Minimum unbiased risk estimate based 2DPCA for color image denoising.
Neurocomputing(2021)
摘要
Low-rank approximation of matrices plays an important role in many application scenarios, including image denoising. This paper introduces a new low-rank approximation method named minimum unbiased risk estimate formulation of 2DPCA (MURE-2DPCA). MURE-2DPCA starts by considering the problem of estimating noise-free matrices from observations, and can exhibit robustness to outliers. In the case of a single data matrix constructed with Gaussian vectors, we find that the optimal dimension of the principal subspace can be determined automatically from the data itself. Based on MURE-2DPCA, a three-step algorithm is developed for color image denoising. Experiments demonstrate the ability and efficiency of our algorithm in achieving the denoising task.
更多查看译文
关键词
Stein’s unbiased risk estimate,Linear model,Gaussian matrix,Two-dimensional principal components analysis,Low-rank approximation,Color image denoising
AI 理解论文
溯源树
样例
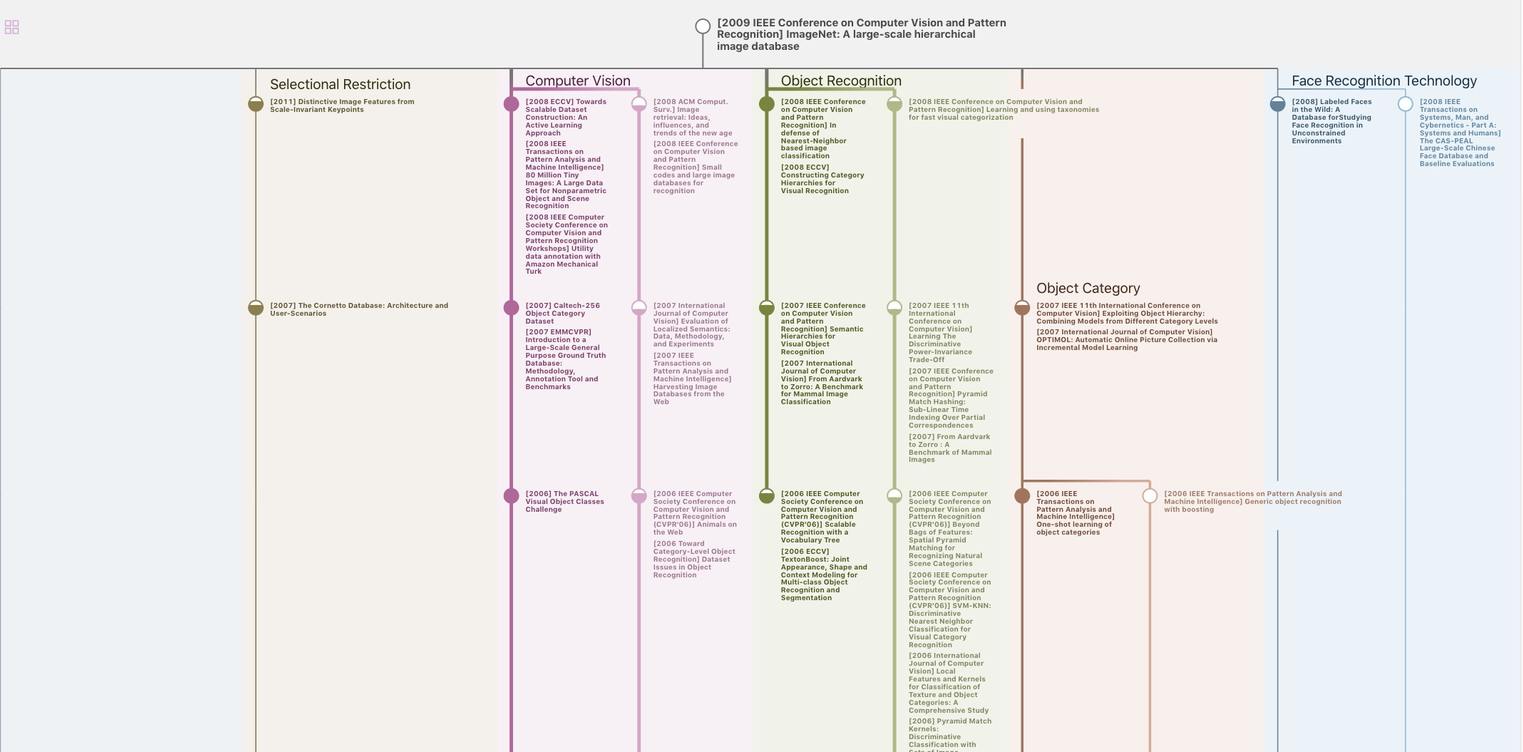
生成溯源树,研究论文发展脉络
Chat Paper
正在生成论文摘要