Memory-Efficient Semi-Supervised Continual Learning: The World is its Own Replay Buffer
2021 INTERNATIONAL JOINT CONFERENCE ON NEURAL NETWORKS (IJCNN)(2021)
摘要
Rehearsal is a critical component for class-incremental continual learning, yet it requires a substantial memory budget. Our work investigates whether we can significantly reduce this memory budget by leveraging unlabeled data from an agent's environment in a realistic and challenging continual learning paradigm. Specifically, we explore and formalize a novel semi-supervised continual learning (SSCL) setting, where labeled data is scarce yet non-i.i.d. unlabeled data from the agent's environment is plentiful. Importantly, data distributions in the SSCL setting are realistic and therefore reflect object class correlations between, and among, the labeled and unlabeled data distributions. We show that a strategy built on pseudo-labeling, consistency regularization, Out-of-Distribution (OoD) detection, and knowledge distillation reduces forgetting in this setting. Our approach, DistillMatch, increases performance over the state-of-the-art by no less than 8.7% average task accuracy and up to 54.5% average task accuracy in SSCL CIFAR-100 experiments. Moreover, we demonstrate that DistillMatch can save up to 0.23 stored images per processed unlabeled image compared to the next best method which only saves 0.08. Our results suggest that focusing on realistic correlated distributions is a significantly new perspective, which accentuates the importance of leveraging the world's structure as a continual learning strategy. Our code is available at https://github.com/GT-RIPL/DistillMatch-SSCL
更多查看译文
关键词
semisupervised continual learning,replay buffer,class-incremental continual learning,data distributions,SSCL,object class correlations,pseudolabeling,Out-of-Distribution detection,knowledge distillation,DistillMatch,rehearsal,computer vision
AI 理解论文
溯源树
样例
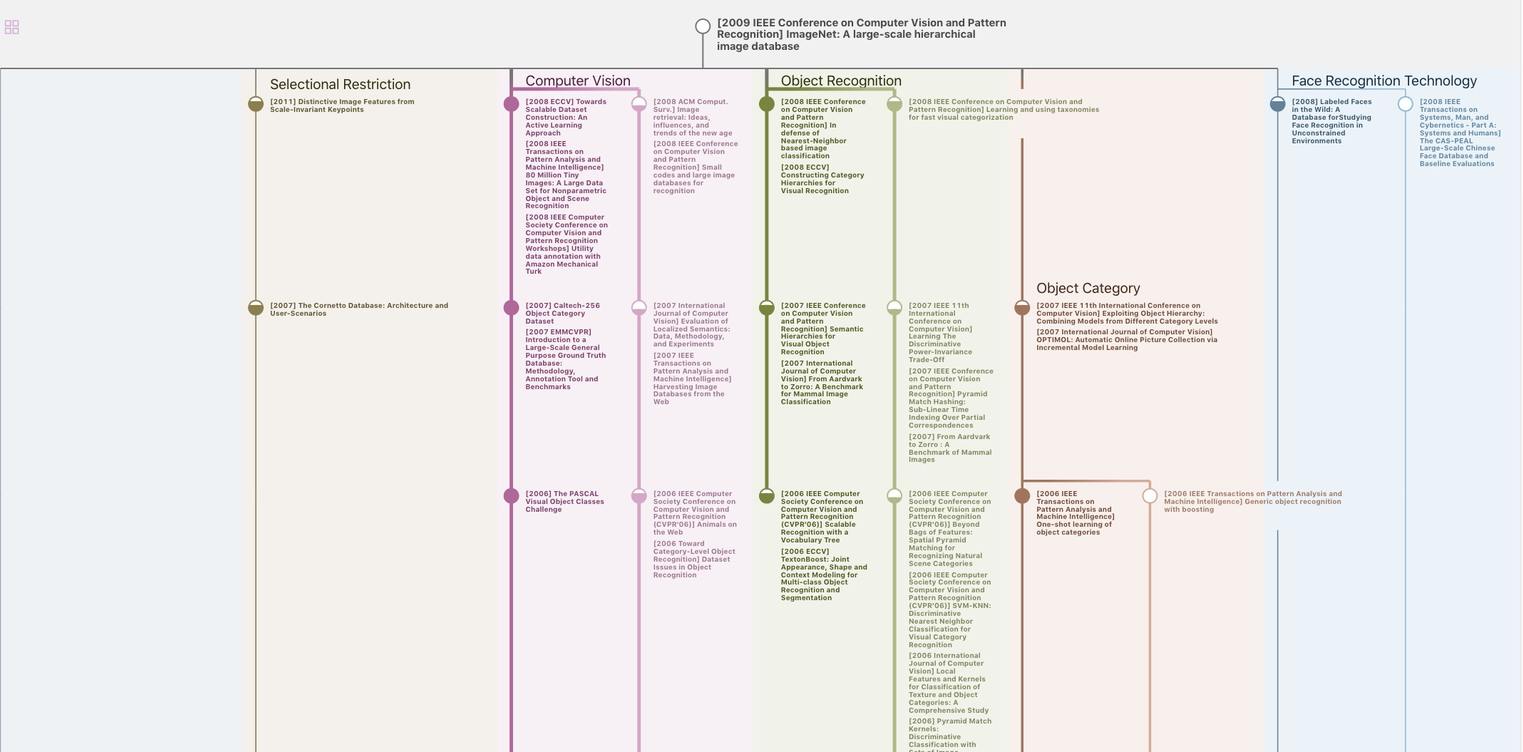
生成溯源树,研究论文发展脉络
Chat Paper
正在生成论文摘要