Bandits Under The Influence (Extended Version)
arxiv(2020)
摘要
Recommender systems should adapt to user interests as the latter evolve. A prevalent cause for the evolution of user interests is the influence of their social circle. In general, when the interests are not known, online algorithms that explore the recommendation space while also exploiting observed preferences are preferable. We present online recommendation algorithms rooted in the linear multi-armed bandit literature. Our bandit algorithms are tailored precisely to recommendation scenarios where user interests evolve under social influence. In particular, we show that our adaptations of the classic LinREL and Thompson Sampling algorithms maintain the same asymptotic regret bounds as in the non-social case. We validate our approach experimentally using both synthetic and real datasets.
更多查看译文
关键词
bandits,extended version,influence
AI 理解论文
溯源树
样例
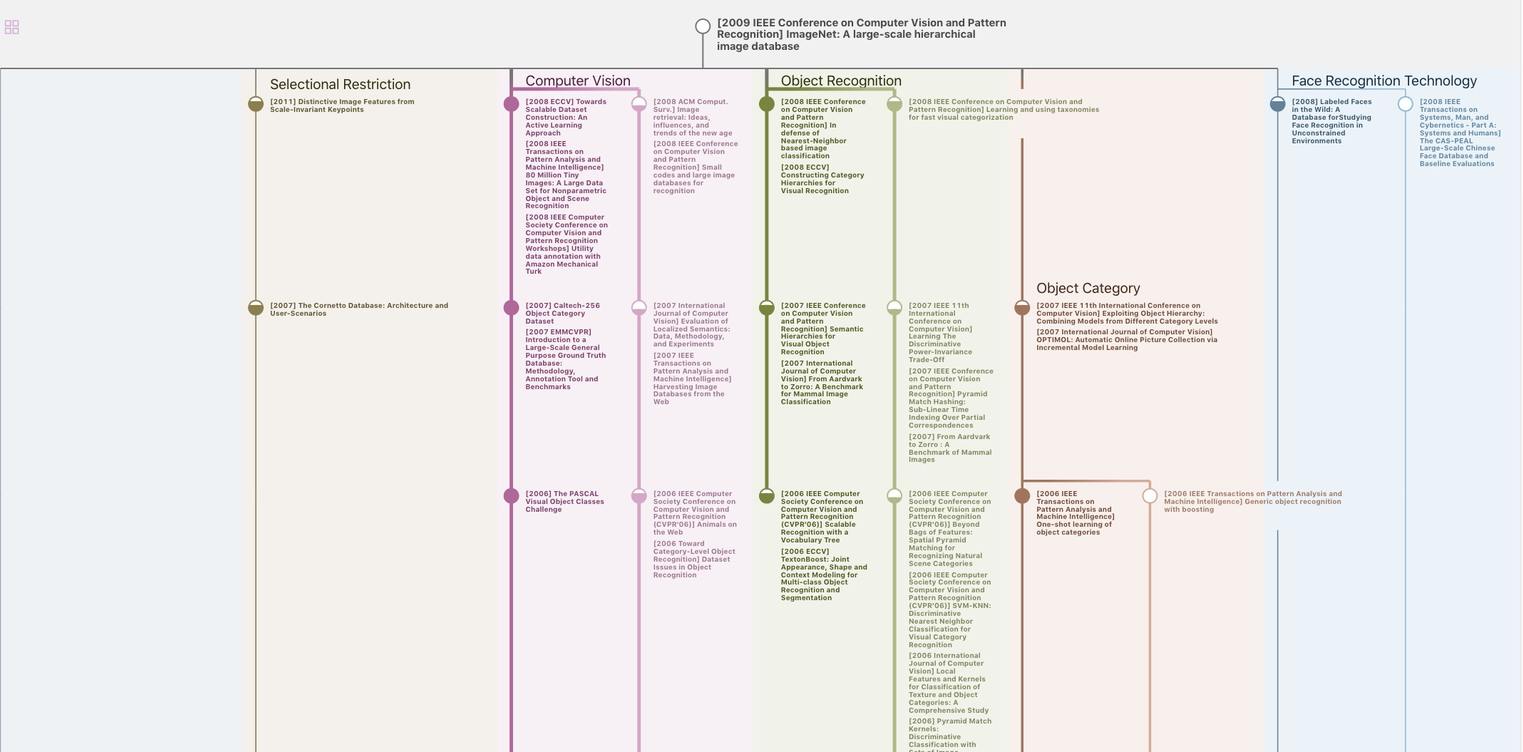
生成溯源树,研究论文发展脉络
Chat Paper
正在生成论文摘要