Exact Decomposition of Joint Low Rankness and Local Smoothness Plus Sparse Matrices.
IEEE transactions on pattern analysis and machine intelligence(2023)
摘要
It is known that the decomposition in low-rank and sparse matrices (L+S for short) can be achieved by several Robust PCA techniques. Besides the low rankness, the local smoothness (LSS) is a vitally essential prior for many real-world matrix data such as hyperspectral images and surveillance videos, which makes such matrices have low-rankness and local smoothness property at the same time. This poses an interesting question: Can we make a matrix decomposition in terms of L&LSS +S form exactly? To address this issue, we propose in this paper a new RPCA model based on three-dimensional correlated total variation regularization (3DCTV-RPCA for short) by fully exploiting and encoding the prior expression underlying such joint low-rank and local smoothness matrices. Specifically, using a modification of Golfing scheme, we prove that under some mild assumptions, the proposed 3DCTV-RPCA model can decompose both components exactly, which should be the first theoretical guarantee among all such related methods combining low rankness and local smoothness. In addition, by utilizing Fast Fourier Transform (FFT), we propose an efficient ADMM algorithm with a solid convergence guarantee for solving the resulting optimization problem. Finally, a series of experiments on both simulations and real applications are carried out to demonstrate the general validity of the proposed 3DCTV-RPCA model.
更多查看译文
关键词
Exact recovery guarantee,joint low-rank and local smoothness matrices,correlated total variation regularization,3DCTV-RPCA,Fast Fourier Transform (FFT),convergence guarantee
AI 理解论文
溯源树
样例
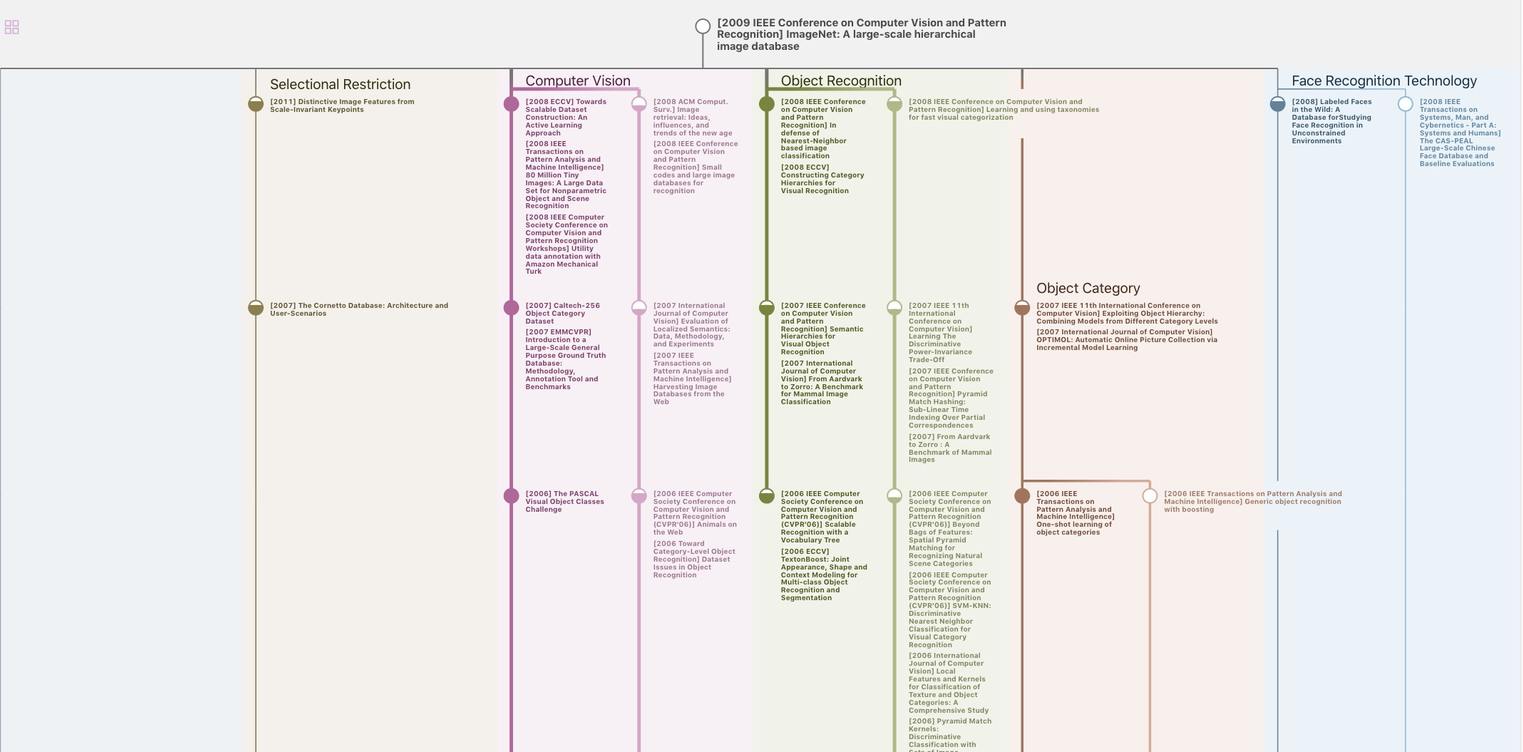
生成溯源树,研究论文发展脉络
Chat Paper
正在生成论文摘要