Combining Learning from Demonstration with Learning by Exploration to Facilitate Contact-Rich Tasks
2021 IEEE/RSJ INTERNATIONAL CONFERENCE ON INTELLIGENT ROBOTS AND SYSTEMS (IROS)(2021)
摘要
Collaborative robots are expected to work alongside humans and directly replace human workers in some cases, thus effectively responding to rapid changes in assembly lines. Current methods for programming contact-rich tasks, particularly in heavily constrained spaces, tend to be fairly inefficient. Therefore, faster and more intuitive approaches are urgently required for robot teaching. This study focuses on combining visual servoing-based learning from demonstration (LfD) and force-based learning by exploration (LbE) to enable the fast and intuitive programming of contact-rich tasks with minimal user efforts. Two learning approaches were developed and integrated into a framework, one relying on human-to-robot motion mapping (visual servoing approach) and the other relying on force-based reinforcement learning. The developed framework implements the noncontact demonstration teaching method based on the visual servoing approach and optimizes the demonstrated robot target positions according to the detected contact state. The developed framework is compared with two most commonly used baseline techniques, i.e., teach pendant-based teaching and hand-guiding teaching. Furthermore, the efficiency and reliability of the framework are validated via comparison experiments involving the teaching and execution of contact-rich tasks. The proposed framework shows the best performance in terms of the teaching time, execution success rate, risk of damage, and ease of use.
更多查看译文
关键词
visual servoing approach,demonstrated robot target positions,detected contact state,hand-guiding teaching,facilitate contact-rich tasks,collaborative robots,human workers,heavily constrained spaces,intuitive approaches,robot teaching,fast programming,intuitive programming,learning approaches,human-to-robot motion mapping,force-based reinforcement learning,noncontact demonstration teaching method
AI 理解论文
溯源树
样例
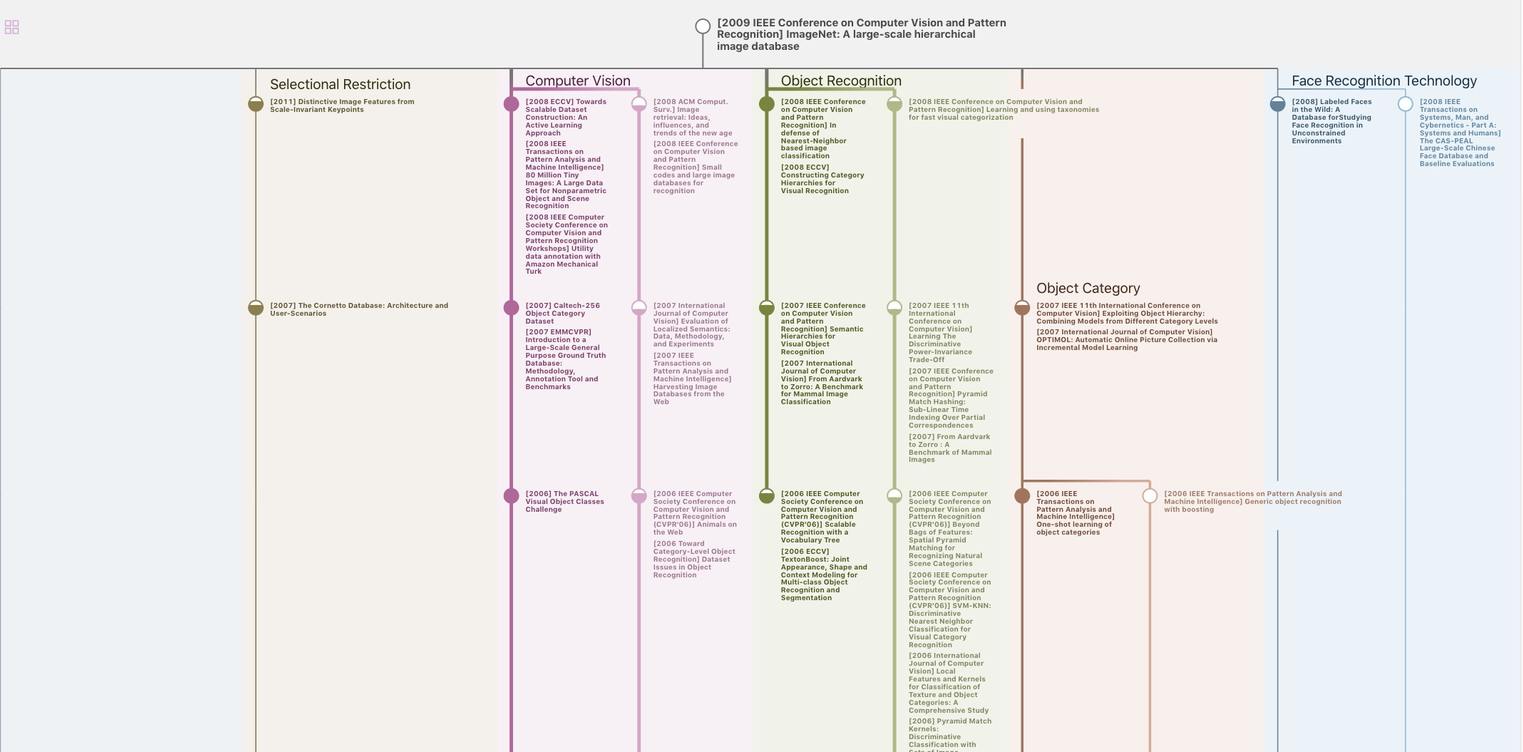
生成溯源树,研究论文发展脉络
Chat Paper
正在生成论文摘要