Understanding Strengths And Weaknesses Of Complementary Sensor Modalities In Early Fusion For Object Detection
2020 IEEE INTELLIGENT VEHICLES SYMPOSIUM (IV)(2020)
摘要
In object detection for autonomous driving and robotic applications, conventional RGB cameras often fail to sense objects under extreme illumination conditions and on texture-less surfaces, while LIDAR sensors often fail to sense small or thin objects located far from the sensor. For these reasons, an intuitive and obvious choice for perception system designers is to install multiple sensors of different modalities to increase (in theory) the detection robustness. In this paper we focus on the analysis of an object detector that performs early fusion of RGB images and LIDAR 3D points. Our goal is to go beyond the intuition of simply adding more sensor modalities to improve performance, and instead analyze, quantify, and understand the performance differences, strengths and weaknesses of the object detector under three different modalities: 1) RGB-only, 2) LIDAR-only, and 3) Early fusion (RGB and LIDAR), and under two key scene variables: 1) Distance of objects from the sensor (density), and 2) Illumination (Darkness). We also propose methodologies to generate 2D weak semantic training masks, and a methodology to evaluate the object detection performance separately at different distance ranges, which provides a more reliable detection performance measure and correlates well with object LIDAR point density.
更多查看译文
关键词
early fusion,RGB cameras,2D weak semantic training masks,LIDAR 3D points,RGB images,detection robustness,multiple sensors,perception system designers,LIDAR sensors,texture-less surfaces,extreme illumination conditions,robotic applications,autonomous driving,complementary sensor modalities,object LIDAR point density,reliable detection performance,distance ranges,object detection performance
AI 理解论文
溯源树
样例
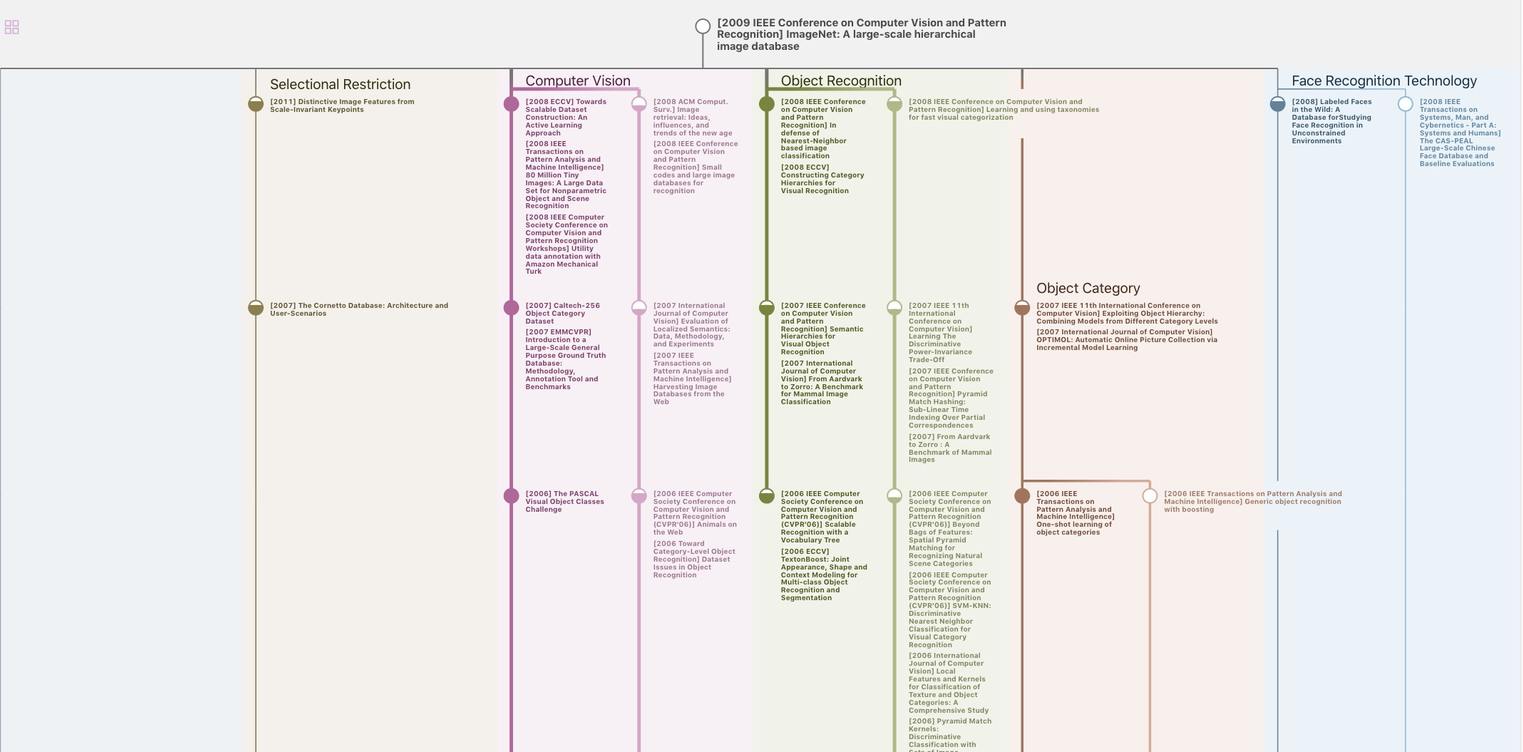
生成溯源树,研究论文发展脉络
Chat Paper
正在生成论文摘要