Calibrationless parallel imaging reconstruction for multislice MR data using low-rank tensor completion
MAGNETIC RESONANCE IN MEDICINE(2021)
摘要
Purpose To provide joint calibrationless parallel imaging reconstruction of highly accelerated multislice 2D MR k-space data. Methods Adjacent image slices in multislice MR data have similar coil sensitivity maps, spatial support, and image content. Such similarities can be utilized to improve image quality by reconstructing multiple slices jointly with low-rank tensor completion. Specifically, the multichannel k-space data from multiple slices are constructed into a block-wise Hankel tensor and iteratively updated by promoting tensor low-rankness through higher-order SVD. This multislice block-wise Hankel tensor completion was implemented for 2D spiral and Cartesian k-space undersampling where sampling patterns vary between adjacent slices. The approach was evaluated with human brain MR data and compared to the traditional single-slice simultaneous autocalibrating and k-space estimation reconstruction. Results The proposed multislice block-wise Hankel tensor completion approach robustly reconstructed highly undersampled multislice 2D spiral and Cartesian data. It produced substantially lower level of artifacts compared to the traditional single-slice simultaneous autocalibrating and k-space estimation reconstruction. Quantitative evaluation using error maps and root mean square error demonstrated its significantly improved performance in terms of residual artifacts and root mean square error. Conclusion Our proposed multislice block-wise Hankel tensor completion method exploits the similar coil sensitivity and image content within multislice MR data through a tensor completion framework. It offers a new and effective approach to acquire and reconstruct highly undersampled multislice MR data in a calibrationless manner.
更多查看译文
关键词
Hankel tensor completion, low-rank, multislice, parallel imaging
AI 理解论文
溯源树
样例
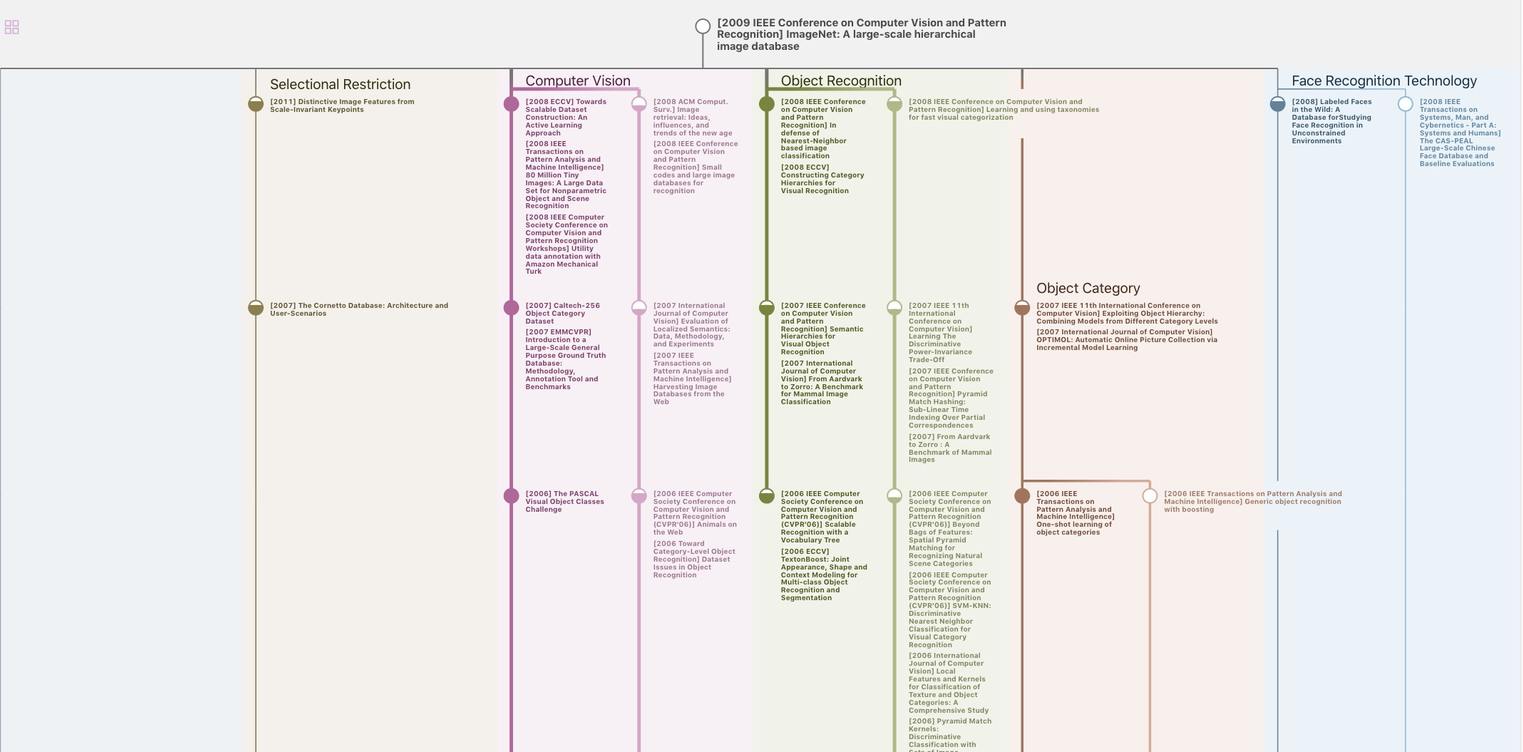
生成溯源树,研究论文发展脉络
Chat Paper
正在生成论文摘要