Long Expressive Memory for Sequence Modeling
International Conference on Learning Representations (ICLR)(2022)
摘要
We propose a novel method called Long Expressive Memory (LEM) for learning long-term sequential dependencies. LEM is gradient-based, it can efficiently process sequential tasks with very long-term dependencies, and it is sufficiently expressive to be able to learn complicated input-output maps. To derive LEM, we consider a system of multiscale ordinary differential equations, as well as a suitable time-discretization of this system. For LEM, we derive rigorous bounds to show the mitigation of the exploding and vanishing gradients problem, a well-known challenge for gradient-based recurrent sequential learning methods. We also prove that LEM can approximate a large class of dynamical systems to high accuracy. Our empirical results, ranging from image and time-series classification through dynamical systems prediction to speech recognition and language modeling, demonstrate that LEM outperforms state-of-the-art recurrent neural networks, gated recurrent units, and long short-term memory models.
更多查看译文
关键词
sequence modeling,long-term dependencies,multiscale ordinary differential equations,dynamical systems
AI 理解论文
溯源树
样例
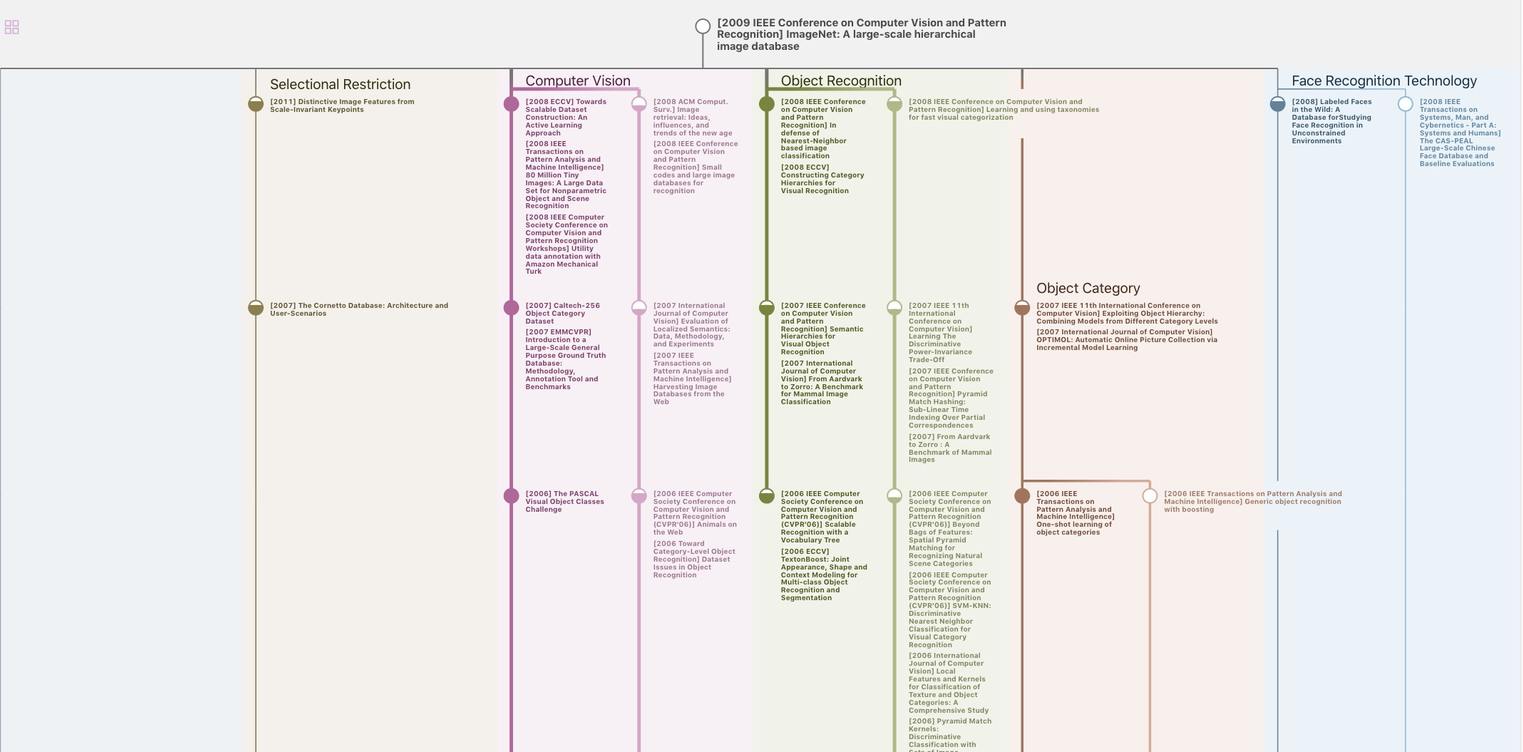
生成溯源树,研究论文发展脉络
Chat Paper
正在生成论文摘要