A scalable model for tracking topical evolution in large document collections
2017 IEEE International Conference on Big Data (Big Data)(2017)
摘要
In this era of big data, many domains on the web naturally have massive amount of labeled text data that are growing over time, for example, digital publication archives, social media posts, and question-answer forums. Probabilistic graphical models have shown great potential for mining such text corpora in recent years. Some of these algorithms utilize explicit annotations and labels associated with documents to guide the probabilistic model to find hidden themes. A few techniques attempt to utilize the timestamps associated with documents to model the evolution of those latent topics. However, no effort has been devoted to utilize these two different dimensions of information together — timestamps and labels or annotations — to discover evolution of labeled themes. In this paper, we present a new topical model called the Supervised Topical Evolution Model (STEM), which is a monolithic graphical model capable of using annotations, timestamps, and textual contents to discover interpretable and evolving themes from big text datasets. STEM simultaneously learns latent themes and their changes over time using a stochastic process that is driven by labels or annotations. In addition, we provide an asynchronously distributed inference process for STEM that results in significant speedup in learning time, making the model scalable for large datasets. Extensive experiments demonstrate that our proposed model is able to infer highly interpretable topics that reflect temporal patterns, in much less time than other comparable topic modeling methods.
更多查看译文
关键词
Scalability,Graphical Models,Temporal Topic Modeling,Probabilistic Inference
AI 理解论文
溯源树
样例
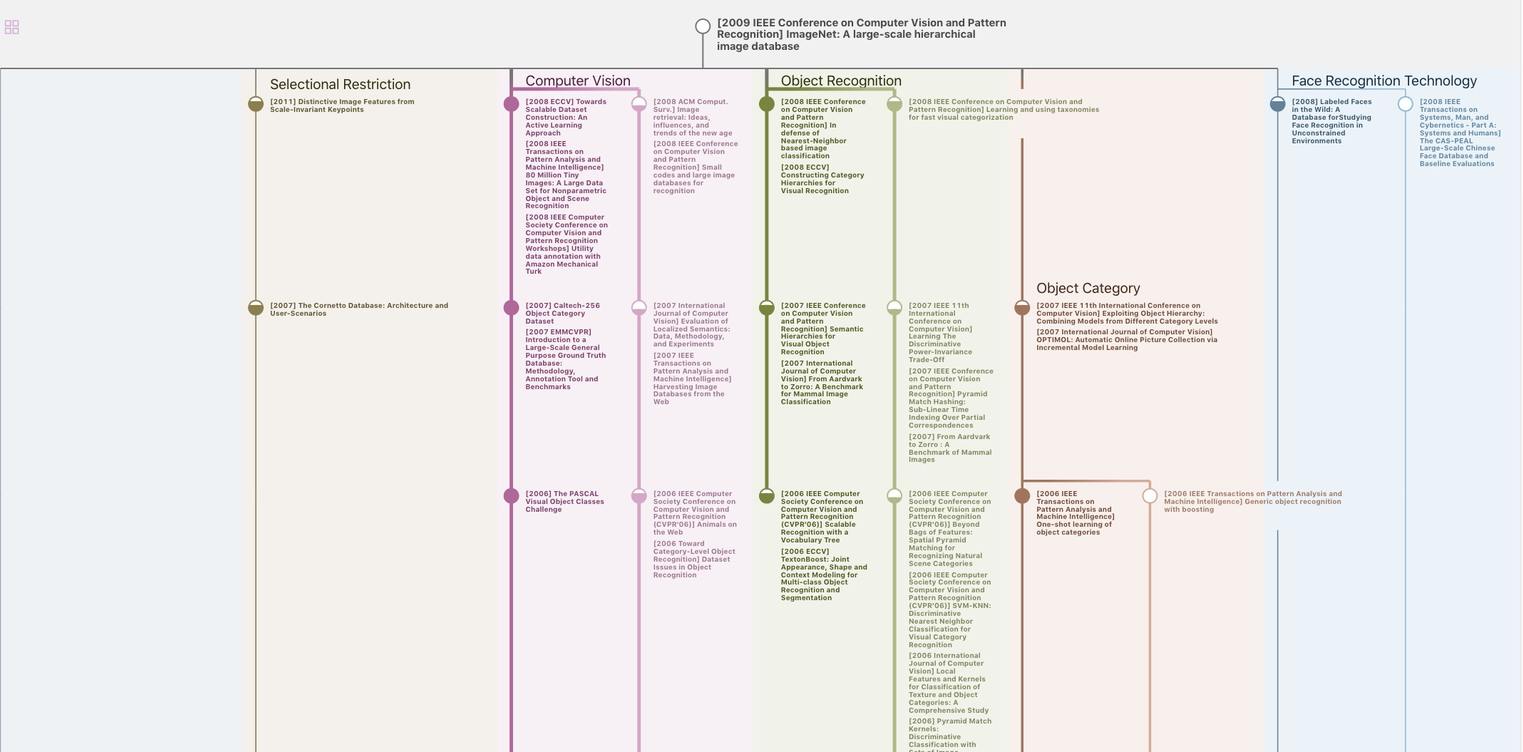
生成溯源树,研究论文发展脉络
Chat Paper
正在生成论文摘要