An automatic and efficient pulmonary nodule detection system based on multi-model ensemble
biorxiv(2020)
摘要
Accurate pulmonary nodule detection plays an important role in early screening of lung cancer. Although there are many presented CAD systems based on deep learning for pulmonary nodule detection, these methods still have some problems in clinical use. The improvement of false negatives rate of tiny nodules, the reduction of false alarms and the optimization of time consumption are some of them that need to be solved as soon as possible. In view of the above problems, in this paper, we first propose a novel full convolution segmentation framework for lung cavity extraction in preprocessing stage to solve the time consumption problem of the existing pulmonary nodule detection systems. Furthermore, a 2D-NestedUNet segmentation network and a 3D-RPN detection network is stacked to get the high recall and low false positive rate on nodule candidate extraction, especially the recall of tiny nodules. Finally, a false positive reduction method based on multi-model ensemble is proposed for the further classification of nodule candidates. Our methods are evaluated on several public datasets, LUNA16, LNDb and ChestCT2019, which demonstrated the superior performance of our CAD system.
更多查看译文
关键词
detection,multi-model
AI 理解论文
溯源树
样例
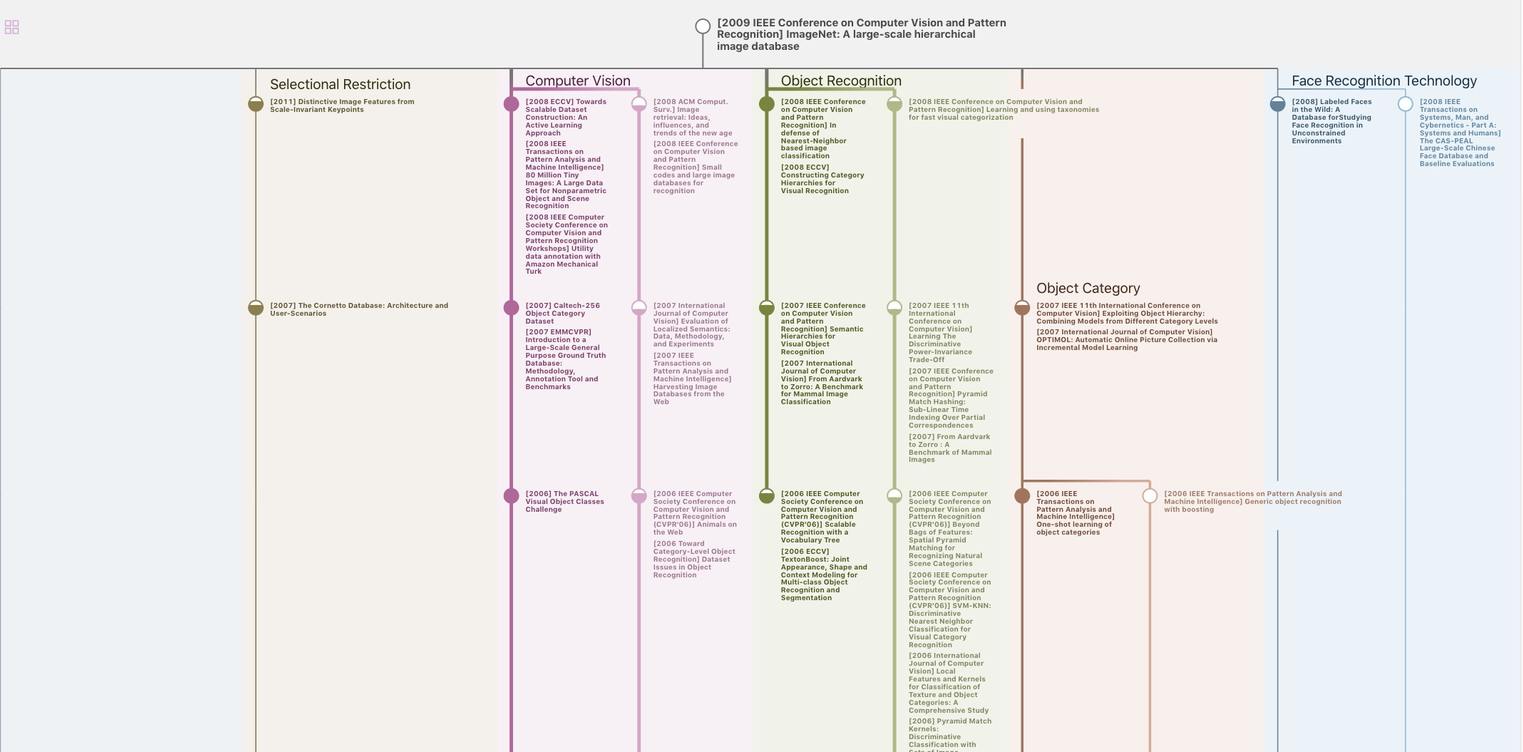
生成溯源树,研究论文发展脉络
Chat Paper
正在生成论文摘要