Imperfect also Deserves Reward: Multi-Level and Sequential Reward Modeling for Better Dialog Management
NAACL-HLT(2021)
摘要
For task-oriented dialog systems, training a Reinforcement Learning (RL) based Dialog Management module suffers from low sample efficiency and slow convergence speed due to the sparse rewards in RL.To solve this problem, many strategies have been proposed to give proper rewards when training RL, but their rewards lack interpretability and cannot accurately estimate the distribution of state-action pairs in real dialogs. In this paper, we propose a multi-level reward modeling approach that factorizes a reward into a three-level hierarchy: domain, act, and slot. Based on inverse adversarial reinforcement learning, our designed reward model can provide more accurate and explainable reward signals for state-action pairs.Extensive evaluations show that our approach can be applied to a wide range of reinforcement learning-based dialog systems and significantly improves both the performance and the speed of convergence.
更多查看译文
关键词
sequential reward,multi-level
AI 理解论文
溯源树
样例
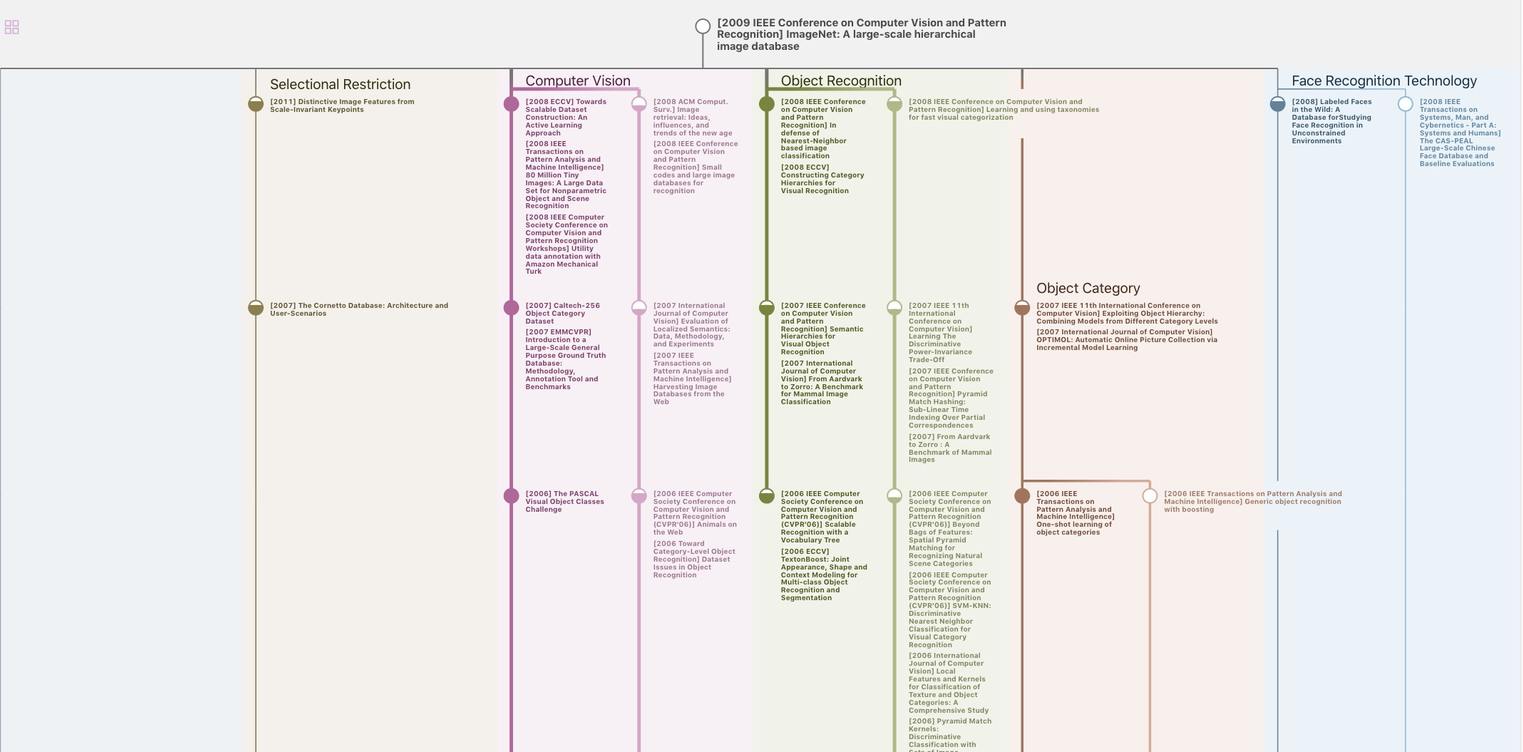
生成溯源树,研究论文发展脉络
Chat Paper
正在生成论文摘要