Design Framework For An Energy-Efficient Binary Convolutional Neural Network Accelerator Based On Nonvolatile Logic
IEICE NONLINEAR THEORY AND ITS APPLICATIONS(2021)
摘要
Convolutional neural network (CNN) accelerators, particularly binarized CNN (BCNN) accelerators have proven to be effective for several artificial-intelligence-oriented several applications; however, their energy efficiency should be further improved for edge applications. In this paper, a design framework for an energy-efficient BCNN accelerator based on nonvolatile logic is presented. Designing BCNN accelerators using nonvolatile logic allows for the accelerators to exhibit a massively parallel and ultra-low standby power capability. Thus, a new design can be realized for accelerators that is different from that of conventional accelerators based solely on CMOS. Considering this, we discuss a concrete design considerations of nonvolatile BCNN accelerators. In fact, a systematic design flow of the nonvolatile BCNN is established by combining Vivado HLS and standard electronic design automation tools. As a typical design example, a BCNN accelerator for inferring 32 x 32 pixel MNIST dataset is designed using a 65-nm CMOS technology. By the logic-synthesis result, the proposed BCNN accelerator is estimated to consume 94.2% lower power than that of a conventional BCNN accelerator when the frame rate is 30 frames per second.
更多查看译文
关键词
BCNN, HLS, EDA, ASIC, FPGA
AI 理解论文
溯源树
样例
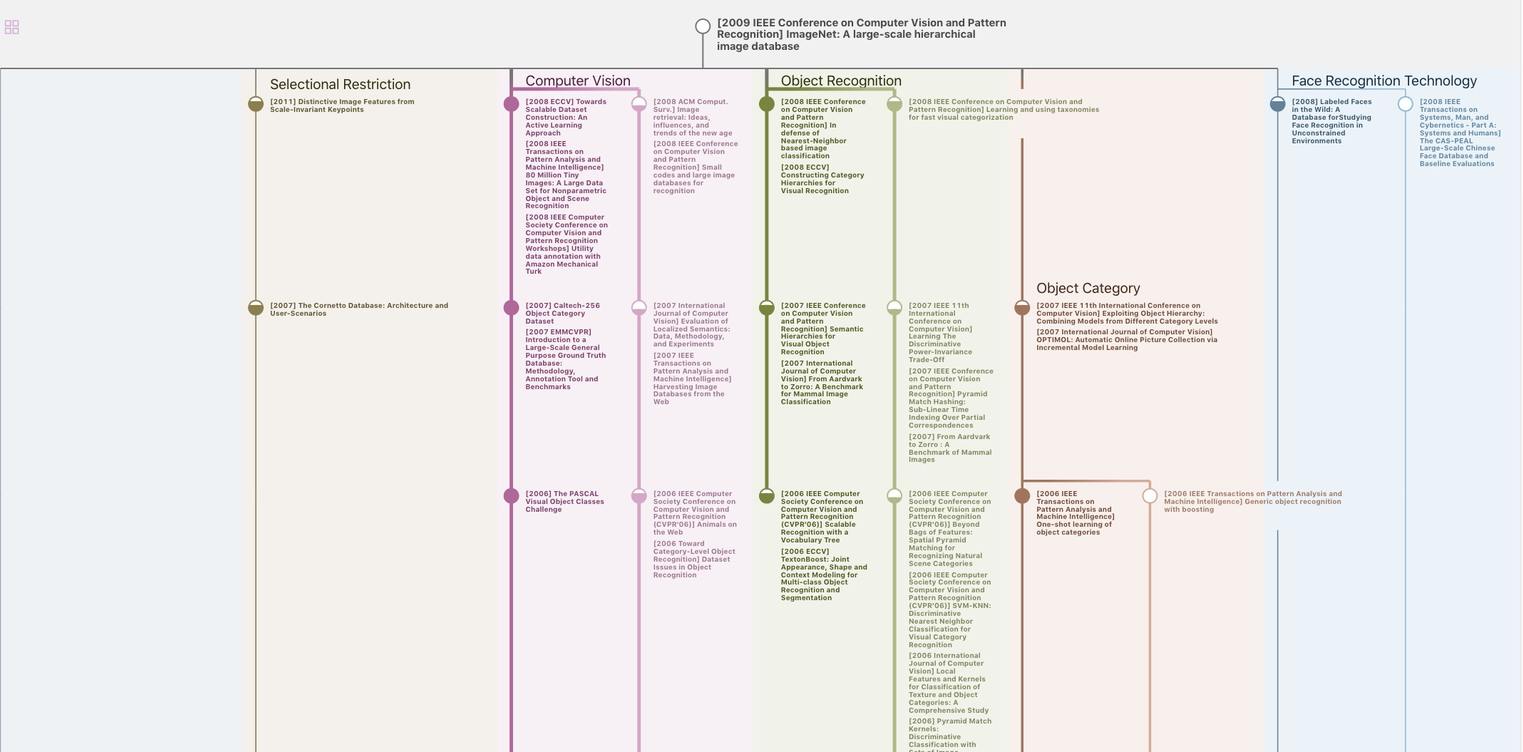
生成溯源树,研究论文发展脉络
Chat Paper
正在生成论文摘要