DWDN: Deep Wiener Deconvolution Network for Non-Blind Image Deblurring
IEEE Transactions on Pattern Analysis and Machine Intelligence(2022)
摘要
We present a simple and effective approach for non-blind image deblurring, combining classical techniques and deep learning. In contrast to existing methods that deblur the image directly in the standard image space, we propose to perform an explicit deconvolution process in a feature space by integrating a classical Wiener deconvolution framework with learned deep features. A multi-scale cascaded feature refinement module then predicts the deblurred image from the deconvolved deep features, progressively recovering detail and small-scale structures. The proposed model is trained in an end-to-end manner and evaluated on scenarios with simulated Gaussian noise, saturated pixels, or JPEG compression artifacts as well as real-world images. Moreover, we present detailed analyses of the benefit of the feature-based Wiener deconvolution and of the multi-scale cascaded feature refinement as well as the robustness of the proposed approach. Our extensive experimental results show that the proposed
deep Wiener deconvolution network
facilitates deblurred results with visibly fewer artifacts and quantitatively outperforms state-of-the-art non-blind image deblurring methods by a wide margin.
更多查看译文
关键词
Image deblurring,wiener deconvolution,feature-based deconvolution,multi-scale cascaded feature refinement,saturation and JPEG artifacts
AI 理解论文
溯源树
样例
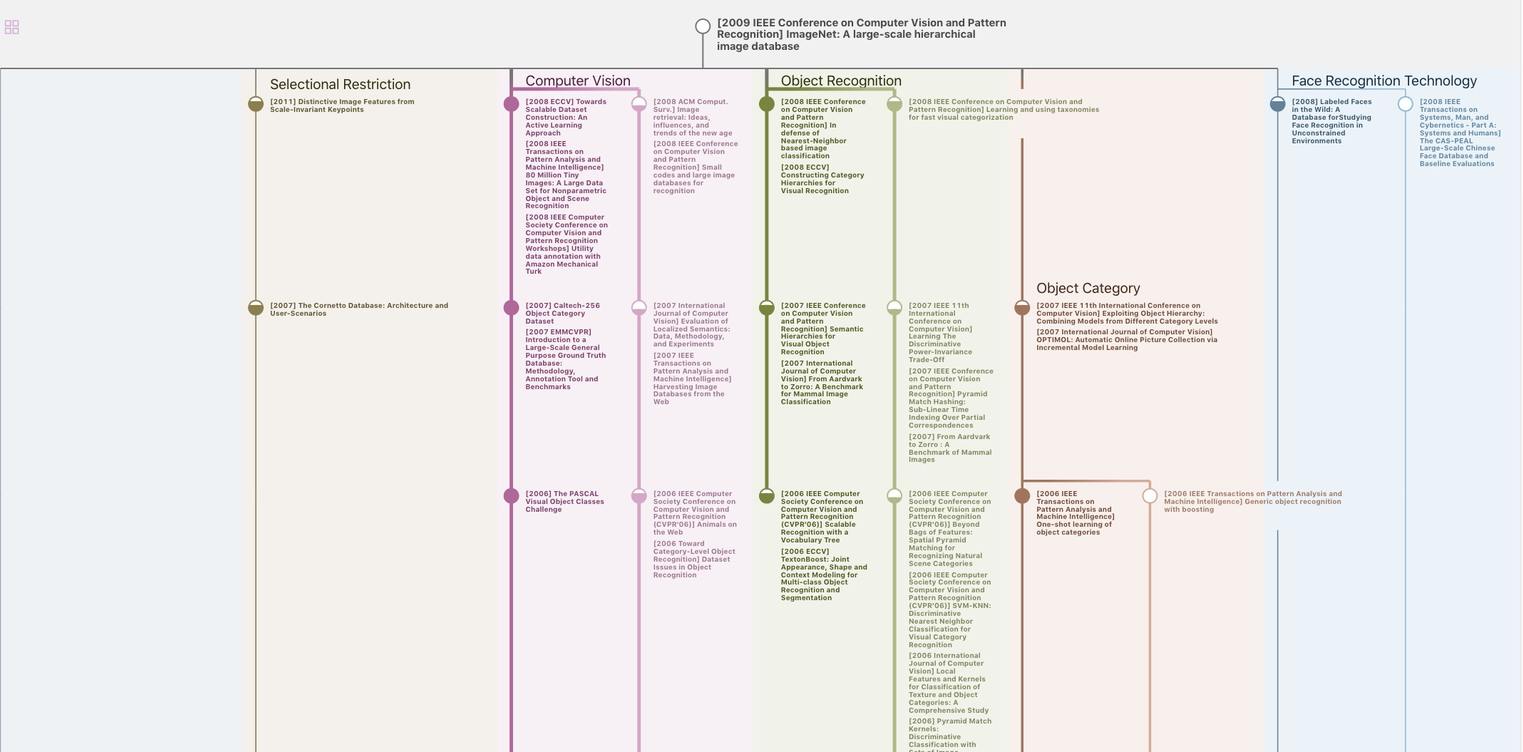
生成溯源树,研究论文发展脉络
Chat Paper
正在生成论文摘要