MEGATRON-CNTRL: Controllable Story Generation with External Knowledge Using Large-Scale Language Models
Conference on Empirical Methods in Natural Language Processing(2020)
摘要
Existing pre-trained large language models have shown unparalleled generative capabilities. However, they are not controllable. In this paper, we propose MEGATRON-CNTRL, a novel framework that uses large-scale language models and adds control to text generation by incorporating an external knowledge base. Our framework consists of a keyword predictor, a knowledge retriever, a contextual knowledge ranker, and a conditional text generator. As we do not have access to ground-truth supervision for the knowledge ranker, we make use of weak supervision from sentence embedding. The empirical results show that our model generates more fluent, consistent, and coherent stories with less repetition and higher diversity compared to prior work on the ROC story dataset. We showcase the controllability of our model by replacing the keywords used to generate stories and re-running the generation process. Human evaluation results show that 77.5% of these stories are successfully controlled by the new keywords. Furthermore, by scaling our model from 124 million to 8.3 billion parameters we demonstrate that larger models improve both the quality of generation (from 74.5% to 93.0% for consistency) and controllability (from 77.5% to 91.5%).
更多查看译文
关键词
controllable story generation,language models,external knowledge
AI 理解论文
溯源树
样例
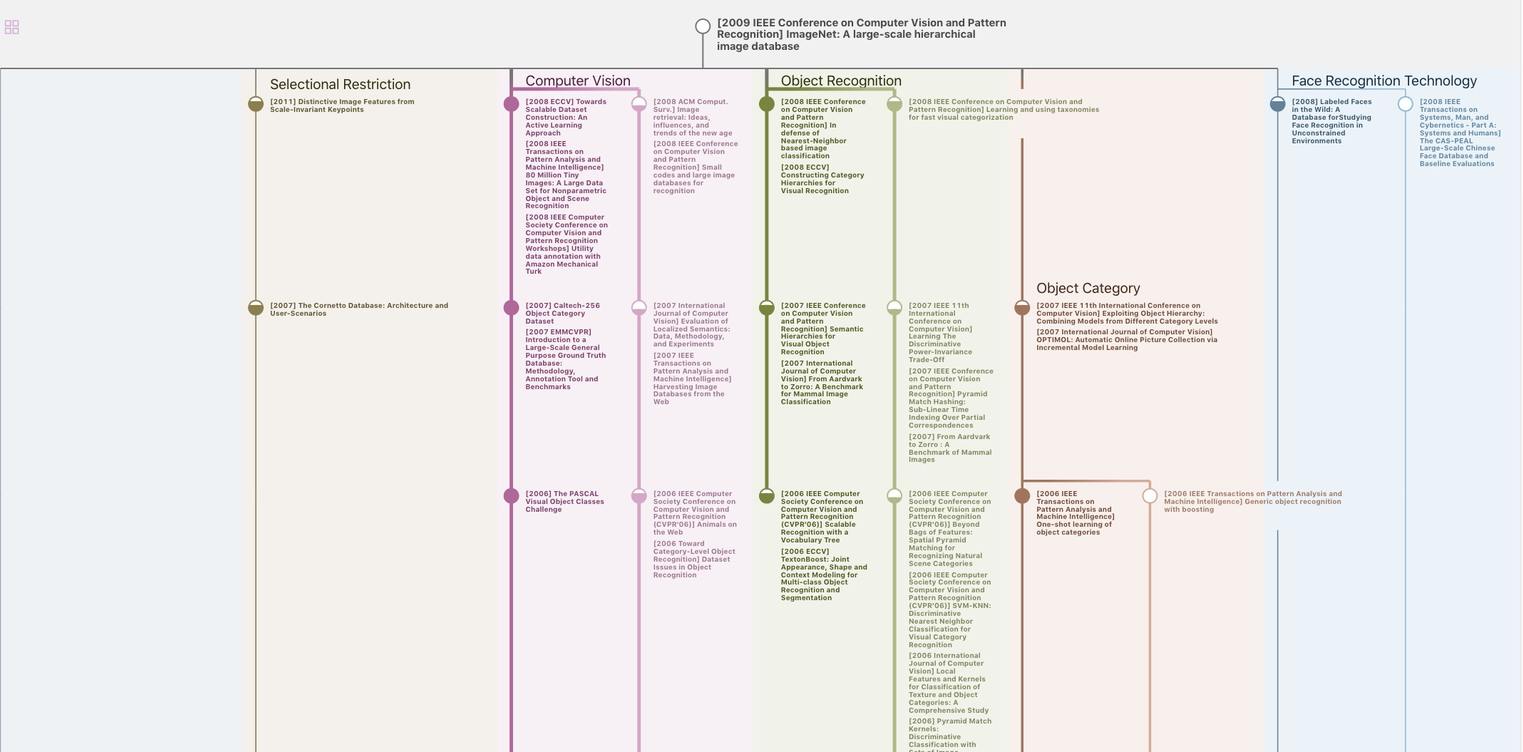
生成溯源树,研究论文发展脉络
Chat Paper
正在生成论文摘要