Mining explainable local and global subgraph patterns with surprising densities
DATA MINING AND KNOWLEDGE DISCOVERY(2020)
摘要
The connectivity structure of graphs is typically related to the attributes of the vertices. In social networks for example, the probability of a friendship between any pair of people depends on a range of attributes, such as their age, residence location, workplace, and hobbies. The high-level structure of a graph can thus possibly be described well by means of patterns of the form ‘the subgroup of all individuals with certain properties X are often (or rarely) friends with individuals in another subgroup defined by properties Y’, ideally relative to their expected connectivity. Such rules present potentially actionable and generalizable insight into the graph. Prior work has already considered the search for dense subgraphs (‘communities’) with homogeneous attributes. The first contribution in this paper is to generalize this type of pattern to densities between a pair of subgroups , as well as between all pairs from a set of subgroups that partition the vertices . Second, we develop a novel information-theoretic approach for quantifying the subjective interestingness of such patterns, by contrasting them with prior information an analyst may have about the graph’s connectivity. We demonstrate empirically that in the special case of dense subgraphs, this approach yields results that are superior to the state-of-the-art. Finally, we propose algorithms for efficiently finding interesting patterns of these different types.
更多查看译文
关键词
Graph mining, Graph summarization, Graph clustering, Community detection, Subjective interestingness, Subgroup discovery
AI 理解论文
溯源树
样例
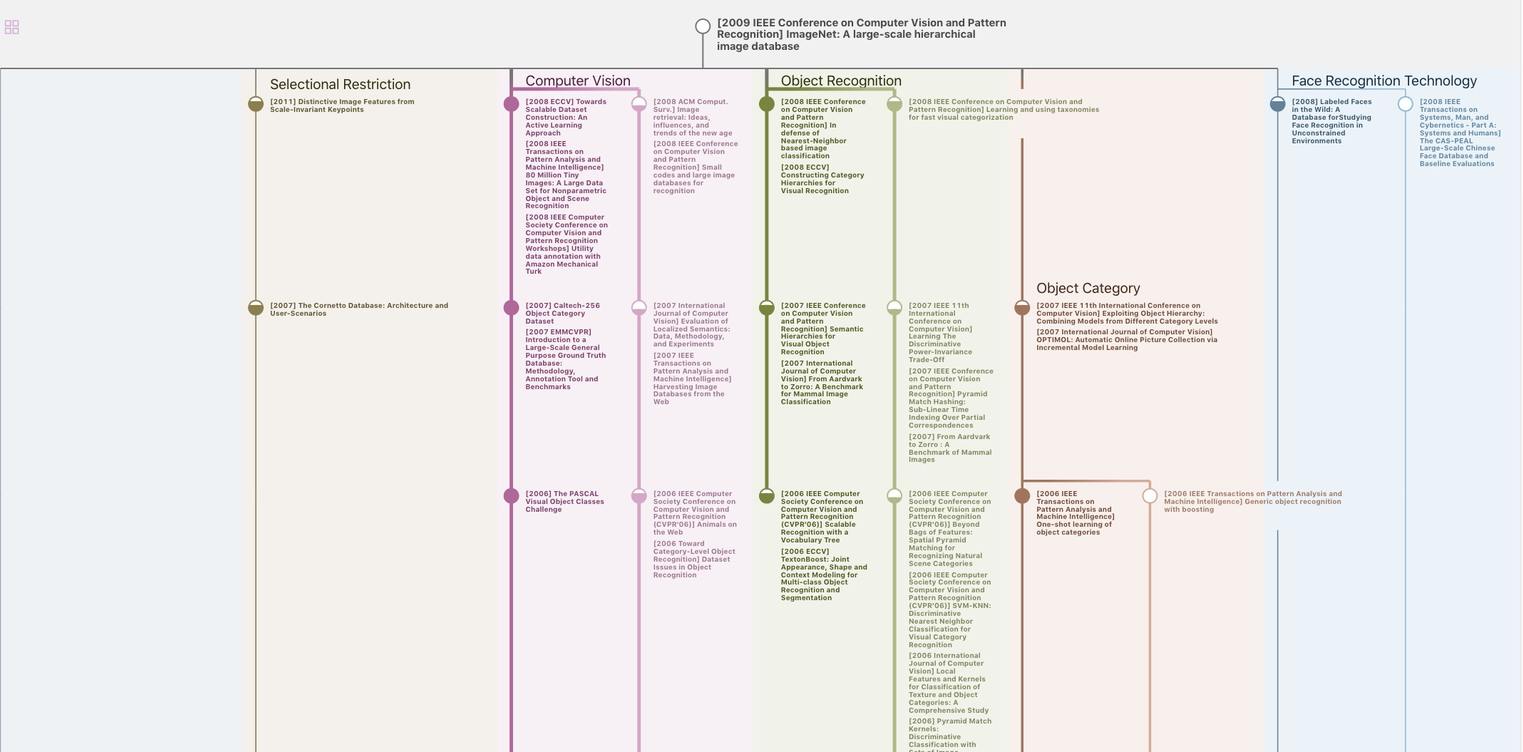
生成溯源树,研究论文发展脉络
Chat Paper
正在生成论文摘要