Best practices for addressing missing data through multiple imputation
INFANT AND CHILD DEVELOPMENT(2024)
摘要
A common challenge in developmental research is the amount of incomplete and missing data that occurs from respondents failing to complete tasks or questionnaires, as well as from disengaging from the study (i.e., attrition). This missingness can lead to biases in parameter estimates and, hence, in the interpretation of findings. These biases can be addressed through statistical techniques that adjust for missing data, such as multiple imputation. Although multiple imputation is highly effective, it has not been widely adopted by developmental scientists given barriers such as lack of training or misconceptions about imputation methods. Utilizing default methods within statistical software programs like listwise deletion is common but may introduce additional bias. This manuscript is intended to provide practical guidelines for developmental researchers to follow when examining their data for missingness, making decisions about how to handle that missingness and reporting the extent of missing data biases and specific multiple imputation procedures in publications.
更多查看译文
关键词
development,missing data,missingness mechanisms,multiple imputation,open scholarship
AI 理解论文
溯源树
样例
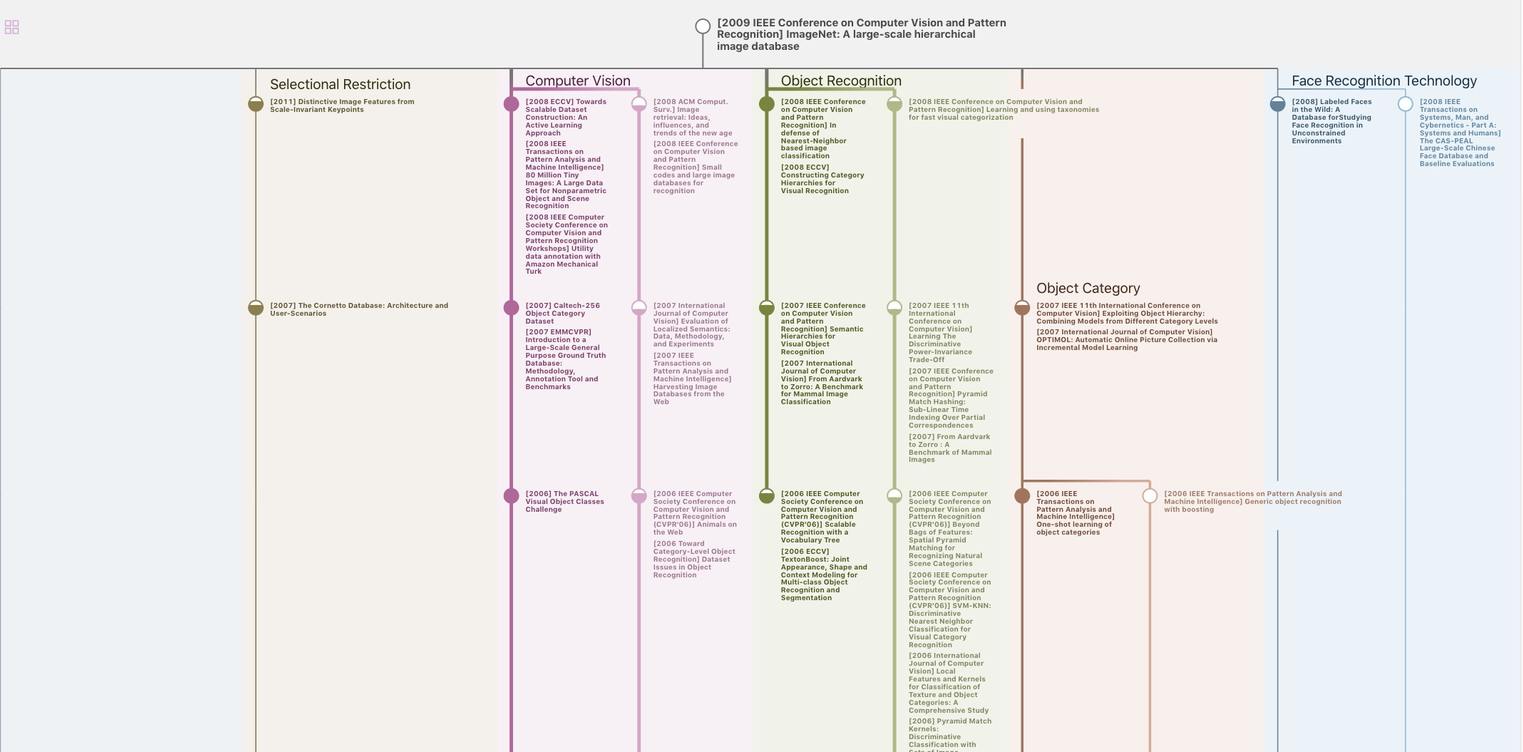
生成溯源树,研究论文发展脉络
Chat Paper
正在生成论文摘要