Interactive Affordance Learning through Manipulation Relationship Graph Construction
arxiv(2021)
摘要
In this paper, we propose Manipulation Relationship Graph (MRG), a novel affordance representation which captures the underlying manipulation relationships of an arbitrary scene. To construct such graph from raw visual observation, a deep nerual network named AffRel is introduced. It consists of a Attribute and Context module, which guide the relationship learning at instance and subgraph level respectively. We quantitatively validate our method on a novel manipulation relationship dataset named SMRD dataset. To evaluate the performance of proposed model and representation, both visual and physical experiments are conducted. Overall, AffRel along with MRG outperforms all baselines, achieving the success rate of 88.89% on task relationship recognition (TRR) and 73.33% on task completion (TC). It demonstrates its superior capability to reason about the affordance in an interactive way for the purpose of robotic manipulation.
更多查看译文
AI 理解论文
溯源树
样例
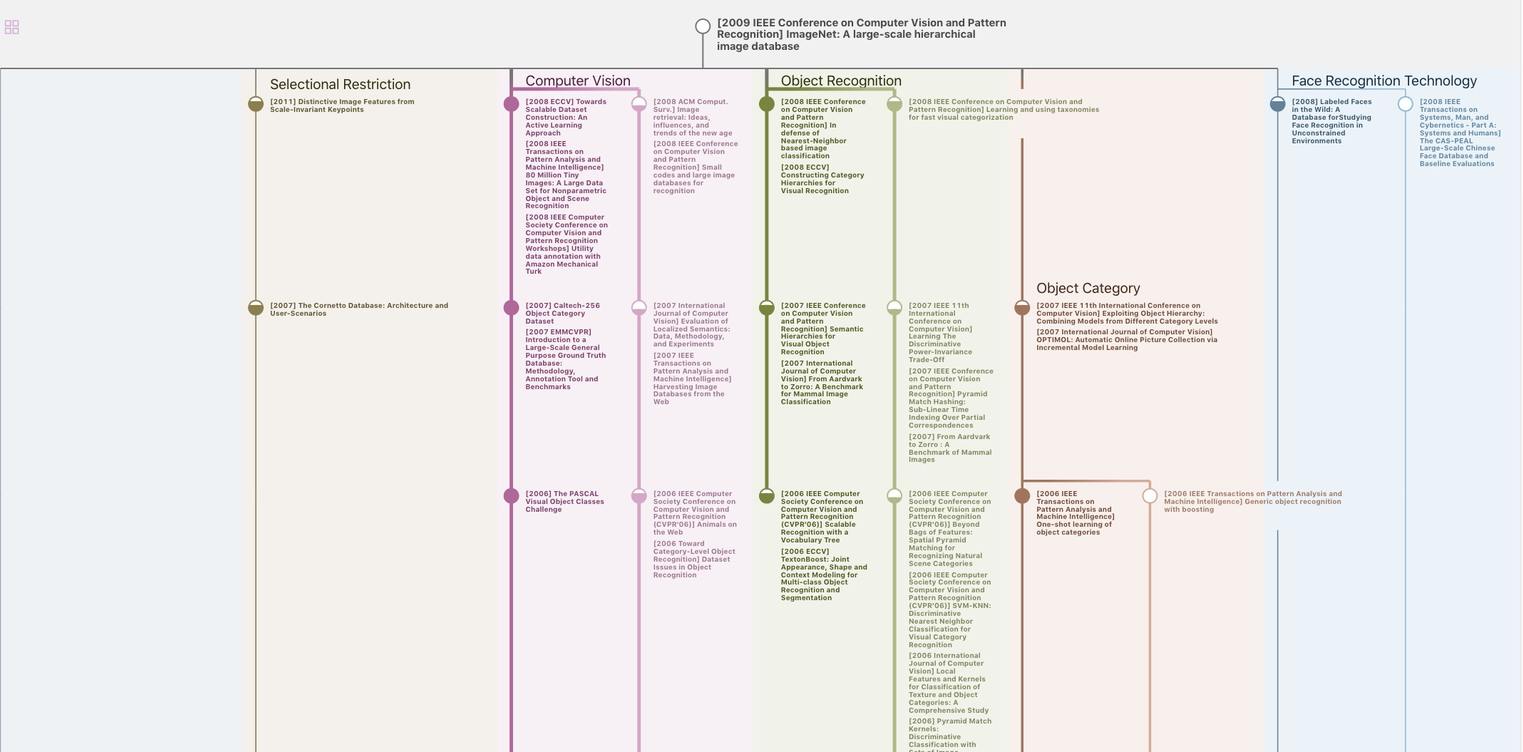
生成溯源树,研究论文发展脉络
Chat Paper
正在生成论文摘要