Enabling Rapid Covid-19 Small Molecule Drug Design Through Scalable Deep Learning Of Generative Models
INTERNATIONAL JOURNAL OF HIGH PERFORMANCE COMPUTING APPLICATIONS(2021)
摘要
We improved the quality and reduced the time to produce machine learned models for use in small molecule antiviral design. Our globally asynchronous multi-level parallel training approach strong scales to all of Sierra with up to 97.7% efficiency. We trained a novel, character-based Wasserstein autoencoder that produces a higher quality model trained on 1.613 billion compounds in 23 minutes while the previous state of the art takes a day on 1 million compounds. Reducing training time from a day to minutes shifts the model creation bottleneck from computer job turnaround time to human innovation time. Our implementation achieves 318 PFLOPs for 17.1% of half-precision peak. We will incorporate this model into our molecular design loop enabling the generation of more diverse compounds; searching for novel, candidate antiviral drugs improves and reduces the time to synthesize compounds to be tested in the lab.
更多查看译文
关键词
COVID 19, machine learning, scalable performance, generative models, drug design
AI 理解论文
溯源树
样例
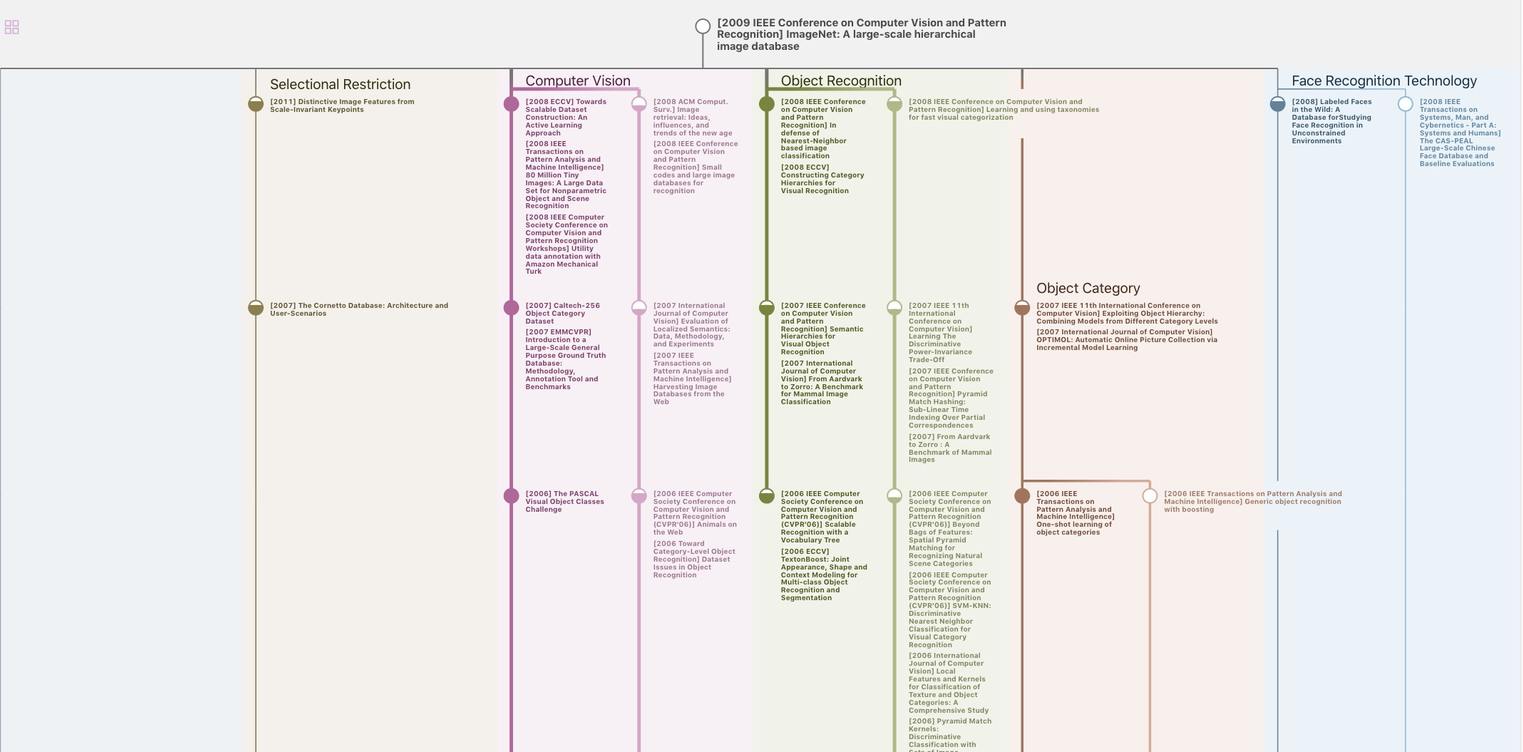
生成溯源树,研究论文发展脉络
Chat Paper
正在生成论文摘要