Grounded, Controllable and Debiased Image Completion with Lexical Semantics
2021 IEEE/CVF CONFERENCE ON COMPUTER VISION AND PATTERN RECOGITION WORKSHOPS (CVPRW 2021)(2021)
摘要
In this paper, we present an approach, namely Lexical Semantic Image Completion (LSIC)(1), that may have potential applications in art, design, and heritage conservation, among several others. Existing image completion procedure is highly subjective by considering only visual context, which may trigger unpredictable results which are plausible but not faithful to a grounded knowledge. To permit both grounded and controllable completion process, we advocate generating results faithful to both visual and lexical semantic context, i.e., the description of leaving holes or blank regions in the image (e.g., hole description). One major challenge for LSIC comes from modeling and aligning the structure of visual-semantic context and translating across different modalities. We devise multi-grained reasoning blocks to address this challenge. Another challenge relates to the unimodal biases, which occurs when the model generates plausible results without using the textual description. We devise an unsupervised unpaired-creation learning path that explicitly performs counterfactual thinking, i.e., what the complete image would be if given an unpaired text description to the incomplete image. A cycle consistency loss is devised to guarantee counterfactual faithfulness. We conduct extensive quantitative and qualitative experiments that reveal the strengths of LSIC in being grounded, controllable, and debiased.
更多查看译文
关键词
LSIC,heritage conservation,grounded knowledge,lexical semantic context,hole description,visual-semantic context,multigrained reasoning blocks,textual description,unpaired text description,counterfactual faithfulness,lexical semantic image completion,debiased image completion,controllable image completion,grounded image completion,blank regions,unimodal biases,unsupervised unpaired-creation learning path,counterfactual thinking,cycle consistency loss
AI 理解论文
溯源树
样例
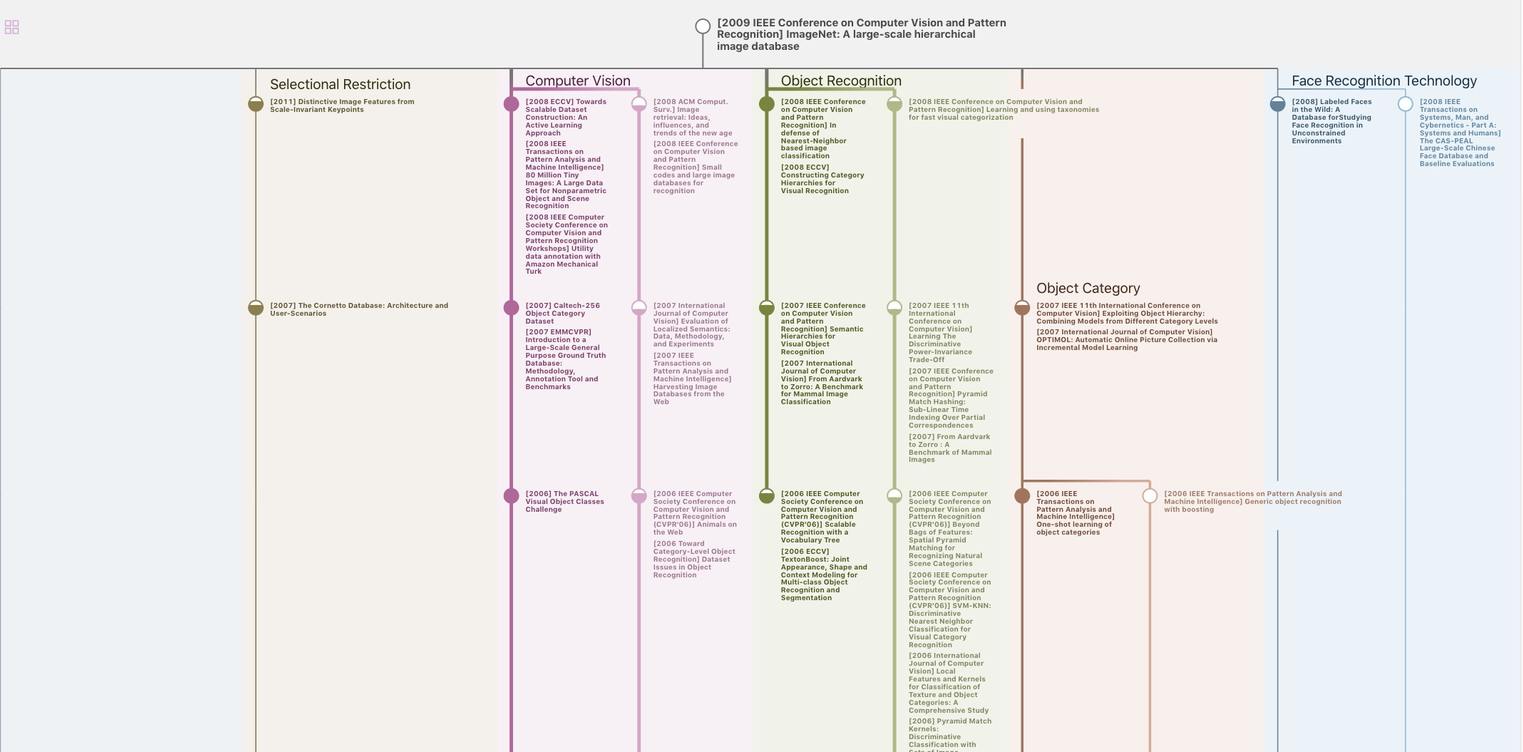
生成溯源树,研究论文发展脉络
Chat Paper
正在生成论文摘要