SimGAN: Hybrid Simulator Identification for Domain Adaptation via Adversarial Reinforcement Learning
2021 IEEE INTERNATIONAL CONFERENCE ON ROBOTICS AND AUTOMATION (ICRA 2021)(2021)
摘要
As learning-based approaches progress towards automating robot controllers design, transferring learned policies to new domains with different dynamics (e.g. sim-to-real transfer) still demands manual effort. This paper introduces SimGAN, a framework to tackle domain adaptation by identifying a hybrid physics simulator to match the simulated trajectories to the ones from the target domain, using a learned discriminative loss to address the limitations associated with manual loss design. Our hybrid simulator combines neural networks and traditional physics simulation to balance expressiveness and generalizability, and alleviates the need for a carefully selected parameter set in System ID. Once the hybrid simulator is identified via adversarial reinforcement learning, it can be used to refine policies for the target domain, without the need to interleave data collection and policy refinement. We show that our approach outperforms multiple strong baselines on six robotic locomotion tasks for domain adaptation.
更多查看译文
关键词
domain adaptation,hybrid simulator identification,adversarial reinforcement learning
AI 理解论文
溯源树
样例
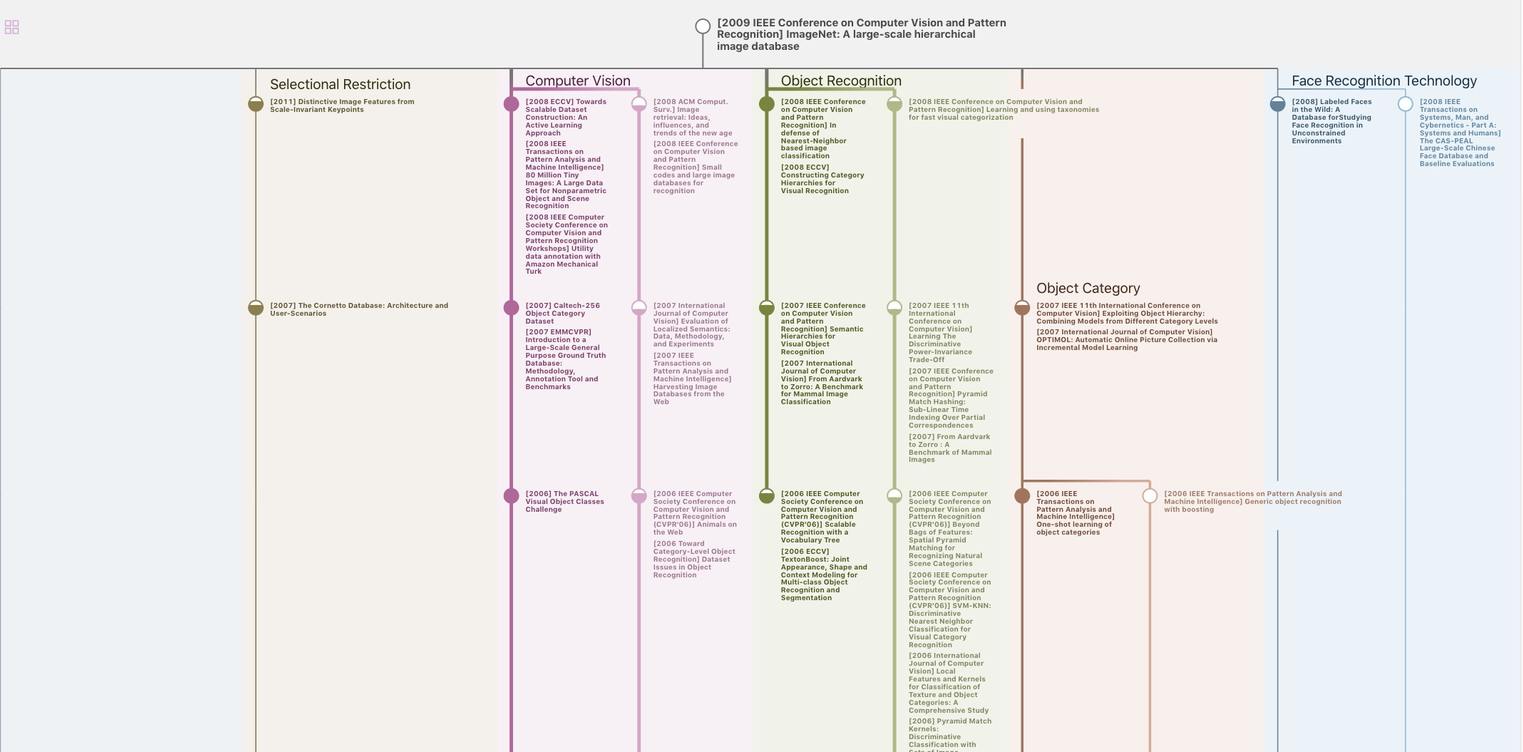
生成溯源树,研究论文发展脉络
Chat Paper
正在生成论文摘要