Teaching Agents how to Map: Spatial Reasoning for Multi-Object Navigation.
IEEE/RJS International Conference on Intelligent RObots and Systems (IROS)(2022)
摘要
In the context of visual navigation, the capacity to map a novel environment is necessary for an agent to exploit its observation history in the considered place and efficiently reach known goals. This ability can be associated with spatial reasoning, where an agent is able to perceive spatial relationships and regularities, and discover object affordances. In classical Reinforcement Learning (RL) setups, this capacity is learned from reward alone. We introduce supplementary supervision in the form of auxiliary tasks designed to favor the emergence of spatial perception capabilities in agents trained for a goal-reaching downstream objective. We show that learning to estimate metrics quantifying the spatial relationships between an agent at a given location and a goal to reach has a high positive impact in Multi-Object Navigation settings. Our method significantly improves the performance of different baseline agents, that either build an explicit or implicit representation of the environment, even matching the performance of incomparable oracle agents taking ground-truth maps as input.
更多查看译文
关键词
spatial reasoning,agents,teaching,multi-object
AI 理解论文
溯源树
样例
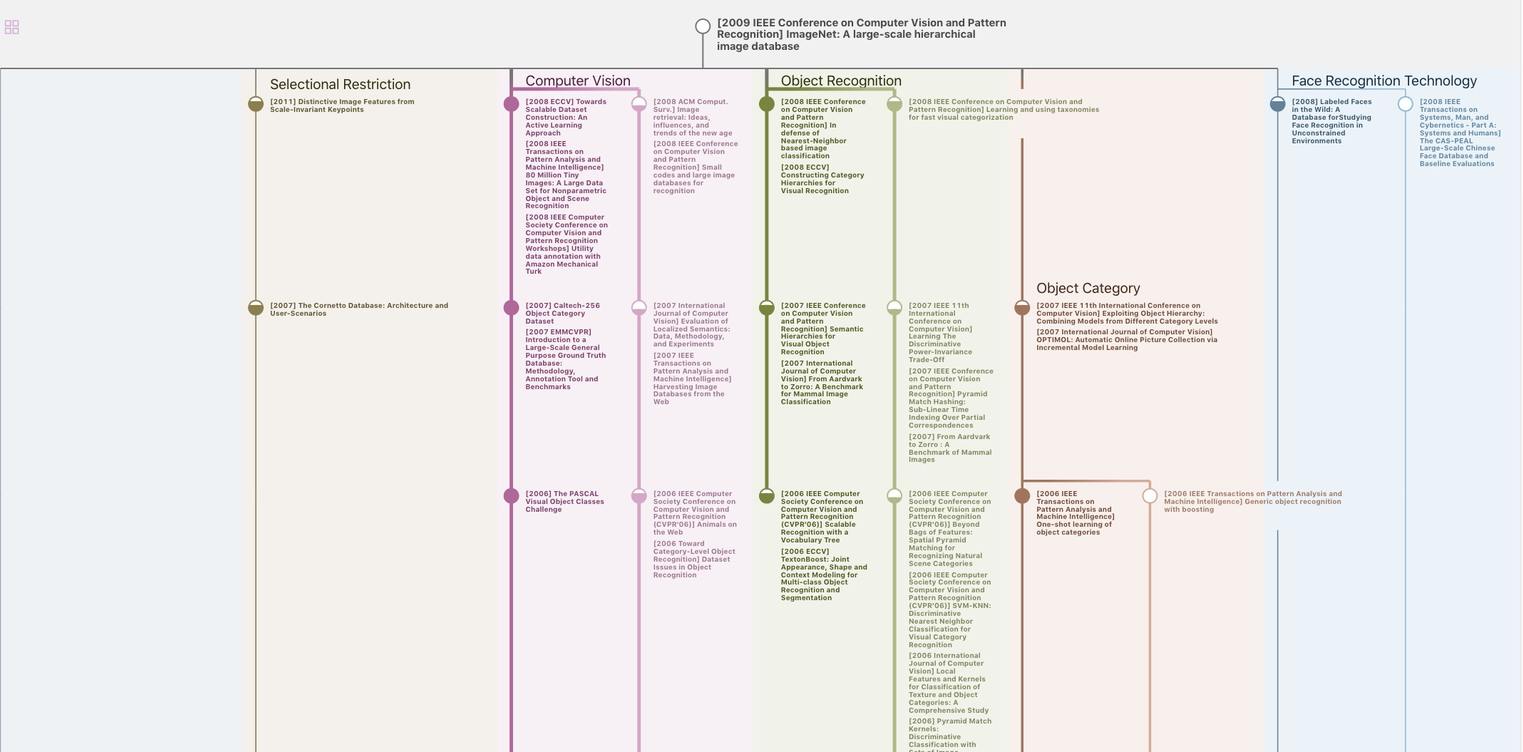
生成溯源树,研究论文发展脉络
Chat Paper
正在生成论文摘要