Big Transfer (BiT): General Visual Representation Learning Supplementary Material
semanticscholar(2020)
摘要
Throughout the paper we evaluate BiT using BiT-HyperRule. Here, we investigate whether BiT-L would benefit from additional computational budget for selecting fine-tuning hyperparameters. For this investigation we use VTAB-1k as it contains a diverse set of 19 tasks. For each task we fine-tune BiT-L 40 times using 800 training images. Each trial uses randomly sampled hyperparameters as described below. We select the best model for each dataset using the validation set with 200 images. The results are shown in fig. 1. Overall, we observe that VTAB-1k score saturates roughly after 20 trials and that further tuning results in overfitting on the validation split.
更多查看译文
AI 理解论文
溯源树
样例
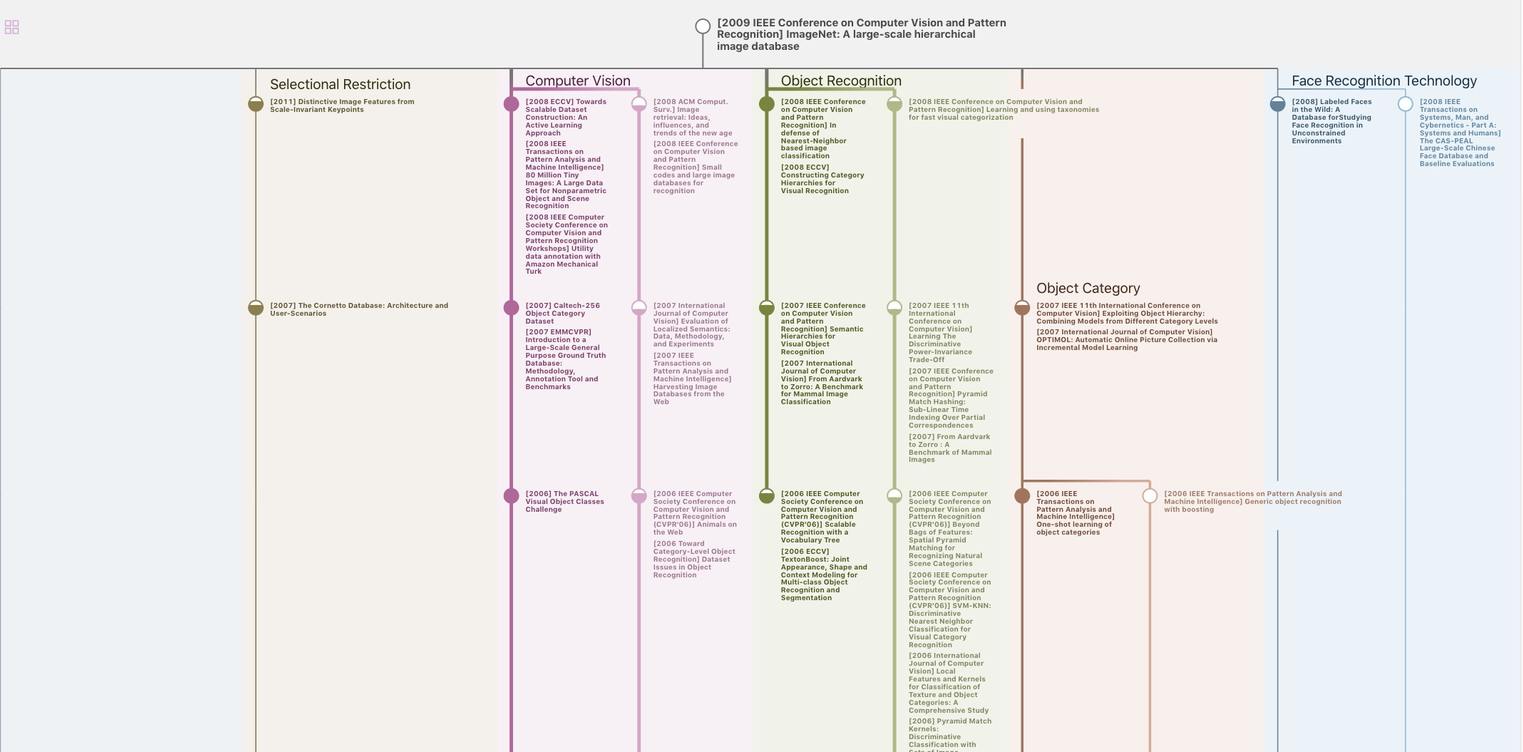
生成溯源树,研究论文发展脉络
Chat Paper
正在生成论文摘要