Automap: Towards Ergonomic Automated Parallelism for ML Models
arxiv(2021)
摘要
The rapid rise in demand for training large neural network architectures has brought into focus the need for partitioning strategies, for example by using data, model, or pipeline parallelism. Implementing these methods is increasingly supported through program primitives, but identifying efficient partitioning strategies requires expensive experimentation and expertise. We present the prototype of an automated partitioner that seamlessly integrates into existing compilers and existing user workflows. Our partitioner enables SPMD-style parallelism that encompasses data parallelism and parameter/activation sharding. Through a combination of inductive tactics and search in a platform-independent partitioning IR, automap can recover expert partitioning strategies such as Megatron sharding for transformer layers.
更多查看译文
关键词
ergonomic automated parallelism,models
AI 理解论文
溯源树
样例
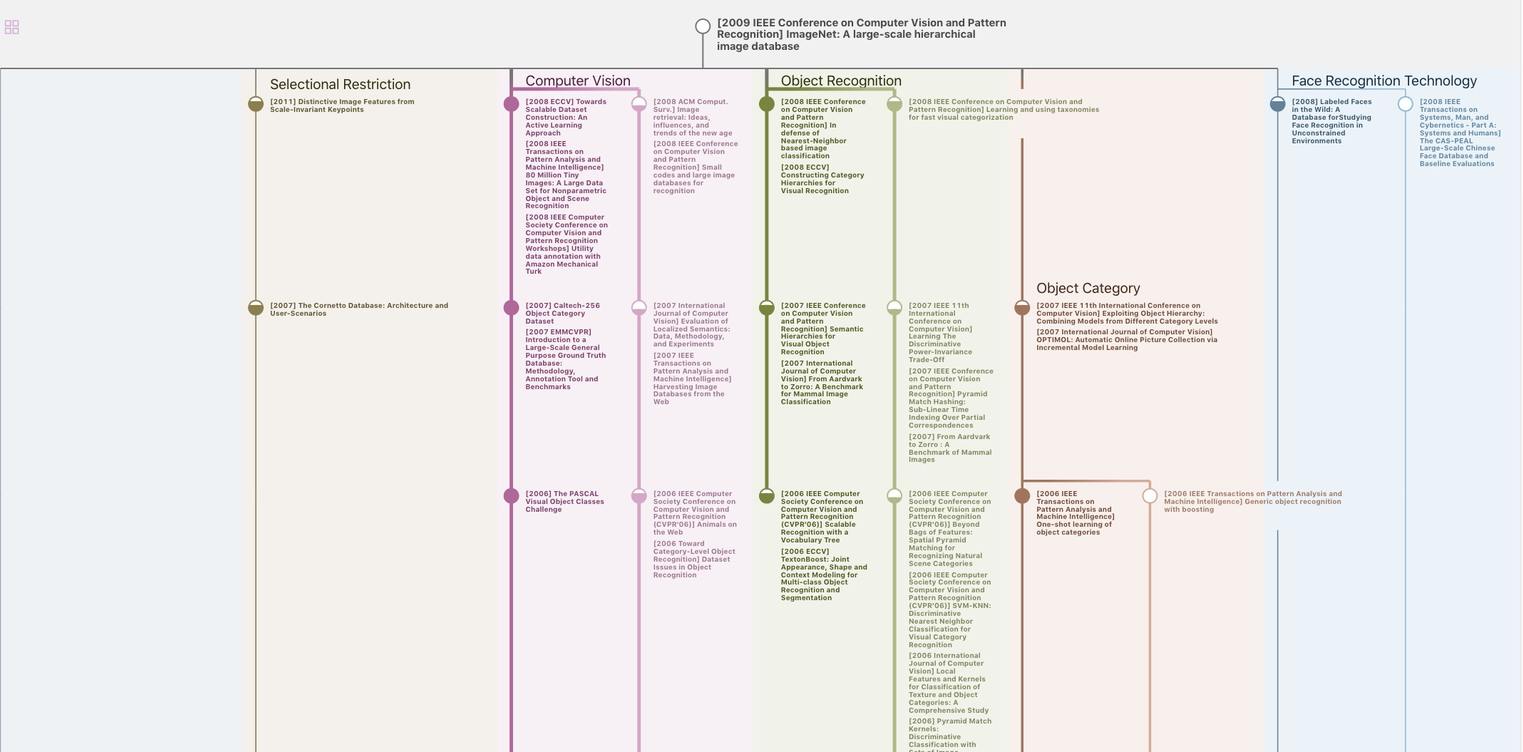
生成溯源树,研究论文发展脉络
Chat Paper
正在生成论文摘要