Variational Learning for Unsupervised Knowledge Grounded Dialogs
European Conference on Artificial Intelligence(2021)
摘要
Recent methods for knowledge grounded dialogs generate responses by incorporating information from an external textual document [Lewis et al. , 2020; Guu et al. , 2020]. These methods do not require the exact document to be known during training and rely on the use of a retrieval system to fetch relevant documents from a large index. The documents used to generate the responses are modeled as latent variables whose prior probabilities need to be estimated. Models such as RAG [ Lewis et al. , 2020 ] and REALM [ Guu et al. , 2020 ] , marginalize the document probabilities over the documents retrieved from the index to define the log likelihood loss function which is optimized end-to-end. In this paper, we develop a variational approach to the above technique wherein, we instead maximize the Evidence Lower bound (ELBO). Using a collection of three publicly available open-conversation datasets, we demonstrate how the posterior distribution, that has information from the ground-truth response, allows for a better approximation of the objective function during training. To overcome the challenges associated with sampling over a large knowledge collection, we develop an efficient approach to approximate the ELBO. To the best of our knowledge we are the first to apply variational training for open-scale unsupervised knowledge grounded dialog systems.
更多查看译文
关键词
Natural Language Processing: Dialogue and Interactive Systems,Natural Language Processing: Language Grounding
AI 理解论文
溯源树
样例
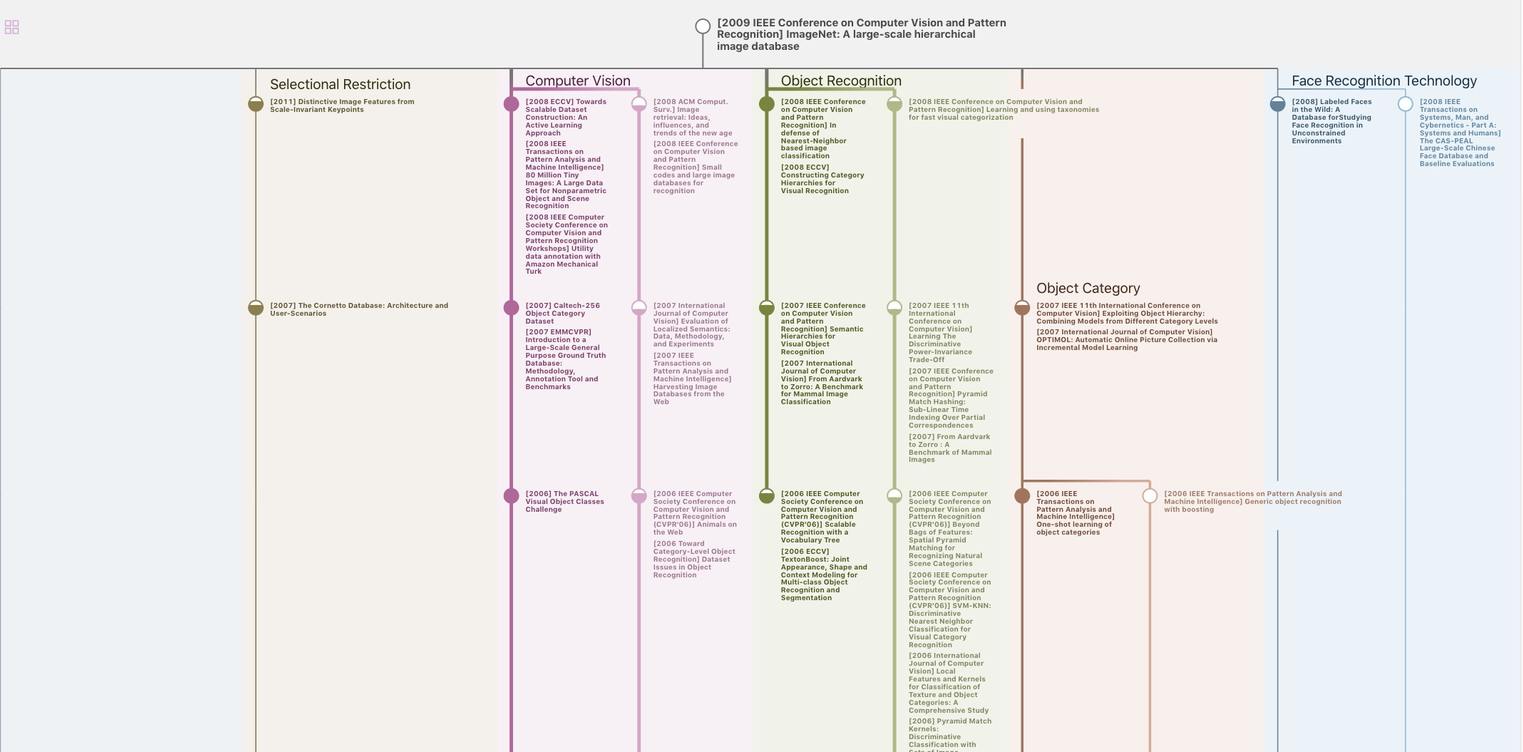
生成溯源树,研究论文发展脉络
Chat Paper
正在生成论文摘要