COCO-Search18: A Dataset for Predicting Goal-directed Attention Control
biorxiv(2020)
摘要
Attention control is a basic behavioral process that has been studied for decades. The currently best models of attention control are deep networks trained on free-viewing behavior to predict bottom-up attention control—saliency. We introduce COCO-Search18, the first dataset of laboratory-quality large enough to train deep-network models. We collected eye-movement behavior from 10 people searching for each of 18 target-object categories in 6202 natural-scene images, yielding ∼300,000 search fixations. We thoroughly characterize COCO-Search18, and benchmark it using three machine-learning methods: a ResNet50 object detector, a ResNet50 trained on fixation-density maps, and an inverse-reinforcement-learning model trained on behavioral search scanpaths. Models were also trained/tested on images transformed to approximate a foveated retina, a fundamental biological constraint. These models, each having a different reliance on behavioral training, collectively comprise the new state-of-the-art in predicting goal-directed search fixations. Our expectation is that future work using COCO-Search18 will far surpass these initial efforts, finding applications in domains ranging from human-computer interactive systems that can anticipate a person’s intent and render assistance to the potentially early identification of attention-related clinical disorders (ADHD, PTSD, phobia) based on deviation from neurotypical fixation behavior.
更多查看译文
关键词
Goal Dataset,Attention Dataset,Fixation Dataset,Gaze Dataset,Visual Search,Inverse-Reinforcement Learning
AI 理解论文
溯源树
样例
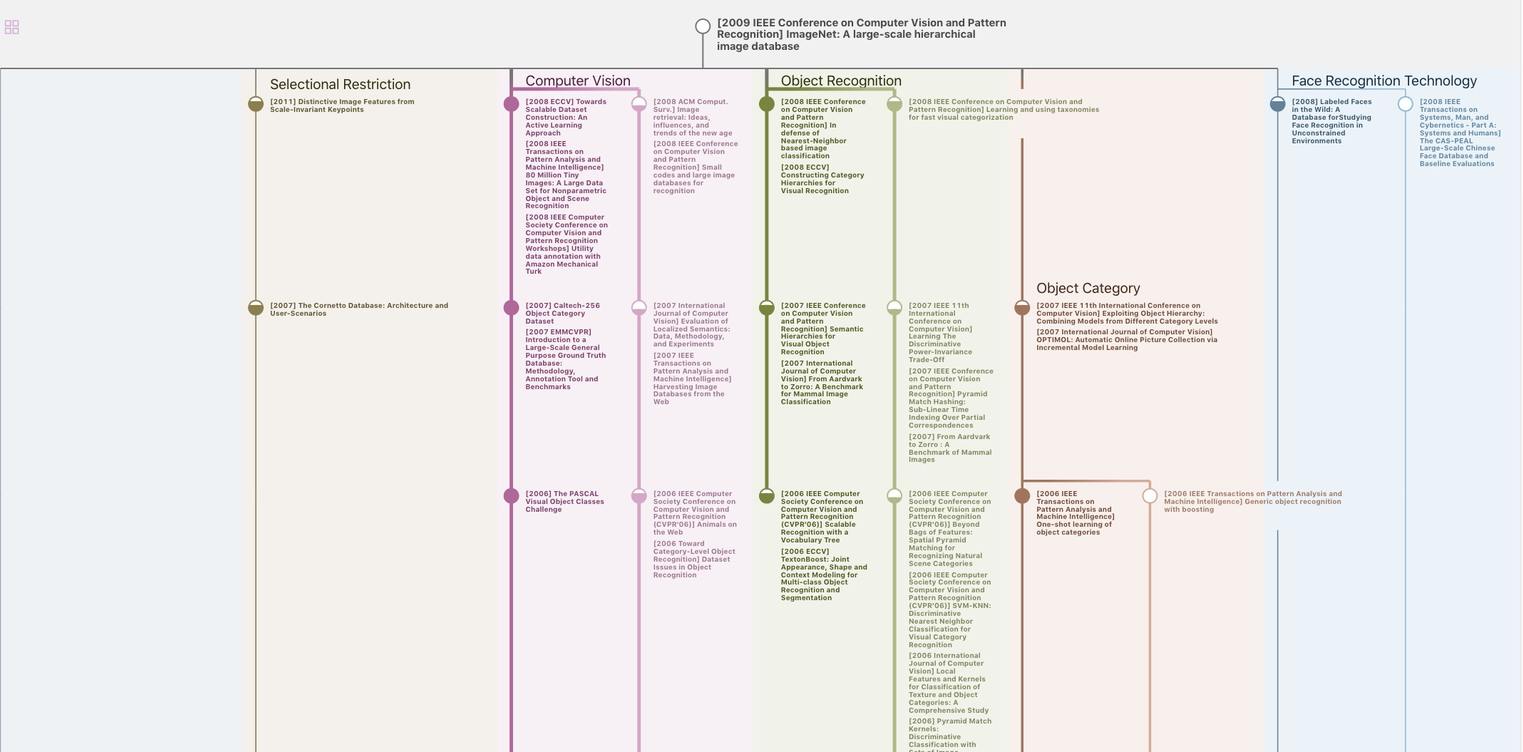
生成溯源树,研究论文发展脉络
Chat Paper
正在生成论文摘要