GLiDE: Generalizable Quadrupedal Locomotion in Diverse Environments with a Centroidal Model
WAFR(2023)
摘要
Model-free reinforcement learning (RL) for legged locomotion commonly relies on a physics simulator that can accurately predict the behaviors of every degree of freedom of the robot. In contrast, approximate reduced-order models are commonly used for many model predictive control strategies. In this work we abandon the conventional use of high-fidelity dynamics models in RL and we instead seek to understand what can be achieved when using RL with a much simpler centroidal model when applied to quadrupedal locomotion. We show that RL-based control of the accelerations of a centroidal model is surprisingly effective, when combined with a quadratic program to realize the commanded actions via ground contact forces. It allows for a simple reward structure, reduced computational costs, and robust sim-to-real transfer. We show the generality of the method by demonstrating flat-terrain gaits, stepping-stone locomotion, two-legged in-place balance, balance beam locomotion, and direct sim-to-real transfer.
更多查看译文
关键词
Legged locomotion, Reinforcement learning, Centroidal model
AI 理解论文
溯源树
样例
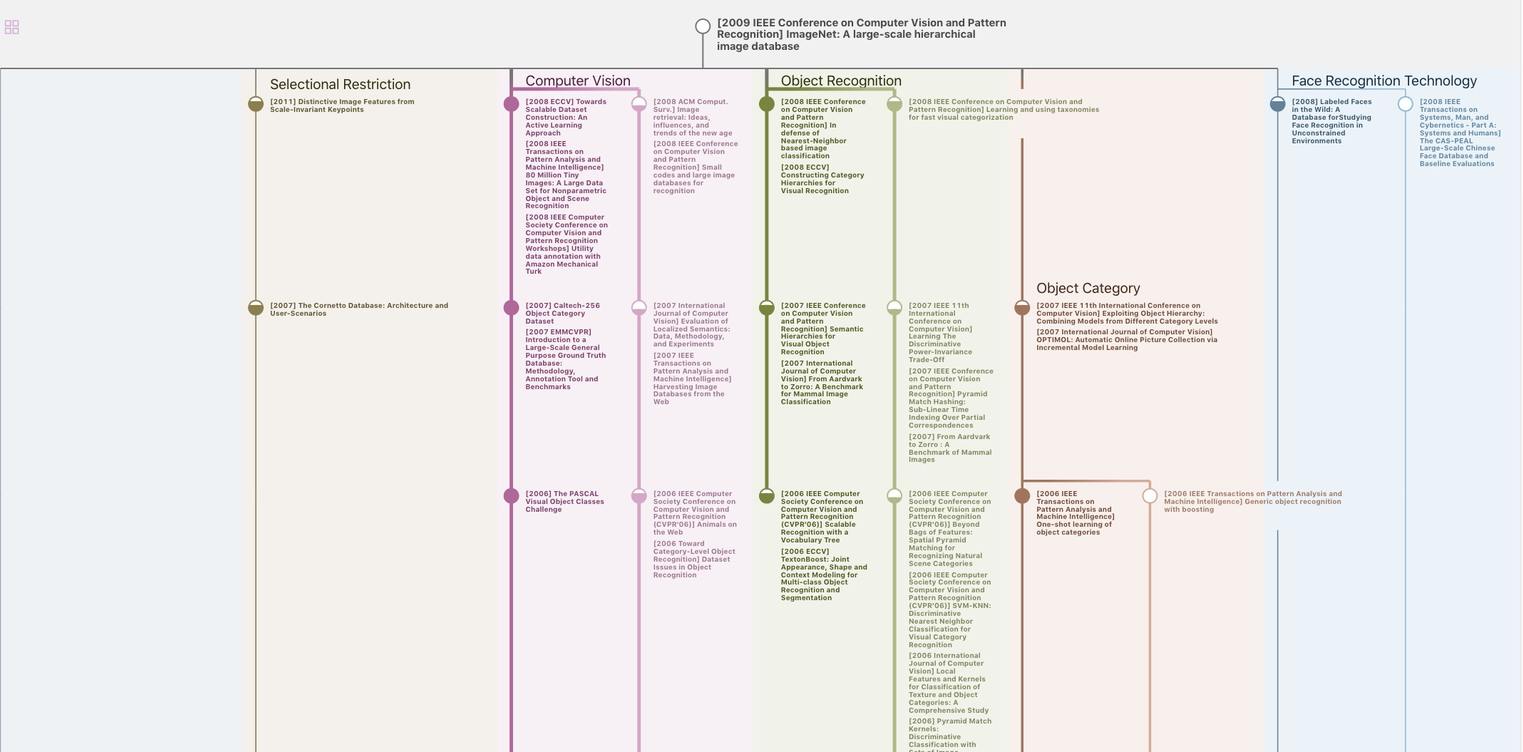
生成溯源树,研究论文发展脉络
Chat Paper
正在生成论文摘要