Learning Object Placement by Inpainting for Compositional Data Augmentation
European Conference on Computer Vision(2020)
摘要
We study the problem of common sense placement of visual objects in an image. This involves multiple aspects of visual recognition: the instance segmentation of the scene, 3D layout, and common knowledge of how objects are placed and where objects are moving in the 3D scene. This seemingly simple task is difficult for current learning-based approaches because of the lack of labeled training pair of foreground objects paired with cleaned background scenes. We propose a self-learning framework that automatically generates the necessary training data without any manual labeling by detecting, cutting, and inpainting objects from an image. We propose a PlaceNet that predicts a diverse distribution of common sense locations when given a foreground object and a background scene. We show one practical use of our object placement network for augmenting training datasets by recomposition of object-scene with a key property of contextual relationship preservation. We demonstrate improvement of object detection and instance segmentation performance on both Cityscape[4] and KITTI[9] datasets. We also show that the learned representation of our PlaceNet displays strong discriminative power in image retrieval and classification.
更多查看译文
关键词
Object placement,Inpainting,Data augmentation
AI 理解论文
溯源树
样例
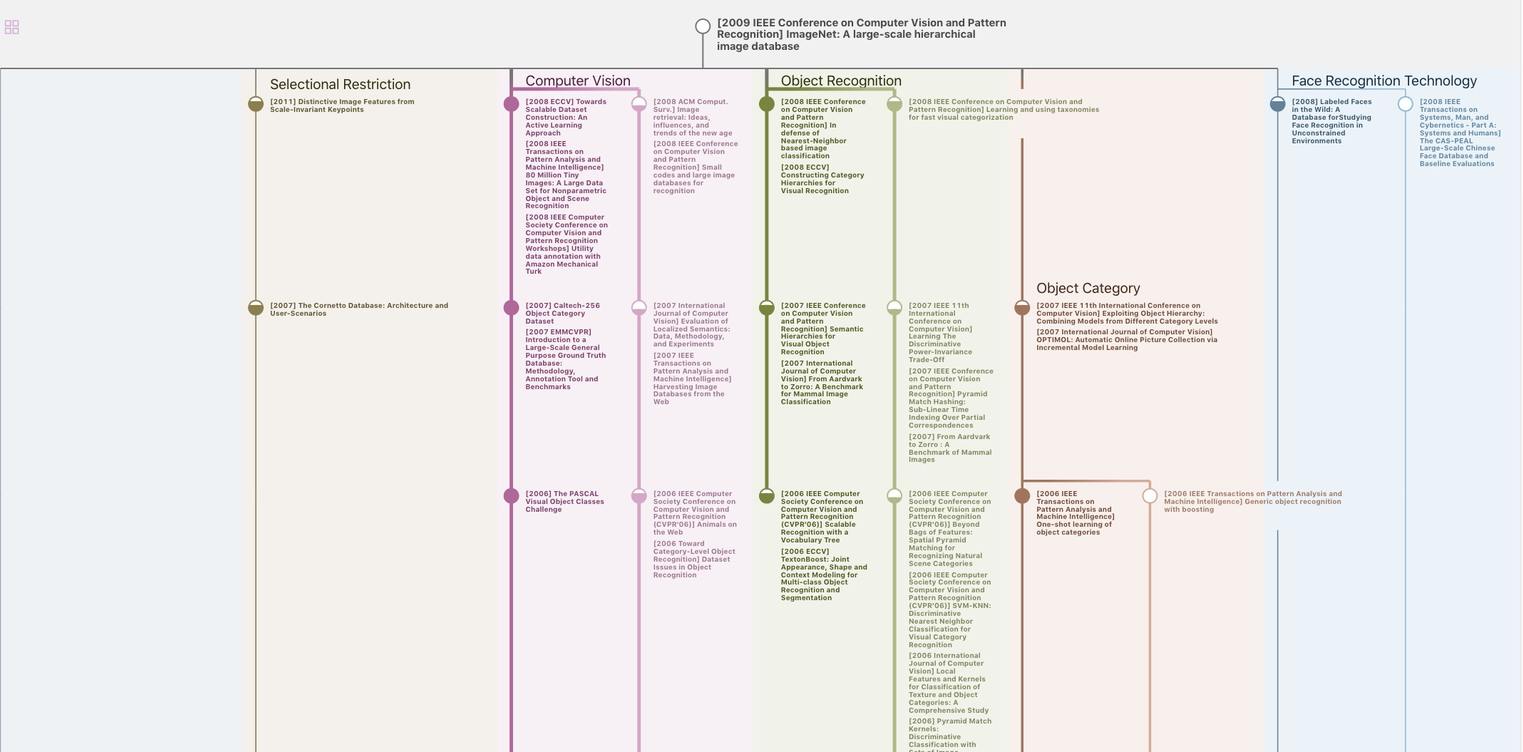
生成溯源树,研究论文发展脉络
Chat Paper
正在生成论文摘要