Learning Quintuplet Loss for Large-Scale Visual Geolocalization
IEEE MultiMedia(2020)
摘要
With the maturity of artificial intelligence technology, large-scale visual geolocalization (LSVGL) is increasingly important in urban computing, where the task is to accurately and efficiently recognize the geolocation of a given query image. The main challenge of LSVGL faced by many experiments due to the appearance of real-word places may differ in various ways while perspective deviation almost inevitably exists between training images and query images because of the arbitrary perspective. To cope with this situation, in this article, we in-depth analyze the limitation of triplet loss, which is the most commonly used metric learning loss in state-of-the-art LSVGL framework and propose a new quintuplet loss by embedding all the potential positive samples to the primitive triplet loss. Extensive experiments are conducted to verify the effectiveness of the proposed approach and the results demonstrate that our new loss can enhance various LSVGL methods.
更多查看译文
关键词
visual geo-localization,triplet loss,quintuplet loss,deep neural network
AI 理解论文
溯源树
样例
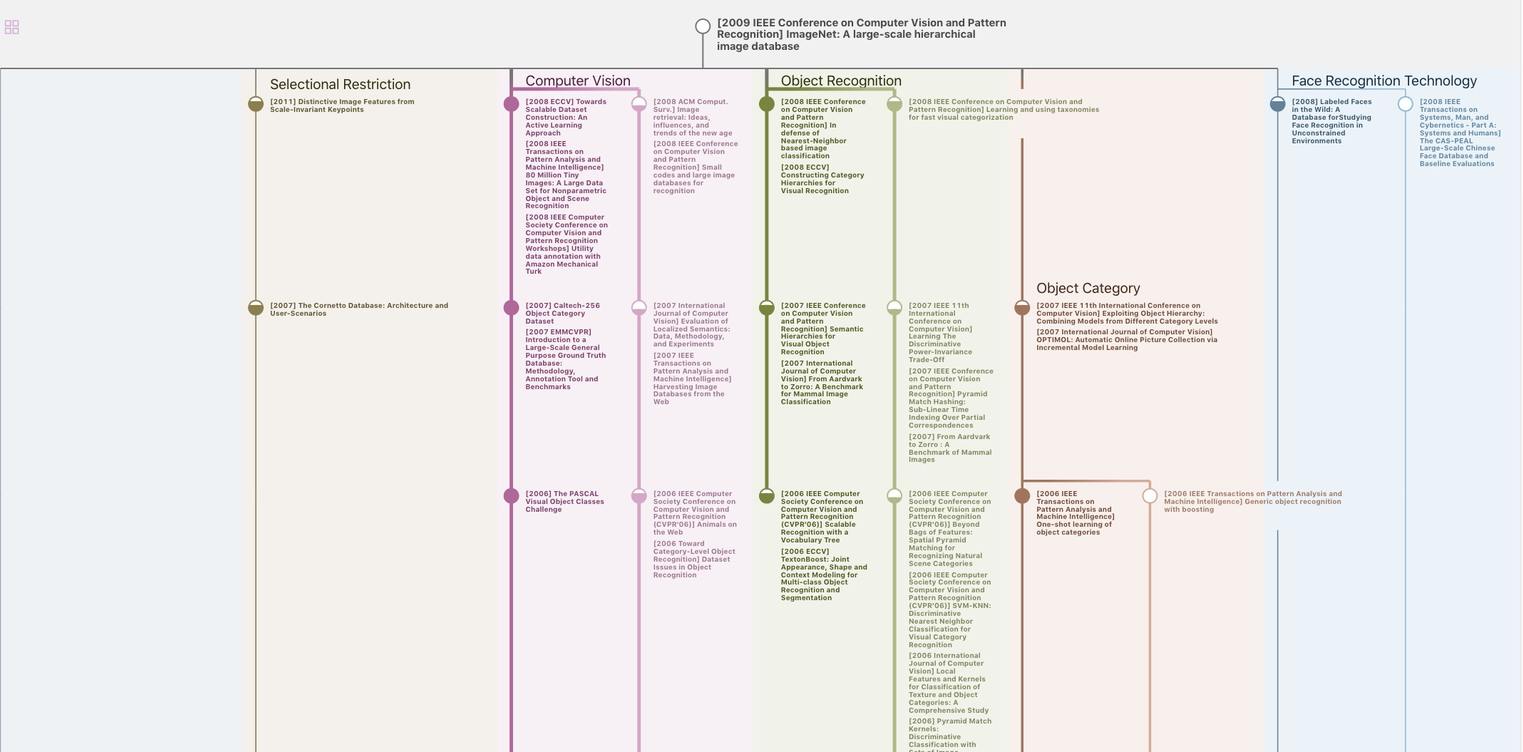
生成溯源树,研究论文发展脉络
Chat Paper
正在生成论文摘要