TransMOT: Spatial-Temporal Graph Transformer for Multiple Object Tracking
arxiv(2023)
摘要
Tracking multiple objects in videos relies on modeling the spatial-temporal interactions of the objects. In this paper, we propose TransMOT, which leverages powerful graph transformers to efficiently model the spatial and temporal interactions among the objects. TransMOT is capable of effectively modeling the interactions of a large number of objects by arranging the trajectories of the tracked targets and detection candidates as a set of sparse weighted graphs, and constructing a spatial graph transformer encoder layer, a temporal transformer encoder layer, and a spatial graph transformer decoder layer based on the graphs. Through end-to-end learning, TransMOT can exploit the spatial-temporal clues to directly estimate association from a large number of loosely filtered detection predictions for robust MOT in complex scenes. The proposed method is evaluated on multiple benchmark datasets, including MOT15, MOT16, MOT17, and MOT20, and it achieves state-of-the-art performance on all the datasets.
更多查看译文
关键词
Transformer (machine learning model),Video tracking,Encoder,Benchmark (computing),Association (object-oriented programming),Computer vision,Set (abstract data type),Layer (object-oriented design),Tracking (particle physics),Computer science,Artificial intelligence
AI 理解论文
溯源树
样例
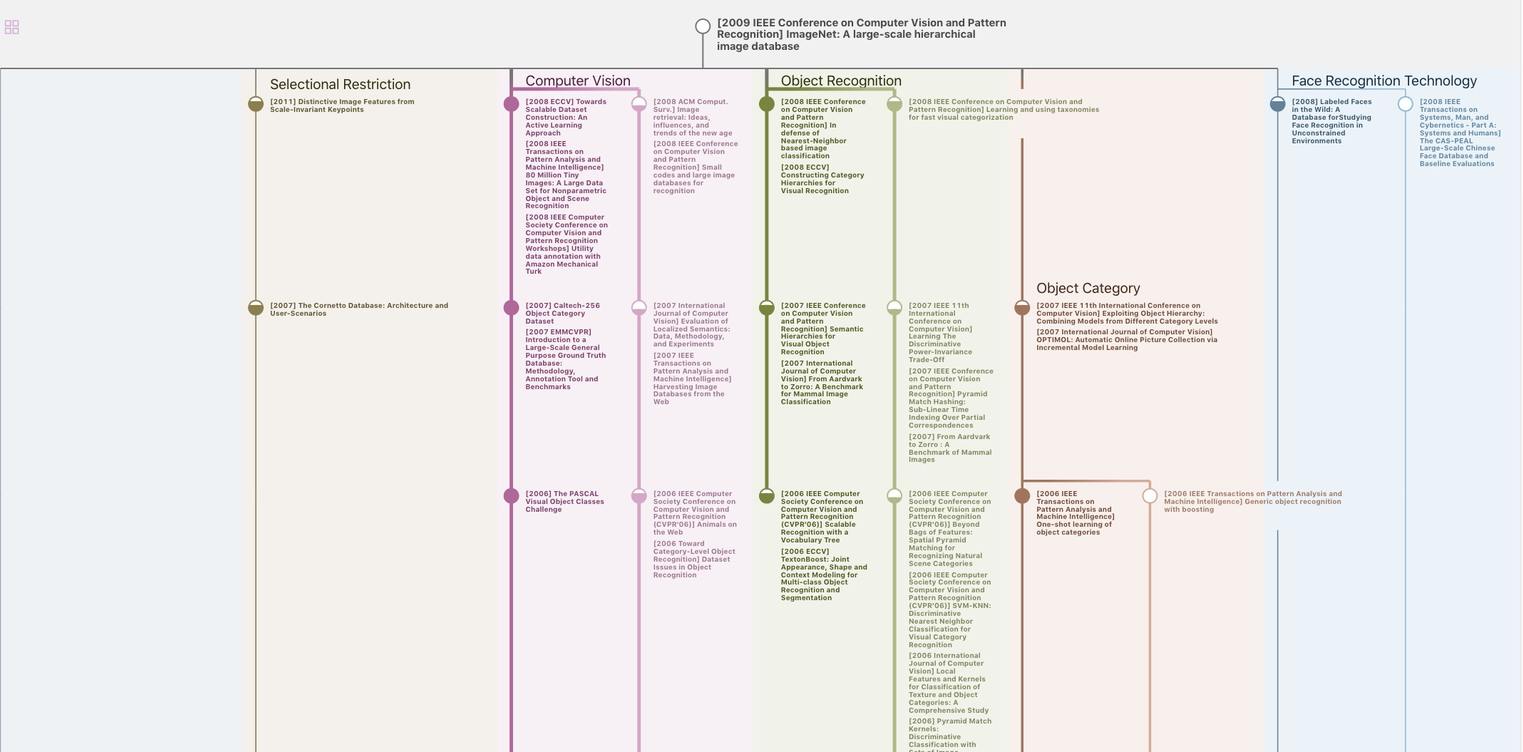
生成溯源树,研究论文发展脉络
Chat Paper
正在生成论文摘要