LiDAR few-shot domain adaptation via integrated CycleGAN and 3D object detector with joint learning delay
2021 IEEE INTERNATIONAL CONFERENCE ON ROBOTICS AND AUTOMATION (ICRA 2021)(2021)
摘要
The success of supervised LiDAR perception methods relies on the availability of large sets of labeled point cloud data, for which the labeling process is costly and time consuming. Given unpaired LiDAR datasets of similar sizes from two domains, with one (source) containing task-specific labels e.g. 3D bounding boxes for all frames, but only a small percentage of frames being labeled in the other (target) domain, it is challenging to train a model that generalizes well on validation data from the target domain. In this paper we propose a novel LiDAR few-shot domain adaptation architecture and training strategy to address this challenge. Our method is based on adapting a task-specific network (3D object detector) to work within the CycleGAN framework modified to operate with LiDAR features, and on the joint end-to-end training of generators, discriminators, and task-specific layers. To overcome nonconvergence issues we propose a training strategy that introduces a mechanism to delay the joint learning between the generators/discriminators and the task-specific network by allowing them to start learning independently, while slowly introducing joint learning as they converge, hence avoiding instability during the early stages of the training. Our proposed integrated architecture enables a direct way to evaluate the performance of the model instead of feeding pre-computed generated data into a separate pre-trained model. We include an experimental section where we evaluate our proposed architecture on the publicly available KITTI and Nuscenes datasets, as well as on our own labeled dataset. We present useful mean average precision plots that illustrate the benefits of our domain adaptation architecture as a function of number of labeled target domain frames.
更多查看译文
关键词
task-specific network,integrated architecture,labeled target domain frames,integrated CycleGAN,3D object detector,joint learning delay,supervised LiDAR perception methods,labeled point cloud data,labeling process,CycleGAN framework,LiDAR features,end-to-end training,task-specific layers,unpaired LiDAR datasets,LiDAR few-shot domain adaptation architecture
AI 理解论文
溯源树
样例
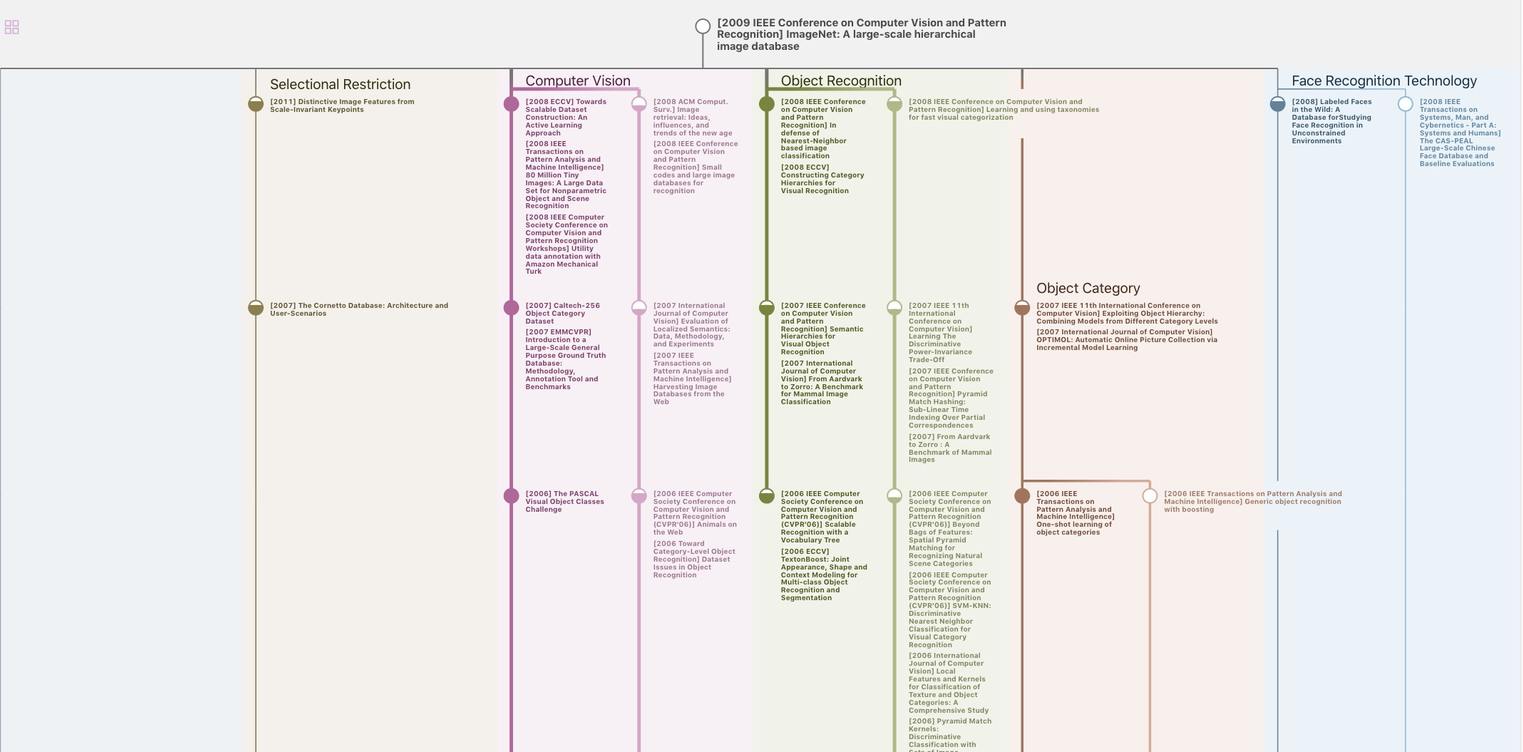
生成溯源树,研究论文发展脉络
Chat Paper
正在生成论文摘要