文
关键词
Competing risks, missing cause of failure, cause-specific Cox model, penalized likelihood estimation, constrained optimization
AI 理解论文
溯源树
样例
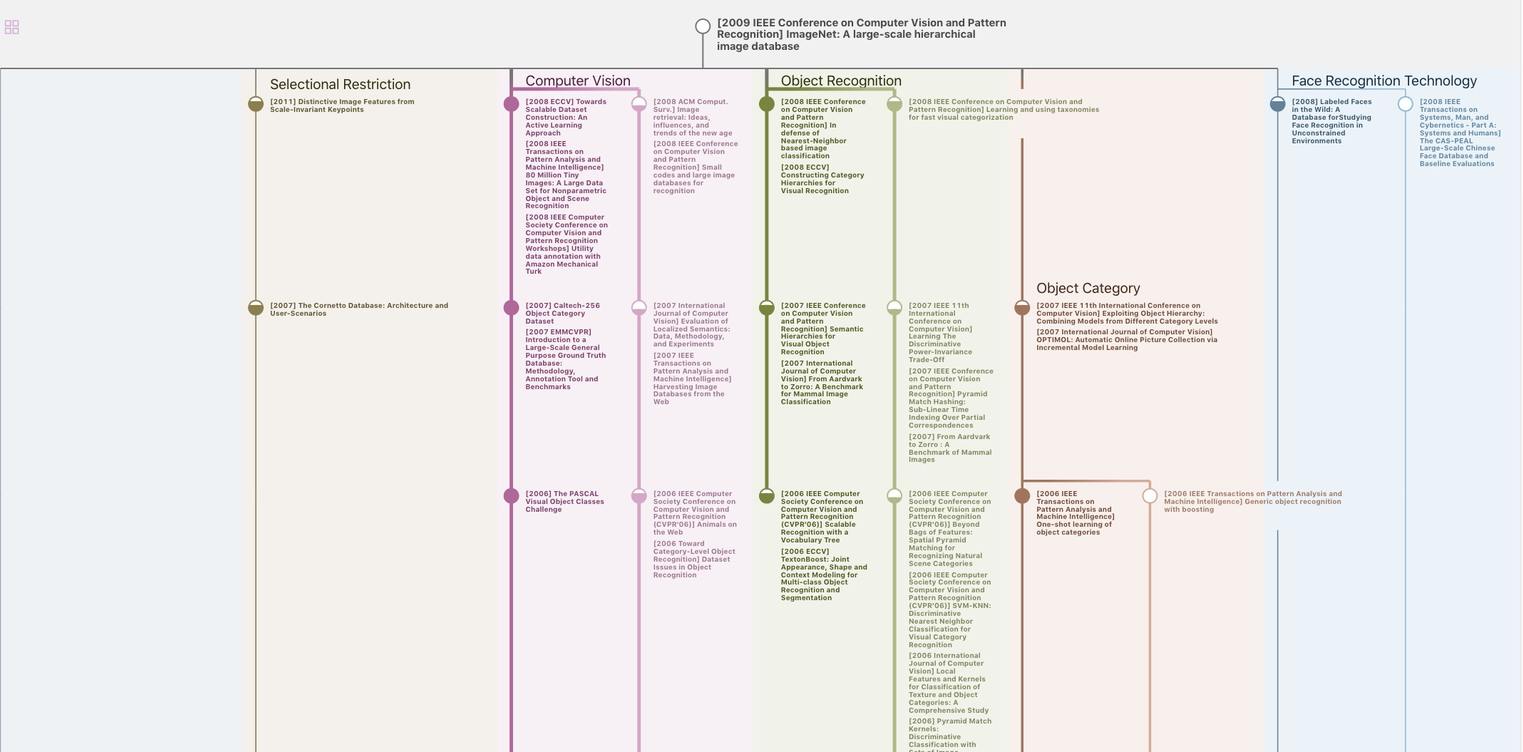
生成溯源树,研究论文发展脉络
Chat Paper
正在生成论文摘要