A Worst-Case Analysis of Trap-Assisted Tunneling Leakage in DRAM Using a Machine Learning Approach
IEEE Electron Device Letters(2021)
摘要
The variability in trap-assisted tunneling leakage that is enhanced by random discrete dopants (RDD) causes refresh failure in scaled 6F
2
dynamic random-access memory (DRAM) cells. Thus, the worst-case leakage analysis is in high demand, but it requires significant computational cost. To overcome this issue, we performed 200 leakage variability simulations with RDD and a single trap to train a multi-layer neural-network (NN) model. Moreover, we propose a simulation flow using the NN model to find the worst RDD configuration among 5,000 candidates. We demonstrate the worst-case leakage can be found with 96.7% probability using only 5.5% computational cost compared to a full 3D TCAD statistical simulation approach.
更多查看译文
关键词
DRAM,leakage,machine learning,trap-assisted tunneling,worst-case analysis
AI 理解论文
溯源树
样例
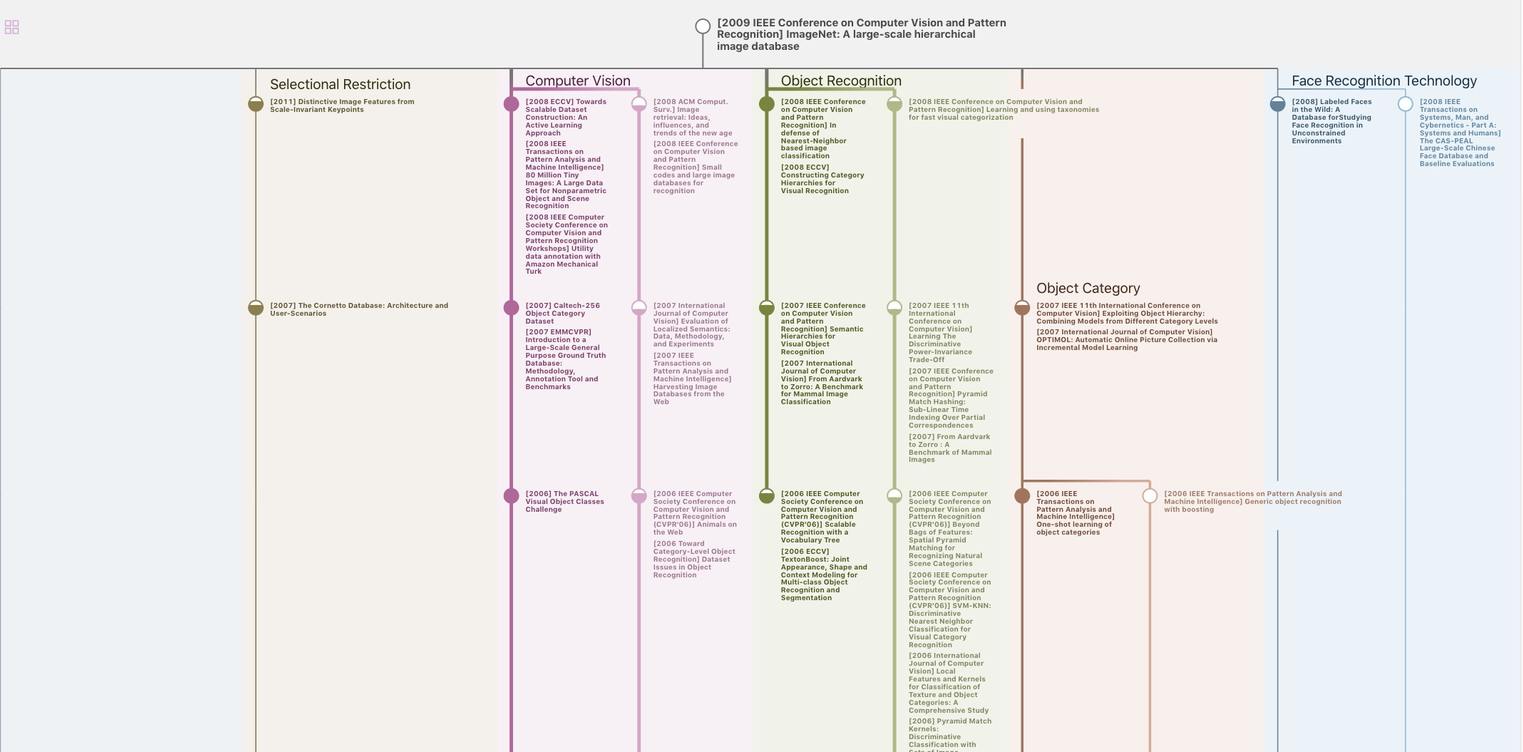
生成溯源树,研究论文发展脉络
Chat Paper
正在生成论文摘要