Gift: Generalizable Interaction-Aware Functiona Tool Affordances Without Labels
ROBOTICS: SCIENCE AND SYSTEM XVII(2021)
摘要
Tool use requires reasoning about the lit between an object's affordances and the demands of a task. Visual affordance learning can benefit from goal-directed interaction experience, but current techniques rely on human labels or expert demonstrations to generate this data. In this paper, we describe a method that grounds affordances in physical interactions instead, thus removing the need for human labels or expert policies. We use an efficient sampling-based method to generate successful trajectories that provide contact data, which are then used to reveal aflbrdance representations. Our framework, GIFT, operates in two phases: first, we discover visual affordances from goal-directed interaction with a set of procedurally generated tools; second, we train a model to predict new instances of the discovered affordances on novel tools in a self-supervised fashion. In our experiments, we show that GIFT can leverage a sparse keypoint representation to predict grasp and interaction points to accommodate multiple tasks, such as hooking, reaching, and hammering. GIFT outperforms baselines on all tasks and matches a human oracle on two of three tasks using novel tools. Qualitative results available at: www.pair.toronto.edu/gift-tools-rss21.
更多查看译文
关键词
functional,gift,interaction-aware
AI 理解论文
溯源树
样例
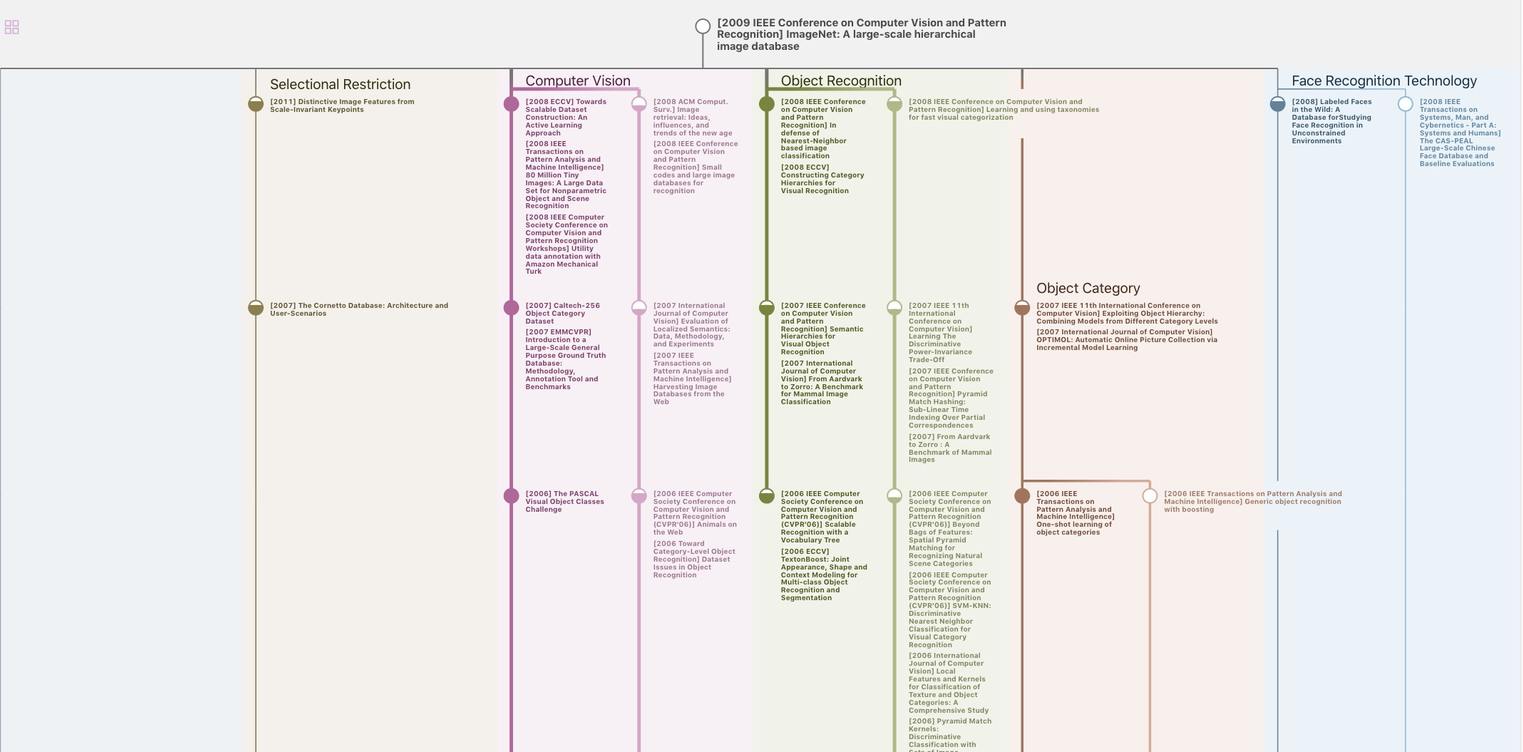
生成溯源树,研究论文发展脉络
Chat Paper
正在生成论文摘要