Nonconvex Robust High-Order Tensor Completion Using Randomized Low-Rank Approximation
IEEE TRANSACTIONS ON IMAGE PROCESSING(2024)
摘要
Within the tensor singular value decomposition (T-SVD) framework, existing robust low-rank tensor completion approaches have made great achievements in various areas of science and engineering. Nevertheless, these methods involve the T-SVD based low-rank approximation, which suffers from high computational costs when dealing with large-scale tensor data. Moreover, most of them are only applicable to third-order tensors. Against these issues, in this article, two efficient low-rank tensor approximation approaches fusing random projection techniques are first devised under the order-d ( d >= 3 ) T-SVD framework. Theoretical results on error bounds for the proposed randomized algorithms are provided. On this basis, we then further investigate the robust high-order tensor completion problem, in which a double nonconvex model along with its corresponding fast optimization algorithms with convergence guarantees are developed. Experimental results on large-scale synthetic and real tensor data illustrate that the proposed method outperforms other state-of-the-art approaches in terms of both computational efficiency and estimated precision.
更多查看译文
关键词
High-order T-SVD framework,robust high-order tensor completion,randomized low-rank tensor approximation,nonconvex regularizers,ADMM algorithm
AI 理解论文
溯源树
样例
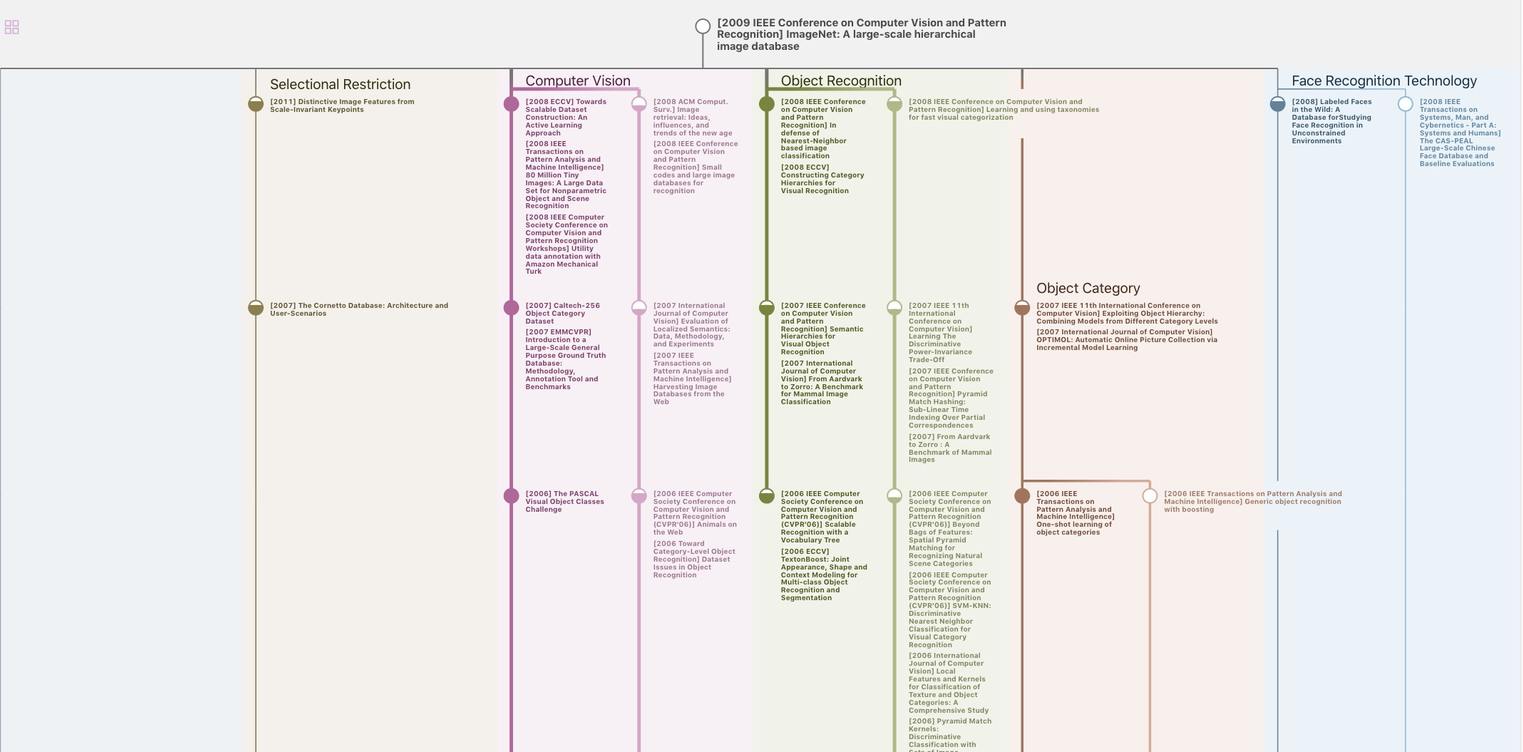
生成溯源树,研究论文发展脉络
Chat Paper
正在生成论文摘要