Age-Invariant Speaker Embedding for Diarization of Cognitive Assessments
2021 12th International Symposium on Chinese Spoken Language Processing (ISCSLP)(2021)
摘要
This paper investigates an age-invariant speaker embedding approach to speaker diarization, which is an essential step towards the automatic cognitive assessments from speech. Studies have shown that incorporating speaker traits (e.g., age, gender, etc.) can improve speaker diarization performance. However, we found that age information in the speaker embeddings is detrimental to speaker diarization if there is a severe mismatch between the age distributions in the training data and test data. To minimize the detrimental effect of age mismatch, an adversarial training strategy is introduced to remove age variability from the utterance-level speaker embeddings. Evaluations on an interactive dialog dataset for Montreal cognitive assessments (MoCA) show that the adversarial training strategy can produce age-invariant embeddings and reduce diarization error rate (DER) by 4.33%. The approach also outperforms the conventional method even with less training data.
更多查看译文
关键词
speaker diarization,Montreal cognitive assessments,age-invariant speaker embedding,deep neural networks
AI 理解论文
溯源树
样例
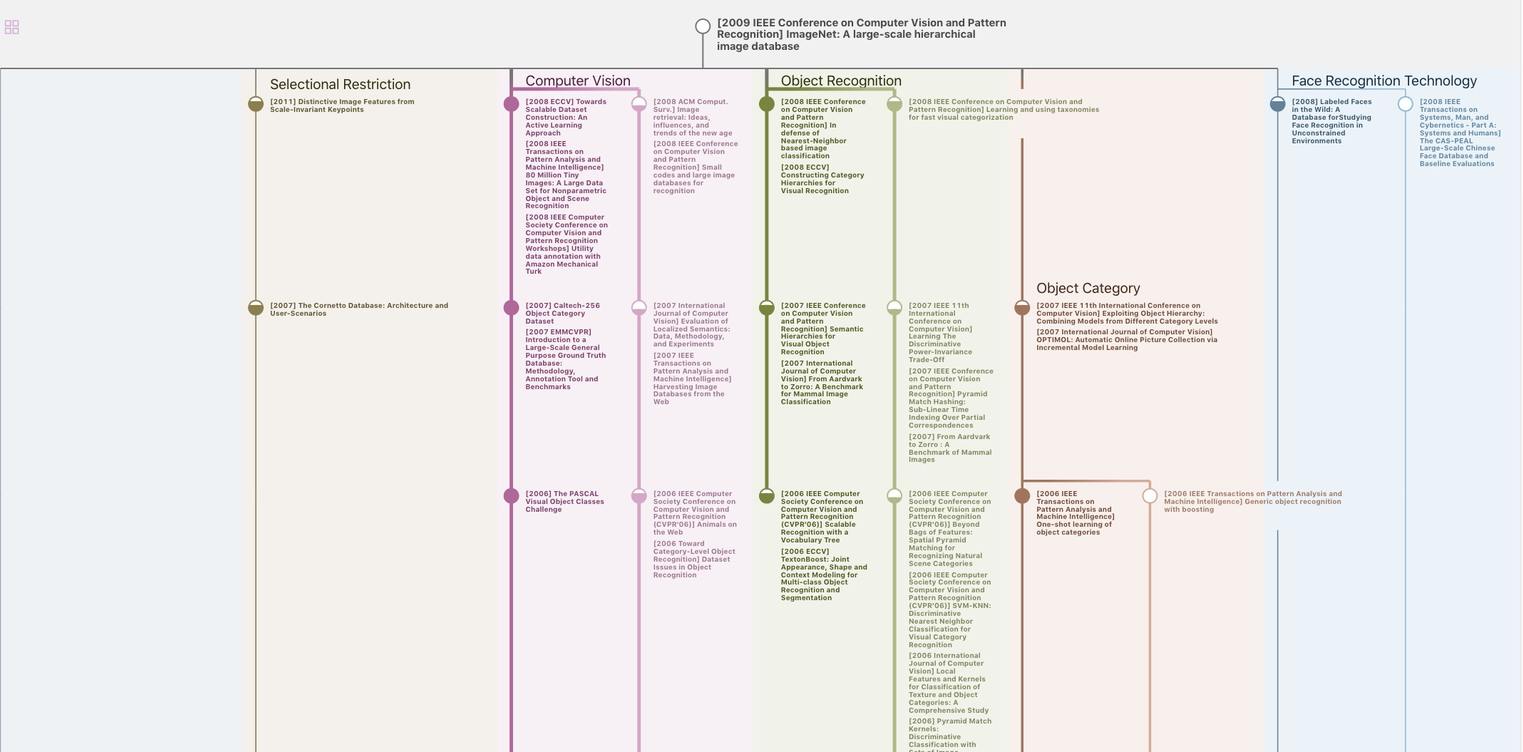
生成溯源树,研究论文发展脉络
Chat Paper
正在生成论文摘要