Few-Shot Ensemble Learning for Video Classification with SlowFast Memory Networks
MM '20: The 28th ACM International Conference on Multimedia Seattle WA USA October, 2020(2020)
摘要
In the era of big data, few-shot learning has recently received much attention in multimedia analysis and computer vision due to its appealing ability of learning from scarce labeled data. However, it has been largely underdeveloped in the video domain, which is even more challenging due to the huge spatial-temporal variability of video data. In this paper, we address few-shot video classification by learning an ensemble of SlowFast networks augmented with memory units. Specifically, we introduce a family of few-shot learners based on SlowFast networks which are used to extract informative features at multiple rates, and we incorporate a memory unit into each network to enable encoding and retrieving crucial information instantly. Furthermore, we propose a choice controller network to leverage the diversity of few-shot learners by learning to adaptively assign a confidence score to each SlowFast memory network, leading to a strong classifier for enhanced prediction. Experimental results on two widely-adopted video datasets demonstrate the effectiveness of the proposed method, as well as its superior performance over the state-of-the-art approaches.
更多查看译文
关键词
Ensemble Learning, Few-Shot Learning, Video Classification, Memory Network
AI 理解论文
溯源树
样例
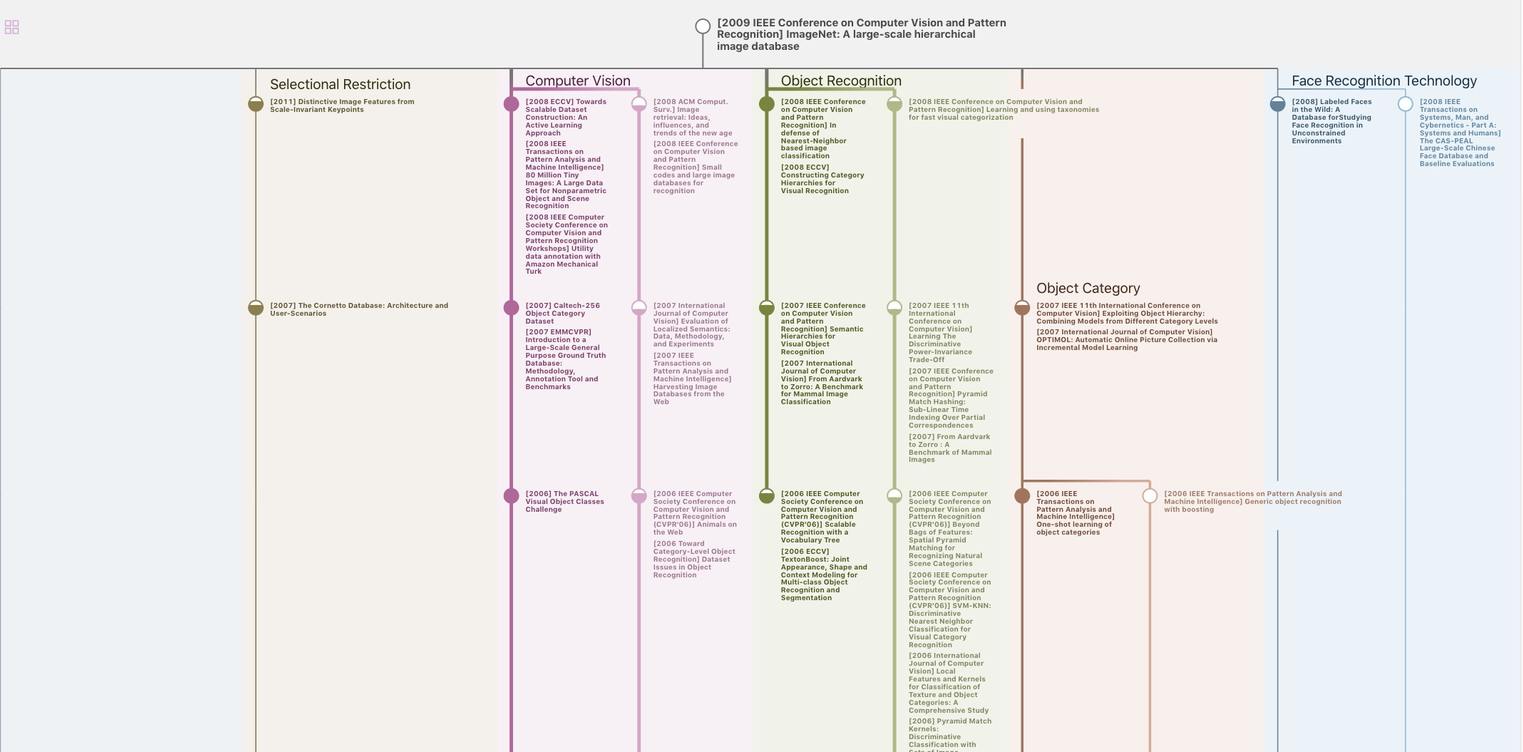
生成溯源树,研究论文发展脉络
Chat Paper
正在生成论文摘要