Design Of An Energy-Efficient Binarized Convolutional Neural Network Accelerator Using A Nonvolatile Field-Programmable Gate Array With Only-Once-Write Shifting
JAPANESE JOURNAL OF APPLIED PHYSICS(2021)
摘要
This paper presents an energy-efficient hardware accelerator for binarized convolutional neural networks (BCNNs). In this BCNN accelerator, a data-shift operation becomes dominant to effectively control input/weight-data streams under limited memory bandwidth. A magnetic-tunnel-junction (MTJ)-based nonvolatile field-programmable gate array (NV-FPGA), where the amount of stored-data updating is minimized in a configurable logic block, is a well-suited hardware platform for implementing such a BCNN accelerator. Owing to the nonvolatile storage capability of the NV-FPGA, not only power consumption in the data-shift operation but also standby power consumption in the idle function block is reduced without losing internal data. It is demonstrated under 45 nm complementary metal-oxide-semiconductor/MTJ process technologies that the energy consumption of the proposed BCNN accelerator is 50.7% lower than that of a BCNN accelerator using a conventional static-random-access-memory-based FPGA.
更多查看译文
关键词
FPGA, Binary Convolutional Neural Network, Nonvolatile, Lookup Table Circuit, Magnetic Tunnel Junction, Edge-AI
AI 理解论文
溯源树
样例
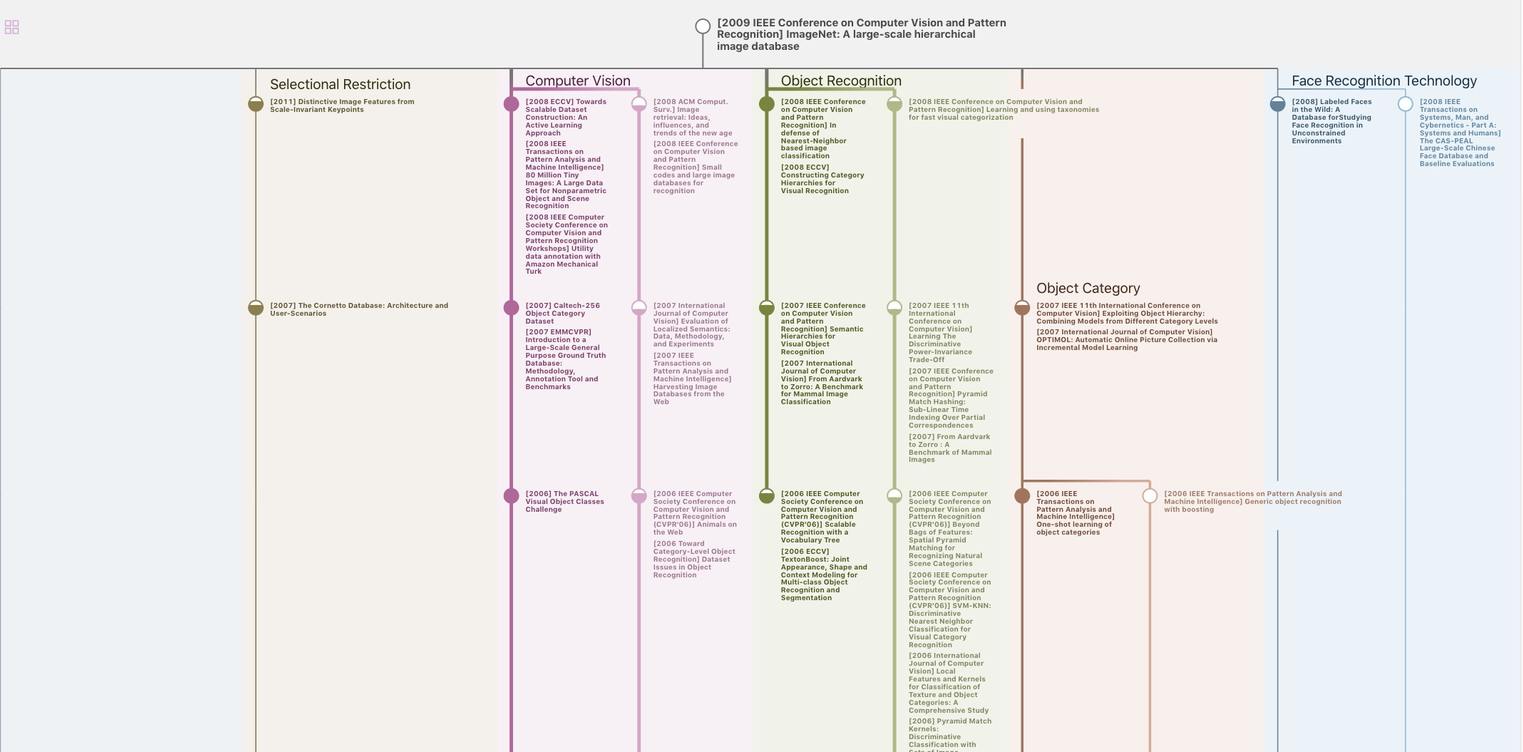
生成溯源树,研究论文发展脉络
Chat Paper
正在生成论文摘要