Attention-based multi-label neural networks for integrated prediction and interpretation of twelve widely occurring RNA modifications
NATURE COMMUNICATIONS(2021)
摘要
Recent studies suggest that epi-transcriptome regulation via post-transcriptional RNA modifications is vital for all RNA types. Precise identification of RNA modification sites is essential for understanding the functions and regulatory mechanisms of RNAs. Here, we present MultiRM, a method for the integrated prediction and interpretation of post-transcriptional RNA modifications from RNA sequences. Built upon an attention-based multi-label deep learning framework, MultiRM not only simultaneously predicts the putative sites of twelve widely occurring transcriptome modifications (m 6 A, m 1 A, m 5 C, m 5 U, m 6 Am, m 7 G, Ψ, I, Am, Cm, Gm, and Um), but also returns the key sequence contents that contribute most to the positive predictions. Importantly, our model revealed a strong association among different types of RNA modifications from the perspective of their associated sequence contexts. Our work provides a solution for detecting multiple RNA modifications, enabling an integrated analysis of these RNA modifications, and gaining a better understanding of sequence-based RNA modification mechanisms.
更多查看译文
关键词
rna,modifications,attention-based,multi-label
AI 理解论文
溯源树
样例
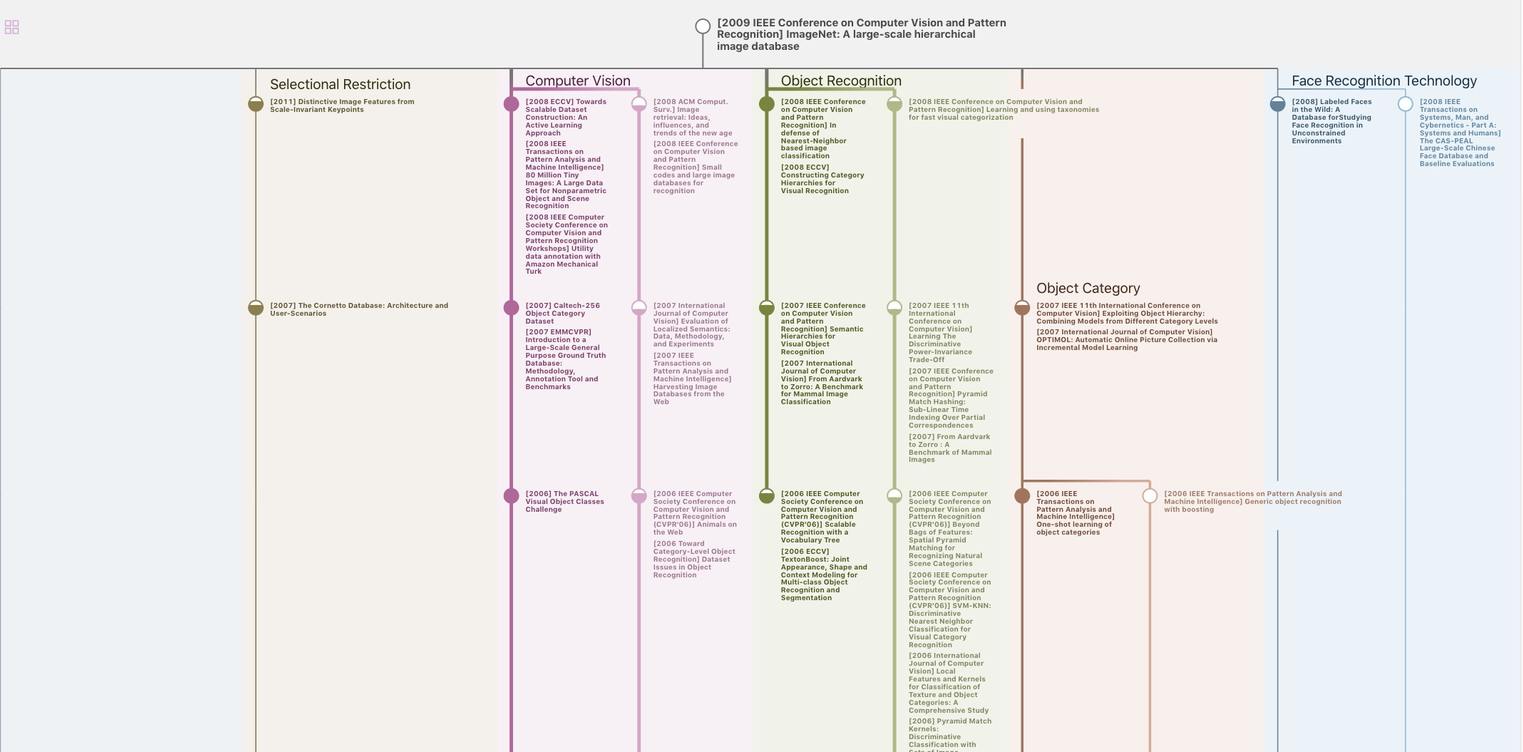
生成溯源树,研究论文发展脉络
Chat Paper
正在生成论文摘要