Adjusting for Autocorrelated Errors in Neural Networks for Time Series.
Annual Conference on Neural Information Processing Systems(2021)
摘要
An increasing body of research focuses on using neural networks to model time series. A common assumption in training neural networks via maximum likelihood estimation on time series is that the errors across time steps are uncorrelated. However, errors are actually autocorrelated in many cases due to the temporality of the data, which makes such maximum likelihood estimations inaccurate. In this paper, in order to adjust for autocorrelated errors, we propose to learn the autocorrelation coefficient jointly with the model parameters. In our experiments, we verify the effectiveness of our approach on time series forecasting. Results across a wide range of real-world datasets with various state-of-the-art models show that our method enhances performance in almost all cases. Based on these results, we suggest empirical critical values to determine the severity of autocorrelated errors. We also analyze several aspects of our method to demonstrate its advantages. Finally, other time series tasks are also considered to validate that our method is not restricted to only forecasting.
更多查看译文
关键词
autocorrelated errors,neural networks
AI 理解论文
溯源树
样例
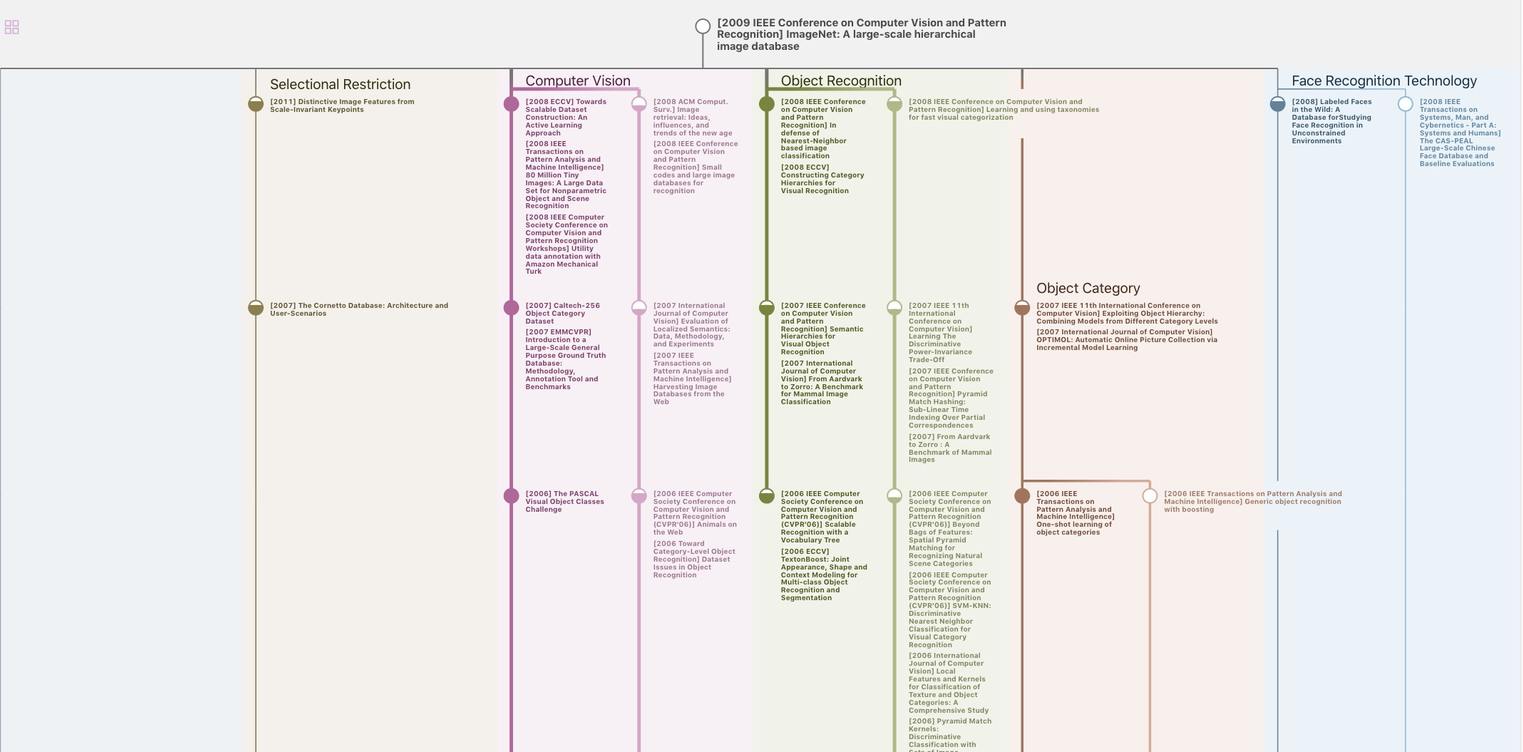
生成溯源树,研究论文发展脉络
Chat Paper
正在生成论文摘要