TextRGNN: Residual Graph Neural Networks for Text Classification
arxiv(2023)
摘要
Recently, text classification model based on graph neural network (GNN) has attracted more and more attention. Most of these models adopt a similar network paradigm, that is, using pre-training node embedding initialization and two-layer graph convolution. In this work, we propose TextRGNN, an improved GNN structure that introduces residual connection to deepen the convolution network depth. Our structure can obtain a wider node receptive field and effectively suppress the over-smoothing of node features. In addition, we integrate the probabilistic language model into the initialization of graph node embedding, so that the non-graph semantic information of can be better extracted. The experimental results show that our model is general and efficient. It can significantly improve the classification accuracy whether in corpus level or text level, and achieve SOTA performance on a wide range of text classification datasets.
更多查看译文
关键词
residual graph neural networks,classification
AI 理解论文
溯源树
样例
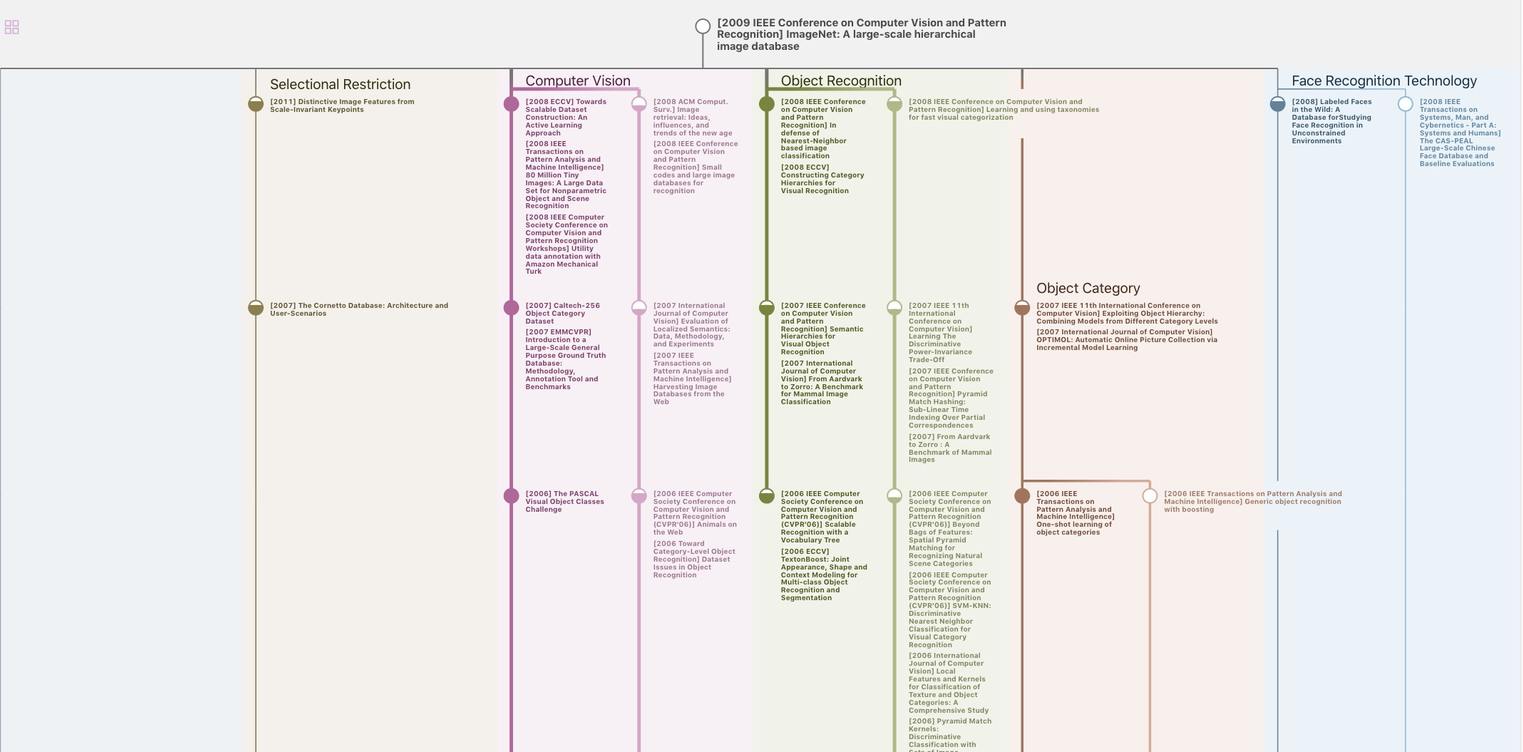
生成溯源树,研究论文发展脉络
Chat Paper
正在生成论文摘要