Image Compression With Laplacian Guided Scale Space Inpainting
2020 IEEE INTERNATIONAL CONFERENCE ON IMAGE PROCESSING (ICIP)(2020)
摘要
We present an image compression algorithm that preserves high-frequency details and information of rare occurrences. Our approach can be thought of as image inpainting in the frequency scale space. Given an image, we construct a Laplacian image pyramid, and store only the finest and coarsest levels, thereby removing the middle-frequency of the image. Using a network backbone borrowed from an image super-resolution algorithm, we train our network to hallucinate the missing middle-level Laplacian image. We introduce a novel training paradigm where we train our algorithm using only a face dataset where the faces are aligned and scaled correctly. We demonstrate that image compression learned on this restricted dataset leads to better GAN network [1] convergence and generalization to completely different image domains. We also show that Lapacian inpainting could be simplified further with a few selective pixels as seeds.
更多查看译文
关键词
Image Inpainting, Super-Resolution
AI 理解论文
溯源树
样例
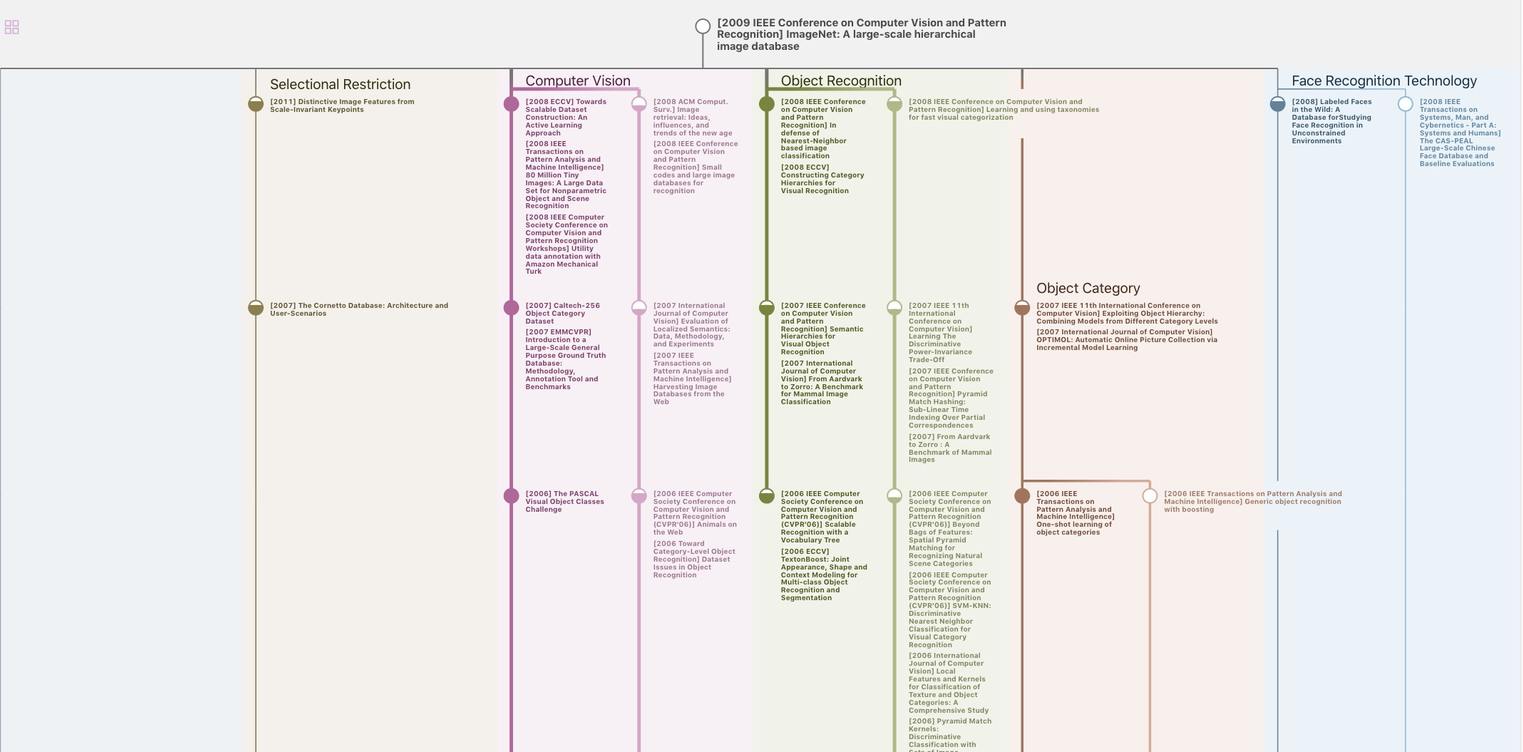
生成溯源树,研究论文发展脉络
Chat Paper
正在生成论文摘要